Prime Surprisal as a Tool for Assessing Error-Based Learning Theories: A Systematic Review
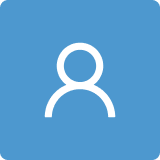
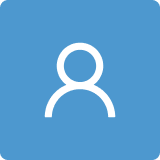
Round 1
Reviewer 1 Report
Comments and Suggestions for AuthorsThis is a thorough and nuanced discussion of the literature on the prime surprisal effect in syntactic priming. The prime surprisal effect refers to the finding that more syntactic priming is observed when the primed structure is relatively unexpected (given the verb). The authors point out that this should only matter from an error-driven learning perspective if the structure is perceived or identified after the verb. And indeed the effect seems to be larger with English Datives than with passives, where the structure is identifiable before the verb is recognized. (Though the passive is always surprising and so might be primed more overall). The paper is systematic in study selection, and both nuanced and careful in its discussion about the conclusions that can be drawn given the small number of studies involved. I only have minor suggestions for improvement.
My main question is why prime surprisal is defined as probability of structure *conditional on the verb*. An inverse frequency / unconditioned probability effect of the primed structure is also consistent with error-driven learning and not necessarily different in mechanism. So the fact that there is more priming of passives than of actives appears to be consistent with the theory as well, and attributable to the same cause of surprisal.
It is also worth pointing out that Jaeger & Snider (and, earlier, Snider's dissertation) were based on finding such inverse prime frequency effects in other types of priming. This includes Moder (1992) for morphological priming -- frequent verbs priming morphemes they contain less than rare verbs do -- Thomsen et al. (1996) for semantic priming, and Goldinger et al. (1989) and Luce et al. (2000) for inhibitory phonological priming (see Kapatsinski, 2006, 2007, for a review). Some studies have also found that there was less priming between the same prime and target when the prime was frequent compared to when the target was frequent, which also suggests that prime surprisal matters (see https://bpb-us-e1.wpmucdn.com/blogs.uoregon.edu/dist/a/6941/files/2022/10/Kapatsinski2007HDLS.pdf). These earlier studies had different explanations for prime surprisal effects on priming that did not rely on error-driven learning. So even if such an effect is found, one would still need to show that EDL is the most likely explanation (though I think that is not a difficult argument to make).
I do not follow the reasoning on p.10: "another important prediction of EBL theories: that the PS effect is larger in younger age groups, as their linguistic representations are less stable " – EDL would certainly predict more priming overall in younger learners, but I am not sure it would predict a larger surprisal effect: younger learners may not have yet learned the relevant probabilities of structures given verbs. If so, there would not be any difference between high- and low-surprisal structures.
Relatedly, one might expect structure frequencies to influence processing earlier in development than verb-specific probabilities. (Especially, perhaps, in second language learning). Because it takes less data to estimate context-free probabilities, Nick Ellis has argued that learners start out being sensitive to such probabilities before becoming sensitive to context-dependent contingencies (e.g., Murakami & Ellis, 2022).
References:
Goldinger, Stephen D., Paul A. Luce & David B. Pisoni. 1989. Priming lexical neighbors of spoken words: Effects of competition and inhibition. Journal of Memory and Language, 28:501–18.
Kapatsinski, V. 2006. Towards a single-mechanism account of frequency effect. LACUS Forum, 32: 325-335.
Luce, Paul A., Stephen D. Goldinger, Edward T. Auer & Michael S. Vitevitch. 2000. Phonetic priming, neighborhood activation, and parsyn. Perception and Psychophysics 62:615–25.
Moder, Carol L. 1992. Productivity and categorization in morphological classes. Ph.D. diss.: SUNY Buffalo.
Murakami, A., & Ellis, N. C. (2022). Effects of availability, contingency, and formulaicity on the accuracy of English grammatical morphemes in second language writing. Language Learning, 72(4), 899-940.
Thomsen, C. J., H. Lavine & J. Kounios. 1996. Social value and attitude concepts in semantic memory: Relational structure, concept strength and the fan effect. Social Cognition 14:191–225.
Author Response
We would like to thank the Reviewer for their thoughtful comments and suggestions to improve our manuscript. We have addressed each point in the attached document.
Author Response File: Author Response.pdf
Reviewer 2 Report
Comments and Suggestions for Authors
The paper provides a review of research employing ‘prime surprisal’ paradigms to test error-based learning theories of language processing. This is a timely review because the topic is currently of interest to many researchers, but as the authors note, evidence is still scarce and inconsistent. I think the introduction does a very good job by gradually introducing the ‘pieces of the puzzle’ (priming, prediction, error-based learning) leading up to prime surprisal paradigm. The authors break down the sample of papers included in the review along different lines (whether they found significant priming effects, types of analysis, design, and statistical power). This approach produces some insightful observations on various aspects of study design which will no doubt be useful to other researchers interested in conducting this kind of research. The review then offers some useful recommendations for future studies.
I think there are many positive sides to the paper. However, there are also some points which I think should be addressed to improve the paper, mostly to do with clarity and consistency:
Main comments
1. I was surprised to see the Dual-Path model referred to as ‘the syntax-focused error-based learning theory’ (p.2). In my understanding, the model does not postulate prediction of syntactic structure, but simply next-word prediction (that is what the neural network model in Chang et al 2006 was trained to do, I think). So rather than expecting DOD vs PD, I think the prediction error in the example provided would simply be that the parser expects a noun and encounters the preposition ‘to’ instead.
2. I was confused by the choice to group together in the ‘inconclusive’ category both studies with trending but non-significant results (n = 3) and studies with mixed findings, which observed significant effects in a subset of the data (n = 4) (p. 7).
The status of studies which only observed effects in a subset of the data was not clear to me. For instance, Jaeger & Snider (2008) was one of the studies with mixed findings because they found enhanced priming for passives but not for active. Further down when discussing the active-passive alternation, we read that “no active-passive study in the whole dataset reported significant PS (but see Jaeger and Snider, 2008)” (p. 11) but then we read that “all active-passive studies failed to find significant PS”. Did the authors only consider a study to be evidence of surprisal-driven priming if the effect was shown on both structures in the alternation, and if so, why (e.g., does it imply low power)? I think it should be made explicit.
However, if that was indeed the underlying assumption, I think it is at odds with some of the recommendations for future design made later in the paper. On p. 15, the authors suggest including a typical adult group in studies with new populations as a control: “If PS appears in the typical but not the new group, the difference is likely to come from the differences between the participant groups rather than the underlying approach”. But the same logic could be applied to studies such as Jaeger & Snider 2008: if the effect appears for one structure but not the other, then it could mean that the study is sound and it allows us to draw conclusions about differences between active and passive, in which case it should not be classed as inconclusive. On the other hand (and this is something which would also apply to the typical adult-new population design suggested), if we observe an effect in one structure (or population) but not the other, it could also mean that the size of the effect is different for the two structures (or populations), and the study is adequately powered to detect one but not the other.
So in summary, I think it would be useful to clarify what the authors make of results where the effect is observed in one subset of the dataset, and how they differ from the kind of study that is suggested in the discussion (with typical adults as control).
3. Terminology. The manuscript alternates between using the term ‘(prime) surprisal’ to refer to the property of stimuli (e.g., p. 3: “there are several ways in which prime surprisal can be defined and measured (such as binary versus continuous measures of prime surprisal)”) and using it to refer to the observed behavioural effect (increased priming) which is triggered by high-surprisal stimuli (also p. 3: “if prime surprisal is used as a tool […] it is crucial to establish under what circumstances this effect reliably occurs” and further down “if a prime surprisal study is conducted with second language learning adolescents and no surprisal is found…”). Later on, the term ‘surprisingness’ is used to refer to the property of stimuli (pp. 8-9).
I was also confused by the sentence: “it is problematic if PS (or any experimental method) tests prime surprisal (or any cognitive ability) where we cannot yet be relatively confident that the measure can reliably detect the cognitive ability” (p. 15), where (assuming that PS = prime surprisal), the same term is used to refer to both an experimental paradigm and the phenomenon it means to test.
I think the authors should make sure to use separate terminology for the statistical property of the stimuli (which is what I would personally call surprisal), for the enhanced priming effects that are triggered by high surprisal (e.g., some use “inverse frequency priming”) and the experimental paradigm, to avoid confusion.
Minor points
1. There were some small inconsistencies in the numbers reported:
a. The number of records considered is reported as 44 on p. 4 (last line), but it’s 43 in Figure 1.
b. In Figure 1, 40 reports are assessed for eligibility, then 28 are excluded (4 + 13 + 11), which should leave 12 included for review, but the next box in the figure says that 13 are included.
2. There was also an inconsistency with inclusion criteria. According to p. 11 (Modality section), two studies in the sample (Fine and Jaeger, 2013; Fernandes, 2015) investigated PS effects in comprehension. This is at odds with the criteria for inclusion laid out on p. 4 (third paragraph), where it says that the outcome variable should be structural choice in production.
3. I think it would be very useful to have a summary table of the studies included and their main characteristics in the paper itself, in addition to a more in-depth one on the OSF (on a separate note, I could not access the OSF repository linked in the paper). It does not need to include a lot of data, but at least the names of the studies and some of the main variables examined, e.g. whether they found significant effects, types of participants / language / structures.
4. I don’t think “Consistency” is clear as a header for section 3.1 (p. 7)—I initially didn’t understand that it was mapping onto the first point to be covered (how often PS was observed), and I thought it referred to consistency in approaches rather than findings (especially because the preceding paragraph mentions the variety of approaches). So perhaps something like ‘Prevalence of PS effects’, ‘Consistency of findings’ or something along those lines would be clearer.
5. I found the structure of the section on Analysis strategies (p. 8) a bit confusing. The second paragraph (on logistic mixed effects models) seems oddly placed: the first one introduces variation in how surprisingness is measured, so the reader would expect the second paragraph to expand on that point, but that is done in the third one. Perhaps the information on logistic mixed effects models (which is what the studies have in common) could be provided at the beginning of the section, before addressing the issue of how to measure surprisingness (which is where they differ).
Author Response
We would like to thank the Reviewer for their thoughtful comments and suggestions to improve our manuscript. We have addressed each point in the attached document.
Author Response File: Author Response.pdf
Reviewer 3 Report
Comments and Suggestions for AuthorsThe paper's topic is interesting and important. The way in which the analysis is presented is confusing. First of all, the effect of prime-surprisal is connected to error-based learning. Is this different from error-riven learning? There is a great deal of evidence for error-driven learning, both from linguistics and from general cognitive work on learning. This undermines the premise of the paper, namely that it is relevant to assess the merit of prime surprisal for testing error-based learning theories. I would change the framing of the paper a bit, namely a review of PS effects without focus on error-driven learning.
The paper is also a bit disorganised in some ways. For example, section 3.2 begins with a note that suprisal is defined differently in different studies, followed by a discussion of statistical models. Maybe these points are related, but it is made clear how.
Given that I find the conclusion that we should be careful to PS to evaluate EBL difficult to assess.
Comments on the Quality of English Language
A few typos.
Author Response
We would like to thank the Reviewer for their thoughtful comments and suggestions to improve our manuscript. We have addressed each point in the attached document.
Author Response File: Author Response.pdf
Reviewer 4 Report
Comments and Suggestions for AuthorsPeer Review: Prime surprisal as a tool for assessing predictive learning theories: a systematic review.
The manuscript provides a systematic review of studies using prime surprisal to address the consistency of prime surprisal effects and the role it may play in assessing error-based learning theories. The manuscript contributes a necessary, comprehensive, and timely review of the literature and provides a number of tangible suggestions for future research. I have some comments, listed below; however, these comments are predominately related to improving clarity, rather than highlighting any major issues.
Comment 1 (Line 29): It would be useful to clarify why linguistic predictions notoriously difficult to test experimentally.
Comment 2 (Lines 80 and 81): In the examples, it would be useful to have the words “pass” and “give” in italics (or otherwise distinctive) for clarity.
Comment 3 (Line 119): It would be useful to have more information about why it is particularly difficult to compare various measures of prime surprisal. For example, can the review be more specific about why binary vs continuous measures are not easily comparable? Although the method of analysis may be different, if there is little relationship between the outcomes of these measures, does this question the validity of the construct?
Comment 4 (Line 131, and later line 548): It would be useful to clarify what is meant by diversity in each instance.
Comment 5 (Line 148): This section of the manuscript argues that prime surprisal only appears reliably under certain conditions; however, is this the outcome of the systematic review or pre-stating a position prior to the review?
Comment 6 (Line 157): The systematic review states that a narrow definition was chosen to keep sample for systematic review homogenous, but one of the key points that the manuscript has made is that there is a lot of variation. While I agree that isolating a narrow definition of prime surprisal for the purpose of examining variation across other factors in the review is justified, it may be useful to have more information (or perhaps just acknowledgment) of the reasons underlying the decision to think of prime surprisal in a more restrictive way, but not other factors (for example task type, age).
Comment 7 (Figure 1): In the “records screened” section, there is a double asterisk – does this refer to a caption or is it not relevant?
Comment 8 (Line 391): There could be additional information supporting the statement regarding when learning is heightened, and the reasons why it is important to test such populations.
Comment 9 (Line 597): Throughout this paragraph a number of natural languages are discussed, but there is no mention of artificial languages. If the effects are universal are suggested, then it may be relevant to mention artificial grammars here too.
Comment 10 (General comment): The systematic review highlights that there are many inconclusive findings within the field and that improved power may result in a clear picture of the literature. Given that ones of the clear advantages of Bayesian methods of statistical analysis are being able to distinguish between null and inconclusive results, it seems unusual that the use of such methods within this field specifically would not be recommended. Coupled with the benefits of Bayesian stopping rules (which would help with some of the sampling issues) it would be useful to know if there is any reason why Bayesian methods have not been recommended in this instance.
Author Response
We would like to thank the Reviewer for their thoughtful comments and suggestions to improve our manuscript. We have addressed each point in the attached document.
Author Response File: Author Response.pdf
Round 2
Reviewer 2 Report
Comments and Suggestions for AuthorsI would like to thank the authors for sending their revised manuscript; I am satisfied that my points from the original review have all been addressed.
Author Response
We would like to thank the Reviewer for their work on improving our manuscript!
Reviewer 3 Report
Comments and Suggestions for AuthorsTHank you for taking my concerns seriously. The paper is greatly improved and can be published in its present form.
Author Response
We would like to thank the Reviewer for their work on improving our manuscript!