sEMG-Based Continuous Hand Action Prediction by Using Key State Transition and Model Pruning
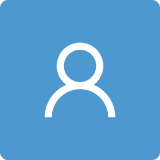
Round 1
Reviewer 1 Report
For the issue that few researchers have investigated continuous hand action prediction based on hand motion continuity, in this paper, authors proposed key state transition as a condition for continuous hand action prediction and simulate the prediction process using a sliding window with long-term memory. Their method achieved relatively high prediction accuracy and low time-consuming for continuous two-hand actions.However, to be a good paper, there are some major modifications have to be made carefully.
1 Since model pruning has been done, is there any experiment comparison before and after pruning?
2 Table 1: a mixture of three and four columns, which is easy to cause misunderstanding without looking carefully.
3 Some sentences in the article need to be carefully pondered and polished. For example, on line 315, page 12: “In this paper, we proposed continuous hand action prediction based on the continuity of hand actions.” The proposal is suitable for a method, not a prediction.
Author Response
Please see the attachment.
Author Response File: Author Response.pdf
Reviewer 2 Report
The manuscript presents a method for hand action prediction using surface electromyography signals.
The paper is well-written, and the methodology and results look good. However, the paper lacks novelty. The methods used GMM-HMM are old and well-known, which should be a baseline approach, not the final one.
The dataset where the method was trained and tested is very small to have any statistical significance on the results. Other works [1, 2] collect larger datasets for the same purpose. Why collect a new one that is no better?
The comparisons with other methods are not enough, the authors compare with LSTM and GRU, which should also be baseline models.
I suggest comparing their method against other datasets and published state-of-the-art methods before considering publication in a Journal.
[1] Kaczmarek P, Mańkowski T, Tomczyński J. putEMG-A Surface Electromyography Hand Gesture Recognition Dataset. Sensors (Basel). 2019 Aug 14;19(16):3548. doi: 10.3390/s19163548. PMID: 31416251; PMCID: PMC6720505.
[2] Ozdemir, Mehmet Akif, et al. "Dataset for multi-channel surface electromyography (sEMG) signals of hand gestures." Data in brief 41 (2022): 107921.
Author Response
Please see the attachment.
Author Response File: Author Response.pdf
Round 2
Reviewer 2 Report
Taking into account the response of the authors. I suggest two changes:
1. Add a contributions paragraph at the end of the introduction stating the innovations that they proposed and that were mentioned in the response to my comments:
Overall, the innovations of the paper are as follows: 1. A new supplement to the research of sEMGbased motion intention recognition; 2. Modified Viterbi algorithm of GMM-HMMs which can build long-term memory for the prediction process; 3. Model pruning which can expand the number of the participating hand gestures for continuous multi-hand action prediction.
2. In the database section, mention the limitations of the existing datasets and how this new dataset fill the gap.
Author Response
Please see the attachment.
Author Response File: Author Response.pdf