PMOT2023: A Large-Scale Multi-Object Tracking (MOT) Dataset with Application to Phytoplankton Observation
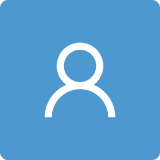
Round 1
Reviewer 1 Report
Positive comments: 1) In this manuscript, the authors have made an attempt to construct a synthetic dataset of 48000 high resolution video frames sequences of phytoplankton that can aid in the detection, classification, counting and estimation of phytoplankton density using automatic identification methods.
2)The dataset can lend itself to various multi object tracking algorithms. .
Shortcomings: The manuscript has certain shortcomings and therefore cannot be published in its current form. However, the study is quite important as regards to the use of high resolution video datasets that can facilitate video-level automatic identification methods for phytoplankton species compared to the traditional and laborious method using a microscope and therefore is worthy of publication in this journal..
Modification required is as follows
1) The manuscript has literature review in almost all sub -headings which distracts the reader from the study carried out by the authors. It is suggested to condense all the literature study in the introduction itself with the last paragraph giving importance of the current study. For. Example, authors can write in the following sequence in the Introduction
After the first paragraph in the introduction , second paragraph to be of machine learning techniques carried out elsewhere, third on sampling and imaging techniques, fourth on multi-object tracking algorithms , fifth para on phytoplankton datasets & evaluation techniques and last para on the work that is carried out. This should be followed by PMOT 2023 dataset construction (no literature survey here ) with sub headings i) phytoplankton sampling (how it has been done for the study area) ii) dataset design and construction ii) video data construction and object trajectory and annotation and finally the PMOT 2023 statistics and analysis with sub-headings as mentioned in the text followed by results and discussion.
Apart from the above modification additional comments are highlighted and marked in the manuscript as sticky notes for improving the text.
Comments for author File: Comments.pdf
Author Response
Dear Reviewer,
Thank you for your thorough review and constructive feedback on my manuscript. I have carefully considered all of your suggestions and have made the necessary revisions to improve the quality of the manuscript.
Please find attached the revised version of the manuscript along with a detailed response to your comments in a separate file. I hope that my revisions have adequately addressed your concerns and improved the clarity and accuracy of the manuscript.
Thank you again for your time and effort in reviewing my manuscript. Your valuable feedback has helped to strengthen the overall quality of the paper.
I modified the content according to your attachment.
At the same time, I also reorganized the structure of the paper according to your instructions.
Sincerely,
Jiaao Yu
Reviewer 2 Report
Dear Authors,
I appreciate your consideration to review the article ID: jmse-2314071, entitled: “PMOT2023: A large-scale multi-object tracking (MOT) dataset with application to phytoplankton observation”, which is under consideration by JMSE journal of MDPI.
The proposed work introduces PMOT2023 dataset of high-resolution video sequences of phytoplankton to facilitate the observation and automatic identification, the proposed dataset contains a duration of 1920 seconds and 48000 frames of images. To consider the paper for publication, authors must be considering the following concerns:
Moderate English language and style revision is required, several tipos have been found.
Author´s name “Qingxuan Lv”, is written correctly?
"PMOT2023 data set" must be included in the keywords.
Every Introduction is composed of four points, justification, related works, description of the proposed work, and description of the organization. I suggest an update of the introduction according to the mentioned.
All the figures that are composed of two or more images must be identified by indices such as a), b), c), etc.
What is the reason to use Sort, deepSort, Bytetrack in the experimental section?
Could you improve the quality of all images?, In Figure 3, I use a zoom 200% to recognize the sentence "Threshold segmentation".
Figure 3. is not described in the text.
I recommend a better description of Figure 3.
Figure 4 don’t correspond to the provided description.
Could you further in deep into the reason of generate four different videos
In section 3.2, change "n frame" by "n-th frame".
If Cx and Cy are constants, could you give us the value?
The paragraph below the Table 1 can be included in the text as part of the table description.
In Figure 7, I consider that the use of discrete parameters (PMOT2023) vs Continuous parameters (SampleSet) is not a fair comparison, I suggest the data homologation.
Paragraph below Table 2 (line 316-319) corresponds to the Table 1 description, I suggest locating the paragraph near Table 1.
Lines 340 and 342 have redundant information that has been provided at the foot of Figure 8.
Every Table, Figure, Equation, .. etc. must be near to its description.
In Table 4, could you include the references in the used Methods; additionally, "Methods" and the title of every column must be written with a capital.
Could you include more details about the process or the way and reason to fix the object detector of Yolo V7?. I don't understand what did you do and why?.
Table 5 is not cited in the text and is not explained.
Table 7. is not provided.
Several important information of in Figure 9 is imperceptible, I used a zoom 600% to observe the provided information into the image.
I appreciate your consideration to integrate the structure of the deep learning method used to evaluate the PMOT2023.
In every technological development is important the integration (Yolo+Bytetrack and Yolo+DeepSort), but in the proposed work the integration of the deep learning model used is not justified, I think that the authors develop the used model empirically, but a justification is required.
I recommend improving the quality of every image, several of them provide information that is imperceptible to the naked eye, I used a zoom in the range of 200% to 600% to observe the provided information.
I suggest the addition of ground-truth image for each Phytoplankton class.
Cold you make emphasis in the confusion matrix or in the parameter FP, FN, TP, and TN, and its relation between Multiple Object Tracking.
How Multiple Object Tracking Accuracy is calculated?
kind regard
Author Response
Dear Reviewer,
Thank you for your thorough review and constructive feedback on my manuscript. I have carefully considered all of your suggestions and have made the necessary revisions to improve the quality of the manuscript.
Please find attached the revised version of the manuscript along with a detailed response to your comments in a separate file. I hope that my revisions have adequately addressed your concerns and improved the clarity and accuracy of the manuscript.
Thank you again for your time and effort in reviewing my manuscript. Your valuable feedback has helped to strengthen the overall quality of the paper.
Sincerely,
Jiaao Yu
Author Response File: Author Response.pdf
Reviewer 3 Report
The paper presents a new data set for MOT
overall. Generating data for researchers requires serious effort. I appreciate the authors for doing this task.
I feel the evaluation toolkit details and evaluation metrics need to be specified, too
if it's the same as MOT data, then work only accumulating labeled video. Which is not very interesting to me.
I would also like to understand how detailed annotations were obtained.
How will this data be helpful in other aquatic studies?
A detailed description of other aquatic challenges needs to be given.
The GitHub link of the data and other evaluation toolkit needs to be given
whether this data is given as a challenge in hackathons for developing the DL algorithm.
a detailed comparison with MOT is needed
Author Response
Dear Reviewer,
Thank you for your thorough review and constructive feedback on my manuscript. I have carefully considered all of your suggestions and have made the necessary revisions to improve the quality of the manuscript.
Please find attached the revised version of the manuscript along with a detailed response to your comments in a separate file. I hope that my revisions have adequately addressed your concerns and improved the clarity and accuracy of the manuscript.
Thank you again for your time and effort in reviewing my manuscript. Your valuable feedback has helped to strengthen the overall quality of the paper.
Sincerely,
Jiaao Yu
Author Response File: Author Response.pdf
Reviewer 4 Report
The point of departure for this manuscript is related to one of the desiderata in the domain of automatic phytoplankton observation, i.e., the lack of available datasets appropriate for the development of video-level machine learning-based approaches (p. 2, l. 58-59 and p. 6, 226-228). In line with this, it reports on the production of a multi-object tracking dataset with application to phytoplankton observation, containing 48,000 micrographs captured by in situ observation devices and grouped in 21 classes of phytoplankton.
In terms of topic, the manuscript is suitable to the journal. My overall opinion is that the presented work is valuable. I suggest to the authors to consider the possibility to address the following remarks.
Remarks:
1. The authors should explain why they refer to the introduced dataset as large-scale? Is it related to the number of recordings (with respect to other phytoplankton-related datasets), the number of represented phytoplankton categories (with respect to other datasets or the given marine environment), etc.?
2. Fig. 4 illustrates the class distribution in the given dataset. It would be useful if the author provided (e.g. in a table) the exact numbers of samples per class.
3. Please discuss the dataset representativeness and balance:
3.1 (Representativeness) To what extent the dataset includes the full range of variability in a phytoplankton population present in the given marine environment? E.g., the dataset captures 21 classes of phytoplankton. What share of phytoplankton classes present in the given marine environment is captured in the dataset?
3.2 (Balance) Are the phytoplankton classes contained in the dataset sampled proportionally?
(I am aware that any claim of dataset representativeness and balance is to a significant extent an act of faith, however it would be useful if the manuscript contained a discussion on these questions.)
4. Keeping in mind that the dataset contains different phytoplankton classes, scales, etc., the authors should explain how values “a” and “b” in Eq. (1) and (2) were determined?
5. The authors state that their dataset can be applied for deep learning “with only minimal preprocessing” (p. 3, l. 76-77). This statement, which is not fully supported in the manuscript, should be either elaborated or removed.
6. Fig. 1, 3, and 6 are not easily readable and may be enhanced.
7. That statement that the given dataset “will not only drive the future development” (p. 6, l. 233) is not fully supported in manuscript (although it may be true) and should be either “softened” or removed.
8. The authors claim that their work “provides new ideas for phytoplankton observation and automatic identification and lays the foundation for the development of standardized, high-quality datasets for video-level automatic recognition algorithms in phytoplankton observation” (p. 3, l. 80-83). While I agree with the second part of this statement (i.e., related to the development of high-quality dataset), the first part of this statement (related to new ideas for phytoplankton observation and automatic identification) has not been clearly explained in the manuscript. The authors should either elaborate on this, or remove it from the manuscript.
Author Response
Dear Reviewer,
Thank you for your thorough review and constructive feedback on my manuscript. I have carefully considered all of your suggestions and have made the necessary revisions to improve the quality of the manuscript.
Please find attached the revised version of the manuscript along with a detailed response to your comments in a separate file. I hope that my revisions have adequately addressed your concerns and improved the clarity and accuracy of the manuscript.
Thank you again for your time and effort in reviewing my manuscript. Your valuable feedback has helped to strengthen the overall quality of the paper.
Sincerely,
Jiaao Yu
Author Response File: Author Response.pdf
Reviewer 5 Report
This paper provides a new dataset called PMOT2023 for the development of video-level automatic identification algorithms for phytoplankton observations. The dataset is based on 48,000 micrographs captured by in situ observation devices and contains 21 classes of phytoplankton. The paper also discusses how multi-objective tracking algorithms can be used to detect, classify, count, and estimate phytoplankton density and help prevent marine ecological disasters.
The paper is well-written and suitable for publication. However, there are some areas that require revision. My specific comments are as follows:
1- Figures 1 and 4 are not referenced in the text of the paper.
2- Section 2.2 on phytoplankton visual tracking is quite lengthy. It would be beneficial to make it more concise.
3- Please change the reference from ‘As shown in Figure 4’ to ‘As shown in Figure 3’.
4- In line 299, the statement ‘The training set is the data samples used to fit the algorithm model for automatic phytoplankton identification, which is used to debug the parameters in the network’ may not be entirely accurate. A more accurate statement might be ‘During the training process, the algorithm adjusts its parameters based on the patterns and features observed in the training data. This helps the algorithm learn to make accurate predictions or classifications for new, unseen data. The training set is not used for debugging the parameters in the network, but rather for training and optimizing the model’.
5- It would be helpful to provide more detailed information about the deep learning model used as a baseline in the methods section.
6- It would be beneficial to release a baseline code along with the dataset. This would allow researchers to quickly explore the dataset and have a point of comparison for their own work.
Author Response
Dear Reviewer,
Thank you for your thorough review and constructive feedback on my manuscript. I have carefully considered all of your suggestions and have made the necessary revisions to improve the quality of the manuscript.
Please find attached the revised version of the manuscript along with a detailed response to your comments in a separate file. I hope that my revisions have adequately addressed your concerns and improved the clarity and accuracy of the manuscript.
Thank you again for your time and effort in reviewing my manuscript. Your valuable feedback has helped to strengthen the overall quality of the paper.
Sincerely,
Jiaao Yu
Author Response File: Author Response.pdf
Round 2
Reviewer 1 Report
I am happy to note that the authors have considerably improved the logical structure of the manuscript and made it quite interesting to read. However figure 3 can be improved with enlarged images since there is lot of space within the box. Similarly in figure 6, the tracks can be drawn thick and bold.
Author Response
Dear Reviewer,
Thank you for your detailed review of our manuscript. We appreciate your insightful comments and suggestions, which we believe have greatly improved the quality of our work.
I have revised Figures 3 and 6 as you suggested to make them more readable.
Sincerely,
Jiaao Yu
Reviewer 2 Report
Dear Author,
I appreciate your time and effort to attend all my comments, I hope you a lot succeed in your Science contribution. In your final version, you must consider the provided information in section 3.2 can not cite itself (Line 219).
If you print Figure 9, the information of the phytoplankton detected can not be recognized; in fact, I use a zoom 200% to observe it, if this information is not required this Figure is not relevant.
Kind Regards
Author Response
Dear Reviewer,
Thank you for your detailed review of our manuscript. We appreciate your insightful comments and suggestions, which we believe have greatly improved the quality of our work.
As you suggested, I have added cite itself wherever SectionX.X in the article.
Figure 9 We just want to show the reader a sample visualization of the multi-objective tracking method on the PMOT2023 dataset. I don't think the w text information in it is necessary for this section. We rather want to show the reader that we can visualize it during testing.
Once again, we appreciate your feedback and support in improving our manuscript. Please let us know if you have any further concerns or suggestions.
Sincerely,
Jiaao Yu
Reviewer 3 Report
the author have revised the paper and it can accepted after language correction and English improvements
Author Response
Dear Reviewer,
Thank you for your detailed review of our manuscript. We appreciate your insightful comments and suggestions, which we believe have greatly improved the quality of our work.
Following your pointers, I have made some improvements to the language expression.
Once again, we appreciate your feedback and support in improving our manuscript. Please let us know if you have any further concerns or suggestions.
Sincerely,
Reviewer 4 Report
The authors have adequately addressed most of the remark from my previous review report and I believe that the manuscript has been sufficiently improved to warrant publication. (In Response 2, the authors state that they have modified Fig. 5, but I assume that they wanted to refer to Fig. 7 instead.)
I suggest to the authors to consider the possibility to address the following remark:
1. Missing citation: “FlowCytobot [? ]”, cf. p. 2, l. 45.
Author Response
Dear Reviewer,
Thank you for your detailed review of our manuscript. We appreciate your insightful comments and suggestions, which we believe have greatly improved the quality of our work.
" (In Response 2, the authors state that they have modified Fig. 5, but I assume that they wanted to refer to Fig. 7 instead.) "
You are right, I did say it wrong, I wanted to refer to Fig. 7.
“FlowCytobot [? ]”
Thank you for your pointers, there was an error in the quote here, I've fixed it.
Once again, we appreciate your feedback and support in improving our manuscript. Please let us know if you have any further concerns or suggestions.
Sincerely,