Deep Impact: A Study on the Impact of Data Papers and Datasets in the Humanities and Social Sciences
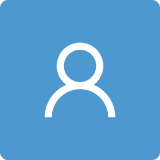
Round 1
Reviewer 1 Report
This study explores the changes in the academic publishing landscape which includes novel publishing venues targeting non-traditional research output such as datasets. It aims to address the value of data papers for research impact and data reuse in the Humanities. Specifically, the impact of data papers in relation to associated research papers and the reuse of associated datasets has been measured by utilizing impact metrics such as citation counts, Altmetrics, views, downloads and tweets.
The findings from this study contribute to the current literature by identifying the most productive area of data publishing in Humanities and Social Sciences; providing the result of analysis on the impact metrics of data papers and associated datasets and research papers; and presenting a positive correlation between the metrics of data papers, associated research papers and datasets.
However, the structure of the paper might be improved by avoiding the separation of too many subsections. For example, Section 1.1.2, Section 1.1.3 and Section 1.1.4 can be integrated into a single subsection. In addition, different research questions addressed in different subsections (e.g. Section 2.2.4, Section 2.3) beyond Section 1.3 may distract the attention of readers. The description on the pyDigest projects in Section 1.1.1 and the description on the two target journal in Section 1.1.3 and 1.1.4 may be abridged while presenting the background of this study and the reasons for selecting the journals, respectively.
Author Response
We would like to thank the reviewer for their helpful and constructive comments.
We have addressed the comments as detailed below.
Section 1.1.2, Section 1.1.3 and Section 1.1.4 can be integrated into a single subsection.
Response: We have integrated sections 1.1.2, 1.1.3, and 1.1.4 into a single subsection (1.1.2).
In addition, different research questions addressed in different subsections (e.g. Section 2.2.4, Section 2.3) beyond Section 1.3 may distract the attention of readers.
Response: We have moved all the research questions (A-E) into section 1.3 and referred to them in sections 2.2.4 and 2.3.
The description on the pyDigest projects in Section 1.1.1 and the description on the two target journal in Section 1.1.3 and 1.1.4 may be abridged while presenting the background of this study and the reasons for selecting the journals, respectively.
Response: We have shortened the text about JOHD in the former section 1.1.3 (now included in section 1.1.2) and motivated the choice of these two journals for our study. We have also reduced the description of the pyDigest project in section 1.1.1 and removed the corresponding figure (which was figure 1).
Reviewer 2 Report
This study provides a general understanding of the impacts of data paper in social science, which is a very important and under-investigated research topic. This study has also clarified the relationship between the data paper and the original articles, materials, and repositories based on real-world evidence. While I still suggest the research questions need to clarify, and IMHO the findings are a little plain and foreseeable. I have the following suggestions and questions:
1. In Introduction, the term of ‘open access’ was used to support the concept of openness. However, general readership might get confused about the wording used here. When seeing open access, we normally come up with the open access movement on journal papers. To diminish the possible misunderstandings, I suggest the author provide a little more justification for noting that open access can also include artifacts such as data, protocols, or other research products.
2. The position of DORA that mentioned in the study should explained. While DORA’s mission is leaning more on the new and fair methods to measure scholarly impact factor (e.g., IF) in different institutions, and does not directly map to the data openness.
3. Another definition question, in Line 74 the article states “Having greater visibility and detailed descriptions of openly available datasets, usually in the form of data papers, has started to be recognized as an important practice within open scholarship.” However, to the best of my knowledge, the archived studies (i.e., openly available data) in a data repository are usually the final products. Seldom of them are published in a data journal. More evidence can better strengthen this claim.
4. Text in Section 1-3 ‘contributions of this study’, does not much talk about the study’s contribution in particular; It looks like clarifying the research questions again.
5. In Line 414, research question 2 was stated here. There’re two questions regarding this RQ2. First, Data reuse could imply many different levels, ranging from citation for background information only to using data in a new research. Specify accurately will be helpful to help readers to anticipate what will come later; Second, it's hard to identify and connect this RQ to the methods and analysis below. From my perspective, besides RQ1, this study also focuses on whether social media, such as twitter hashtags, can have positive impact on the metrics of data papers as well as research papers, which is worth to state as one of the main research questions here.
6. In Line 471-473, after pointing out the questions and insufficiency of using MeSH, it’s expected to answer the reasons why it's still used in this study.
MISC:
1. In Line 22, ‘RDHSS’ was shown but haven’t mentioned before, does it mean RDJ or something else? I suggest the paper specify what this abbreviation came from.
2. In Line 148, Figure 1 should have been Figure 2. Please double check again.
3. In Line 207, five data journals were mentioned here but only showed four of them later.
4. When talks about data (paper) citation, some previous works can provide more background information and help better shape the study:
1) Stuart, D. (2017). Data bibliometrics: Metrics before norms. Online information review, 41(3), 428-435. https://doi.org/10.1108/OIR-01-2017-0008
2) Thelwall, M. (2020). Data in Brief: Can a mega-journal for data be useful? Scientometrics, 124(1), 697-709. https://doi.org/10.1007/s11192-020-03437-1
5. In Line 209, I would recommend to provide the full title of RDJ again here as a reminder, as same goes to JOHD.
6. This study collected metrics from difference sources and tools such as Dimension.ai, Zenodo REST API and the so on. It'll also be helpful if the study provide some imformation for these service.
Author Response
We would like to thank the reviewer for their helpful and constructive comments.
We have addressed each comment as detailed below.
Introduction, the term of ‘open access’ was used to support the concept of openness. However, general readership might get confused about the wording used here. When seeing open access, we normally come up with the open access movement on journal papers. To diminish the possible misunderstandings, I suggest the author provide a little more justification for noting that open access can also include artifacts such as data, protocols, or other research products.
Response: We thank the reviewer for drawing our attention on this very important point. We have added some text at the beginning of the Introduction to clarify the broad scope of Open Research.
The position of DORA that mentioned in the study should explained. While DORA’s mission is leaning more on the new and fair methods to measure scholarly impact factor (e.g., IF) in different institutions, and does not directly map to the data openness.
Response: We thank the reviewer for pointing this out. Indeed, the reference to DORA could seem a bit out of context in the paragraph. In order to clarify the reason why DORA is mentioned without affecting the textflow, we decided to move the reference to footnote 2, explaining the importance of DORA for a fairer evaluation of the research outputs.
Another definition question, in Line 74 the article states “Having greater visibility and detailed descriptions of openly available datasets, usually in the form of data papers, has started to be recognized as an important practice within open scholarship.” However, to the best of my knowledge, the archived studies (i.e., openly available data) in a data repository are usually the final products. Seldom of them are published in a data journal. More evidence can better strengthen this claim.
Response: Thank you for making this point. We have added some references to support this claim.
Text in Section 1-3 ‘contributions of this study’, does not much talk about the study’s contribution in particular; It looks like clarifying the research questions again.
Response: In line with this comment, we have changed the title of section 1.3 to stress that it contains the overview of our research questions.
In Line 414, research question 2 was stated here. There’re two questions regarding this RQ2. First, Data reuse could imply many different levels, ranging from citation for background information only to using data in a new research. Specify accurately will be helpful to help readers to anticipate what will come later; Second, it's hard to identify and connect this RQ to the methods and analysis below. From my perspective, besides RQ1, this study also focuses on whether social media, such as twitter hashtags, can have positive impact on the metrics of data papers as well as research papers, which is worth to state as one of the main research questions here.
Response: We have added a third research question to the list on page 11. Moreover, we have added some text to explain the different levels at which RW2 would be intended, following the reviewer’s point.
In Line 471-473, after pointing out the questions and insufficiency of using MeSH, it’s expected to answer the reasons why it's still used in this study.
Response: We have added an explanation of our choice for using MeSH terms in section 2.1.
MISC:
In Line 22, ‘RDHSS’ was shown but haven’t mentioned before, does it mean RDJ or something else? I suggest the paper specify what this abbreviation came from.
Response: We thank the reviewer for spotting this typo, we have now corrected it to RDJ.
In Line 148, Figure 1 should have been Figure 2. Please double check again.
Response: We thank the reviewer for spotting this and we have corrected the text accordingly.
In Line 207, five data journals were mentioned here but only showed four of them later.
Response: We thank the reviewer for spotting this and we have corrected the text accordingly as the number should be four rather than five.
When talks about data (paper) citation, some previous works can provide more background information and help better shape the study:
1) Stuart, D. (2017). Data bibliometrics: Metrics before norms. Online information review, 41(3), 428-435. https://doi.org/10.1108/OIR-01-2017-0008
2) Thelwall, M. (2020). Data in Brief: Can a mega-journal for data be useful? Scientometrics, 124(1), 697-709. https://doi.org/10.1007/s11192-020-03437-1
Response: We thank the reviewer for making us aware of these papers. We have provided information about them in section 1.2, which has helped to better shape this section.
In Line 209, I would recommend to provide the full title of RDJ again here as a reminder, as same goes to JOHD.
Response: Thank you, we have given the full title of RDJ.
This study collected metrics from difference sources and tools such as Dimension.ai, Zenodo REST API and the so on. It'll also be helpful if the study provide some imformation for these service.
Response: We have added a description of Dimensions in section 2.2.2 and of the Zenodo API in section 2.2.4.
Round 2
Reviewer 2 Report
The current manuscript added more details and make an improvement. I have some more suggestions to add:
The paper stated that citation metrics are not an accurate measure for the data reuse, it may still confuse the reader as to why citation metrics were used in this study. Therefore, a justification on this matter will help clarify the following methodology.
The term of usage statistics in question C was still unclear: could it be understood as the citation and altmetric scores?
Author Response
We would like to thank the reviewer for raising the need for clarification around the two points of citation metrics being used in the paper in spite of them not being optimal and on the phrasing of research question C. We have addressed the first point by specifying how we have tried to tackle the limitations of citation metrics (which are not optimal) by diversifying the range of metrics and objects for which these metrics are taken.
We addressed the second point, following the reviewer's suggestion, by specifying that by replacing the underspecified term "usage statistics" with the more specific terms "citations and altmetrics scores".