Reliable, Fast and Stable Contrast Response Function Estimation
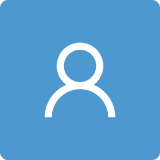
Round 1
Reviewer 1 Report
This research combines computational analysis with physiological recordings to determine the most efficient way to determine the contrast-dependent responses of visual neurons (that is, the method that will give the most accurate estimate for a given amount of recording time).
One point in the introduction I did not fully follow was the link with large datasets - from the ability to record from multiple neurons. The paper seems more concerned with the best way to address the estimation of the Naka-Rushon function for individual neurons, and indeed this is the data plotted. There are other interesting questions here, such as for example that hierarchical analysis methods would be interesting given the expected non-independence of responses of the neurons recorded. As such this aspect of the introduction feels a bit tangential at present.
An interesting conceptual point arises in figure 1. Since these data are drawn from a known model, there is a correct ground-truth, which is unknown for real data. In contrast to this is the criterion we used for deciding that the red curve is the 'correct' fit to the available data, e.g. maximum likelihood of minimum squared error. In my experience non-linear fitting of the Naka-Rushton equation to data of this sort can be very unstable, and strongly dependent on the initial parameters with which the algorithm is seeded. In this example, a quick glance suggests that there would not really be a substantial difference in the size of the residuals of the red and blue fitted curve. I raise this as, in these cases, there is not really a special status for the actual parameter combination that the algorithm lands on (and id expect great uncertainty in these in such cases). So, any particular results is likely to be a local minimum, with potentially many other local minima with very similar qualities of fit. This is important as the logic of the experiment rests on a single defined 'experimental fit' for each data set vs the true underlying model (or true actual curve when better defined with more data). In terms of the methodology here, what would be most relevant is understanding how initial parameters are set, how dependent the fit is on these, and different the quality of fit (or belief in the values of the parameters) vary with the different data samples.
There is a large literature on optimising the sampling of stimulus levels in human psyhchophyics, using for example adaptive sampling methods. It would seem appropriate to link to this, given the amount of theoretical groundwork that has already been done and that seems highly relevant.
One particular point that arises from this is how points are sampled. In the currrent paper, different spacing (linear or logarithmic) different numbers of trials, and different numbers of repetitions are all explored. In human psychophysics, it is generally accepted that different points on the curve may be more informative than others, and require different amounts of samples. For example points on the curve where is starts to accelerate, than again flatten out, are particularly useful. So an additional aspect is not just how many trials, and sample points used, but how the trials are allocated across these points.
Discussion of these points, and in particular the details of the curve fitting procedure, would help to understand the robustness of the approach.
Author Response
We thank the reviewers for their comments and suggestions. All concerns raised have been addressed.
C1. One point in the introduction I did not fully follow was the link with large datasets - from the ability to record from multiple neurons. The paper seems more concerned with the best way to address the estimation of the Naka-Rushon function for individual neurons, and indeed this is the data plotted. There are other interesting questions here, such as for example that hierarchical analysis methods would be interesting given the expected non-independence of responses of the neurons recorded. As such this aspect of the introduction feels a bit tangential at present.
A1. Thank you for the remark. The introduction was rewritten to highlight the underlying problem. Lines 22-29.
C2. An interesting conceptual point arises in figure 1. Since these data are drawn from a known model, there is a correct ground-truth, which is unknown for real data. In contrast to this is the criterion we used for deciding that the red curve is the 'correct' fit to the available data, e.g. maximum likelihood of minimum squared error. In my experience non-linear fitting of the Naka-Rushton equation to data of this sort can be very unstable, and strongly dependent on the initial parameters with which the algorithm is seeded. In this example, a quick glance suggests that there would not really be a substantial difference in the size of the residuals of the red and blue fitted curve. I raise this as, in these cases, there is not really a special status for the actual parameter combination that the algorithm lands on (and id expect great uncertainty in these in such cases). So, any particular results is likely to be a local minimum, with potentially many other local minima with very similar qualities of fit. This is important as the logic of the experiment rests on a single defined 'experimental fit' for each data set vs the true underlying model (or true actual curve when better defined with more data). In terms of the methodology here, what would be most relevant is understanding how initial parameters are set, how dependent the fit is on these, and different the quality of fit (or belief in the values of the parameters) vary with the different data samples.
A2. This point was appointed. In fact, we were concerned about the variation of the nonlinear fitting. All data was iterated with random parameters of the Naka-Rushton equation. We thought they were standard procedures, and for this reason we had not described them. We added an explanation in the new version. Lines 124-127.
C3. There is a large literature on optimising the sampling of stimulus levels in human psyhchophyics, using for example adaptive sampling methods. It would seem appropriate to link to this, given the amount of theoretical groundwork that has already been done and that seems highly relevant.
A3. We pointed out this remark in the first paragraph of the Introduction. Lines 22-29.
C4. One particular point that arises from this is how points are sampled. In the currrent paper, different spacing (linear or logarithmic) different numbers of trials, and different numbers of repetitions are all explored. In human psychophysics, it is generally accepted that different points on the curve may be more informative than others, and require different amounts of samples. For example points on the curve where is starts to accelerate, than again flatten out, are particularly useful. So an additional aspect is not just how many trials, and sample points used, but how the trials are allocated across these points. Discussion of these points, and in particular the details of the curve fitting procedure, would help to understand the robustness of the approach.
A4. We integrated this commentary to another question from the second reviewer. In the results we have added another subsection, Particular cases of CRF, to explain our new results.
Reviewer 2 Report
The paper by Cortes, et al. presents some methodological optimisation work towards more time-efficient estimation of contrast response functions, with particular relevance to multi-electrode array recordings in cat visual cortex. A range of approaches using fixed drifting gratings (in terms of number of contrast levels, relative spacing, and total experiment duration) are considered and applied to a range of simulated and recorded contrast-response functions in a combination experiment with both synthetic and real data.
While a straightforward and useful concept, the work as presented comes across as underdone. Most of the procedures are repeated in triplicate (once in the methods, again in the results, and again in the figure legends) yet key mathematical details are lacking or unclear. The statistics are difficult to follow, despite the fact that none of the statistics are particularly unusual. In the end, I was unable to pull away clear recommendations from the paper, other than that perhaps none of the non-uniform sampling schemes tested significantly outperformed linearly spaced points. The paper should be re-written to focus on the key outcomes the authors are trying to convey.
Since the point of the experiment is to determine, as quickly and as accurately as possible, the true curve describing each cell’s CRF, RMS_#pts can be discarded throughout the manuscript as irrelevant (in comparison to RMS_allpts), and at any rate only a measure of how adequate the fit process (lsqcurvefit) is, rather than a description of how well the experiment allows you to predict future responses.
The presented angle metric depends on the scaling of the variables (e.g. will give different results if the contribution of C50 is scaled in the region [0,1] vs [0,100], holding the other variables equal), it is unclear how the variables are scaled to resolve this issue, if at all.
It is unclear what “logarithmically scaled” means without seeing the objective function written out. Similarly, the notion that the problem is solved when the recording time (in seconds) equals the fit error (in imp/s) is incoherent. A better notion would be to consider the “marginal utility” of obtaining a 90% accurate fit, 99% accurate fit, 99.9% accurate fit, etc. (which is subjective) and maximise the utility per unit time of the experimental procedure, or to look for a notion similar to a ROC curve which considers the sensitivity-specificity trade-off explicitly.
For a monte-carlo experiment, the number of replicates per condition is not clearly stated. I would expect a minimum of 10,000 replicates per experimental condition (not necessarially all with the same NR parameters). Note that with reasonably well written MATLAB code 10,000 replicates of a 16 second, 64 replicate, 20 contrast level experiment including the constrained lsqcurvefit of the NR function takes about 5.5 minutes to execute on my fairly modest desktop.
With the given fit constraints, how well would an extremely linear cell such as the contrast-response for a LGN P cell be fitted? In my hands, I tend to find that 100% is too low an upper bound for c50 for highly linear cells (particularly given that 100% only refers to the maximum contrast achievable by the viewpixx rather than an objective notion of the brightest possible light perceivable).
Related, the “phase-changes” of the NR curve (e.g. saturating hyperbolic vs sigmoid) near n=1 should be discussed if the fit space includes n <= 1. In particular values of n between 0 and 1 permit a fit where c_50 no longer represents the half-maximal response, thereby confusing the interpretation of that parameter, and should probably be excluded unless you’ve got a compelling reason to leave them in.
The distribution of recorded NR parameters (estimated using n_conds = 24) should be visualised with the simulated NR parameters indicated to give an impression of how well the simulated data covers the real data space, and also to how the results may
How well did the different approaches do for estimating curves towards one extreme or the other (e.g. cells with very low c50 or very high n)? The authors make a justification for lumping all of their fits together but I am more interested in
Do all patterns which lack a contrast=0 perform as poorly as 6? If so this would be important to communicate clearly.
In the cat experiment, were neurons recorded in parallel, or was the orientation and spatial frequency of the grating adjusted for each isolated unit?
Author Response
We thank the reviewers for their comments and suggestions. All concerns raised have been addressed.
C1. While a straightforward and useful concept, the work as presented comes across as underdone. Most of the procedures are repeated in triplicate (once in the methods, again in the results, and again in the figure legends) yet key mathematical details are lacking or unclear. The statistics are difficult to follow, despite the fact that none of the statistics are particularly unusual. In the end, I was unable to pull away clear recommendations from the paper, other than that perhaps none of the non-uniform sampling schemes tested significantly outperformed linearly spaced points. The paper should be re-written to focus on the key outcomes the authors are trying to convey.
A1. We rephrased the Section 3.1 Theoretical results to simplify the read. Lines 342-354. Furthermore, statistical data were simplified to be better understood. Many of the statistical reports were added to the figure captions.
C2. Since the point of the experiment is to determine, as quickly and as accurately as possible, the true curve describing each cell’s CRF, RMS_#pts can be discarded throughout the manuscript as irrelevant (in comparison to RMS_allpts), and at any rate only a measure of how adequate the fit process (lsqcurvefit) is, rather than a description of how well the experiment allows you to predict future responses.
A2. In a previous version of this article, reviewers questioned whether a single error was sufficient to solve the problem proposed here. We have overcome this problem by showing more than one type of error. On the one hand, all three types of errors are validated, since they show convergent solutions of experimental conditions (see answer A4). On the other hand, other studies show that using some points of the CRF would be adequate to validate the curve fit. The present work shows that this is not always the case. We have emphasized this argument in the material and methods (Lines 208-209), and discussion (Lines 744-746).
C3. The presented angle metric depends on the scaling of the variables (e.g. will give different results if the contribution of C50 is scaled in the region [0,1] vs [0,100], holding the other variables equal), it is unclear how the variables are scaled to resolve this issue, if at all. It is unclear what “logarithmically scaled” means without seeing the objective function written out.
A3. Points of contrast scales were always considered between [0, 100]%, as Figure 3J shows. We have highlighted this point. Logarithmic scale was further explained in the test. Lines 238-241.
C4. Similarly, the notion that the problem is solved when the recording time (in seconds) equals the fit error (in imp/s) is incoherent. A better notion would be to consider the “marginal utility” of obtaining a 90% accurate fit, 99% accurate fit, 99.9% accurate fit, etc. (which is subjective) and maximise the utility per unit time of the experimental procedure, or to look for a notion similar to a ROC curve which considers the sensitivity-specificity trade-off explicitly.
A4. We knew that the cross point between the error estimator and experimental time could give a subjective analysis. In a previous version of the article, we have used a percentage of the relative error. This procedure gave us similar experimental conditions as best patterns that satisfy our constraints. However, using only one error estimator could change the optimal set of parameters scales we found theoretically and experimentally. We avoid such fluctuations by using three different error estimators. We found that the four final sets of parameters were consistent across the three error estimators, and similar to those experimental conditions used in the previous procedure. This regularity between error estimators indicates that the analysis performed is consistent. We highlighted this observation in the text. Lines 194-197.
C5. For a Monte-Carlo experiment, the number of replicates per condition is not clearly stated. I would expect a minimum of 10,000 replicates per experimental condition (not necessarially all with the same NR parameters). Note that with reasonably well written MATLAB code 10,000 replicates of a 16 second, 64 replicate, 20 contrast level experiment including the constrained lsqcurvefit of the NR function takes about 5.5 minutes to execute on my fairly modest desktop.
A5. Thank you to point out our misleading. We have clarified this in the text. Lines 229-230.
C6. With the given fit constraints, how well would an extremely linear cell such as the contrast-response for a LGN P cell be fitted? In my hands, I tend to find that 100% is too low an upper bound for c50 for highly linear cells (particularly given that 100% only refers to the maximum contrast achievable by the viewpixx rather than an objective notion of the brightest possible light perceivable).
C7. Related, the “phase-changes” of the NR curve (e.g. saturating hyperbolic vs sigmoid) near n=1 should be discussed if the fit space includes n <= 1. In particular values of n between 0 and 1 permit a fit where c_50 no longer represents the half-maximal response, thereby confusing the interpretation of that parameter, and should probably be excluded unless you’ve got a compelling reason to leave them in.
A6 & A7. As the attached figure shows, if C50 is at 100%, CRF are described as saturated, otherwise non-saturated (Panel A). Calculations of the CRF with boundaries higher than 100% contrast for the C50 show a misleading maximum firing rate (Rmax). Rmax tends to reach unreal maximum that are much higher than the real dynamic range (Panel B). If the contrast curve is divided into two areas from its inflection point, a ratio exists between the two areas. An optimal point, alpha, has been calculated to investigate when saturated curves are generated or not. Our preliminary data suggest that neurons presenting a saturated profile would need more energy (more luminescence levels) to reach an asymptotic Rmax. Saturation is a common process in neurons. However, many neurons in the visual cortex expressing such responses may indicate wrong luminescence settings. In our case, few neurons have n close to 1. This work is currently in progress. We have clarified in the text why we do not use C50 greater than 100%. Lines 125-127. We have also studied the profile of such neurons in a new set of analysis.
C8. The distribution of recorded NR parameters (estimated using n_conds = 24) should be visualised with the simulated NR parameters indicated to give an impression of how well the simulated data covers the real data space, and also to how the results may
C9. How well did the different approaches do for estimating curves towards one extreme or the other (e.g. cells with very low c50 or very high n)? The authors make a justification for lumping all of their fits together but I am more interested in.
Simulations and data analysis were performed to answer both questions. These results were added to the article. Lines 549-552; lines 604-631. Figure 5F and Figure 8.
C10. Do all patterns which lack a contrast=0 perform as poorly as 6? If so this would be important to communicate clearly.
We rephrase and add more information for the last paragraph of the article. Lines 756-760.
C11. In the cat experiment, were neurons recorded in parallel, or was the orientation and spatial frequency of the grating adjusted for each isolated unit?
A11. Extracellular recordings were through all layers of the visual cortex. Recordings between areas 17 and 21a, and 18 and posteromedial lateral suprasylvian area (PMLS) were at the same time. Spatial and temporal frequencies were used that were within the stimulation range of areas 17 and 21a (SF = 0.3 c/deg and TF = 3 Hz), and of area 18 and PMLS (Movshon et al. 1978; Zhang et al. 2017, Tardif et al. 1996; Morley and Vickery 1997; Villeneuve et al. 2009). We add this information in the manuscript. Lines 293-300.
Author Response File: Author Response.docx
Round 2
Reviewer 2 Report
The paper is much improved from the previous version, and most of my comments have been addressed to my satisfaction. Comments C8 and C3 were not completely understood but it is not critical that those points be further rectified.
My thanks to the authors for undertaking the additional simulations and analyses requested.
Author Response
Dear Reviewer,
We would like to thank you for your comments that enhanced the quality of the article during this review process.
Sincerely yours,
Nelson Cortes