Reinforcement Learning-Based Formation Pinning and Shape Transformation for Swarms
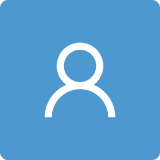
Round 1
Reviewer 1 Report
Comments and Suggestions for AuthorsIn this paper, authors study the field of formation pulling and shape transformation of Boids, with a focus on drone swarms. To address this limitation, they introduce reinforcement learning (RL) into the framework of Boids to enhance their decision-making process and local interactions. In order to achieve continuous obstacle avoidance and maximize spatial coverage in the simulation scenario, they proposed a method based on the Q-learning network to improve the cohesion and repulsion parameters in the Boids model, causing expansion and contraction of the formation and making the behavior of drones in response to changing environmental conditions. Additionally, they introduce a virtual leader to maintain agent position and coordination stability, mirroring the leadership and coordination seen in drone swarms. This method introduces a virtual leader to achieve agent position and coordination stability between agents. These strategies enable drone swarms to make informed decisions about their movement patterns, thereby promoting efficient, synchronized collective movement. Through a series of designed experiments and simulations, they demonstrate the results of reinforcement learning-based intelligent expansion and contraction.
The topic of the paper is interesting, however, the quality of the paper should be improved. Detailed comments are given as:
1. The motivation is not clear. Please explain the importance of the proposed solution. What are the main contributions of the work?
2. Authors have used several notations, I recommend to add a table that contains all the notations. Also check the whole manuscript for typos and grammar errors.
3. In section 2 and 3, it is recommended to provide suitable references for equations and definitions?
4. The proposed algorithm/method section should be clearly identified. Authors should discuss Algorithm 1 in the main text step by step.
5. Few figures are too small, enlarge them, high-quality figures are suggested.
6. The simulation results are weak, it is suggested to provide comparison of the proposed approach with recently published work. In addition, please discuss the computation complexity of the proposed approach. Moreover, which simulation platform is adopted?
Comments on the Quality of English LanguageQuality of English language should be improved
Author Response
Please see the attachment.
Author Response File: Author Response.pdf
Reviewer 2 Report
Comments and Suggestions for AuthorsAccept with Minor English Corrections.
Comments on the Quality of English LanguageGrammatical Check is required.
Author Response
Please see the attachment.
Author Response File: Author Response.pdf
Reviewer 3 Report
Comments and Suggestions for AuthorsThank you for your submission. Here are my comments:
1) please format references in text correctly (spaces, etc.)
2) section 3 could use a simple example to go step-by-step
3) there is a lot of value in your real-world implementation and example, but I am missing evaluation data in terms of efficiency and comparisons between your solution and baselines
Comments on the Quality of English LanguageThe language should be improved.
Author Response
Please see the attachment.
Author Response File: Author Response.pdf
Reviewer 4 Report
Comments and Suggestions for Authors- Could you provide more details on the experimental setup and methodology used in the real-world experiments?
- Could you discuss the limitations of the proposed approach?
- Could you compare your approach with other existing approaches in the literature, such as the following papers, and highlight the advantages and disadvantages of your approach?
"3D aerial vehicle base station (UAV-BS) position planning based on deep Q-learning for capacity enhancement of users with different QoS requirements", Proc. 15th Int. Wireless Commun. Mobile Comput. Conf. (IWCMC), 2019.
"HAPS-UAV-Enabled Heterogeneous Networks: A Deep Reinforcement Learning Approach," in IEEE Open Journal of the Communications Society, 2023.
Comments on the Quality of English Language
Please improve the readability of the paper.
Author Response
Please see the attachment.
Author Response File: Author Response.pdf
Round 2
Reviewer 3 Report
Comments and Suggestions for AuthorsThank you for your revisions.