Wildfire Identification Based on an Improved Two-Channel Convolutional Neural Network
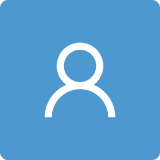
Round 1
Reviewer 1 Report
In general, the article is well presented, rigorously from a scientific point of view, it is interesting and relevant to the journal. But some minor revisions are needed.
The conclusion has to be rewritten. The conclusion has to state the most important outcome of your work. Do not simply summarize the points already made in the body — instead, interpret your findings at a higher level of abstraction.
Comments for author File: Comments.pdf
Author Response
Please see attached file.
Author Response File: Author Response.docx
Reviewer 2 Report
Overall the paper is well written and of interest to the community. A few comments which would improve the quality of the paper:
1. Standard ReLU activation functions have issues with neurons dying over time which effectively reduces the capacity of the network. Why not use one of the fairly common alternatives which avoid this issue (e.g., leaky or parametric ReLU)
2. Line 320 - Authors state the image sizes are unified but do not specify the method. Were the images cropped or stretched?
3. Many of the results presented through the tables have small differences in testing performance. My concern is that the differences may not be statistically significant (i.e., if you were to retrain both models with a different random seed or different test/train split you might not get the same results). For example, the difference in best performance in Tables 2, 3, 6, and 7.
4. There were not many images which were failed to classify in the final testing data (61 total). It would be good to present some of these images in the discussion and identify key features and trends which resulted in the failure.
5. The paper included a lengthy description of many of the methods which are not necessary since the authors did not adapt most of these methods (e.g., pages 4-7 is mostly a summary of methods from other papers). Leaving the discussion in the paper should be fine since it is not plagiarized and may be helpful to readers not familiar with the concepts.
Author Response
Please see attached file.
Author Response File: Author Response.docx