Hotspots and Factors Influencing Vertebrate Roadkill on the Ring Changbai Mountain Scenic Road, China
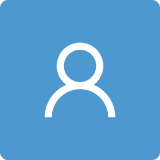
Round 1
Reviewer 1 Report
The manuscript investigates the relation between roadkill and spatial variables. They have a really good survey; 10 years is a very good interval. For the entire manuscript I suggest changing the passive voice, like, the spatial distribution characteristics of roadkill incidence are analyzed in this study, to we analyzed the spatial distribution characteristics of roadkill incidence in this study.
Congratulations to the authors, I hope my comments help you improve the manuscript quality.
Title: I suggest changing the title to:
Hotspots and factors influencing vertebrate roadkill on he Ring Changbai Mountain Scenic Road, China.
1. Introduction
Objectives could be written more clearly.
2. Material and Methods
2.1. Study area
Add more information about the road, is it paved? how many lanes? does it have a shoulder? Traffic volume?
2.2.1. Recording and entry of roadkill locations
I miss some more information about the survey. What speed? What periodicity? How many observers in the car? About surveys on foot, why did you perform them? How you executed it, for example, how many km did you travel on foot, how did you choose these segments? Why did you perform foot surveys? Did you calculate any kind of correction rate for roadkill events?
2.2.4. Examining the factors affecting roadkill in different taxonomic groups
Instead of a Pearson correlation I suggest you perform a GLM with all predictors variables and them use the function dredge from package MuMIn to select the better models. You can use the function model.avg from the same package to get averaged values when more than one model is between those ones with deltaAICc < 2. This way you will understand which variables are more important to explain roadkill density.
About the GLMM, you need to bring more information. What as the response variable? and the random effect? Which kind of distribution did you choose, Poisson? Did you check for overdispersion? How did you choose the best model or models?
Did you check for multicollinearity, for example the variables DTB and DTR may be correlated. I also advise scaling the variables before performing the GLMM, because NDVI is in a different scale.
3. Results
I miss a first section about how many individuals and species you found. Also, about the total effort you performed (total km you traveled).
3.1. Spatial distributions of roadkill
You should explain in material and methods how you assessed if roadkill is random or aggregated. I suggest you use software Siriema that performs a statistical analysis to check it.
Figure 3.
It would be very interesting if you change the color of the segments identified as a roadkill hotspots. Also, add the reserve are in the maps.
3.2.1. Factors affecting roadkill
Here, I advise that instead of performing a Pearson correlation, you use a GLM.
3.2.2. Roadkill density prediction model
NDVI is in a different scale in relation to the other variables. I appeared as an important variable for all groups. I think that after scaling the predictor variables this result may change.
Table 1
You didn’t add the GLMM results for vertebrates.
Conclusions
I think you should point out the segments of the highway that should receive mitigation measures.
Some other more specific comments are in the file attached.
Comments for author File: Comments.pdf
Author Response
The manuscript investigates the relation between roadkill and spatial variables. They have a really good survey; 10 years is a very good interval. For the entire manuscript I suggest changing the passive voice, like, the spatial distribution characteristics of roadkill incidence are analyzed in this study, to we analyzed the spatial distribution characteristics of roadkill incidence in this study.
Reply: yes we have changing most of our manuscript to active voice.
Title: I suggest changing the title to:
Hotspots and factors influencing vertebrate roadkill on the Ring Changbai Mountain Scenic Road, China.
Reply: Thanks to the reviewer for comment on the title of the article. We have revised it according to your good suggestion.
- Introduction
Objectives could be written more clearly.
Reply: thanks for suggestions on introduction, we have modified the research objective, please check the last paragraph in INTRODUCTION.
- Material and Methods
2.1. Study area
Add more information about the road, is it paved? how many lanes? does it have a shoulder? Traffic volume?
Reply: We have added information about roads, highlighted in the text, please check it in 2.1 STUDY AREA.
2.2.1. Recording and entry of roadkill locations
I miss some more information about the survey. What speed? What periodicity? How many observers in the car? About surveys on foot, why did you perform them? How you executed it, for example, how many km did you travel on foot, how did you choose these segments? Why did you perform foot surveys? Did you calculate any kind of correction rate for roadkill events?
Reply: The speed of our survey when driving is 20km/h. From April to October every year, there are two observers in the car, respectively observing the road fatalities on both sides and front of the car.
The reason why we use walking surveys is that the road section surveyed is not long and is relatively close to the town. Therefore, each survey is carried out by two people riding bicycles at a slow pace. The driving speed is close to that of walking, so the accuracy of the survey is higher. After identifying the type of roadkill locations, the carcasses were removed and spray-painted at the location to prevent duplicate counting.
2.2.4. Examining the factors affecting roadkill in different taxonomic groups
Instead of a Pearson correlation I suggest you perform a GLM with all predictors variables and them use the function dredge from package MuMIn to select the better models. You can use the function model.avg from the same package to get averaged values when more than one model is between those ones with deltaAICc < 2. This way you will understand which variables are more important to explain roadkill density.
Reply: We have use the function dredge from package MuMIn to select the better models for each wildlife groups, the results were showed as below. The variables identified through the dredge equation align with the explanatory variables chosen based on the Pearson correlation analysis method.
About the GLMM, you need to bring more information. What as the response variable? and the random effect? Which kind of distribution did you choose, Poisson? Did you check for overdispersion? How did you choose the best model or models?
Reply: Thanks to the reviewer for his suggestions. We used the GLM model and the dredge equation you suggested to construct a road fatality prediction model. The optimal model was selected based on delta<2 and the smallest AICc value. The response variable is the roadkill density of various groups which the roadkill density data is a continuous variable rather than a count variable, and it follows a normal distribution pattern. Therefore, when constructing the generalized linear model, we selected the Gaussian distribution as the Exponential Family.
In 2.2.4, we made the following statement:
"Due to the roadkill density data being a continuous variable rather than a count variable and exhibiting characteristics of a normal distribution, we chose the Gaussian distribution as the Exponential Family when constructing the generalized linear model."
Did you check for multicollinearity, for example the variables DTB and DTR may be correlated. I also advise scaling the variables before performing the GLMM, because NDVI is in a different scale.
Reply: Thanks to the suggestions given by the reviewers, we used a series of models built by GLM and selected the models through the dredge equation. One of DTB or DTR was eliminated from the final equations, eliminating the collinearity problem. The VIF variance inflation factor in Table 2 also proves this point. Therefore, the collinearity problem was considered in the constructed prediction model and related variables were eliminated from the independent variables.
Amphibians:
Call:
stats::glm(formula = RKDAmphibian ~ DTB + DTD + DTP + NDVI + 1, family = gaussian,
data = data)
Residuals:
Min 1Q Median 3Q Max
-10.3628 -1.0462 0.3835 0.6919 5.8695
Coefficients:
Estimate Std. Error t value Pr(>|t|)
(Intercept) 91.280200 1.478356 61.744 < 2e-16 ***
DTB -0.027084 0.001962 -13.802 < 2e-16 ***
DTD 0.009545 0.004746 2.011 0.045957 *
DTP -0.007322 0.002623 -2.791 0.005891 **
NDVI 13.747513 4.089811 3.361 0.000969 ***
---
Signif. codes: 0 '***' 0.001 '**' 0.01 '*' 0.05 '.' 0.1 ' ' 1
Residual standard error: 2.161 on 161 degrees of freedom
Multiple R-squared: 0.8629, Adjusted R-squared: 0.8595
F-statistic: 253.4 on 4 and 161 DF, p-value: < 2.2e-16
Bird:
Call:
stats::glm(formula = RKDBird ~ DTD + DTR + ELEV + 1, family = gaussian, data = high_value_of_roadkill_bird)
Residuals:
Min 1Q Median 3Q Max
-1.0168 -0.5744 -0.3647 0.4183 2.5098
Coefficients:
Estimate Std. Error t value Pr(>|t|)
(Intercept) 1.572e+01 2.206e+00 7.126 3.44e-09 ***
DTD 3.273e-04 1.657e-04 1.975 0.05369 .
DTR 1.961e-04 9.911e-05 1.979 0.05326 .
ELEV -8.214e-03 2.660e-03 -3.088 0.00326 **
---
Signif. codes: 0 '***' 0.001 '**' 0.01 '*' 0.05 '.' 0.1 ' ' 1
Residual standard error: 0.8261 on 51 degrees of freedom
Multiple R-squared: 0.1627, Adjusted R-squared: 0.1134
F-statistic: 3.303 on 3 and 51 DF, p-value: 0.02745
Reptile:
Call:
stats::glm(formula = RKDReptile ~ DTP + DTR + NDVI + 1, family = gaussian, data = highvalue_of_reptile)
Residuals:
Min 1Q Median 3Q Max
-1.8324 -0.6704 0.1490 0.5549 1.6050
Coefficients:
Estimate Std. Error t value Pr(>|t|)
(Intercept) 1.696e+01 1.756e+00 9.659 2.06e-10 ***
DTP 2.155e-03 9.665e-04 2.230 0.0339 *
DTR -1.254e-02 1.684e-03 -7.447 4.12e-08 ***
NDVI -1.054e+01 3.883e+00 -2.714 0.0113 *
---
Signif. codes: 0 '***' 0.001 '**' 0.01 '*' 0.05 '.' 0.1 ' ' 1
Residual standard error: 0.894 on 28 degrees of freedom
Multiple R-squared: 0.7015, Adjusted R-squared: 0.6696
F-statistic: 21.94 on 3 and 28 DF, p-value: 1.638e-07
Mammal:
Call:
stats::glm(formula = RKDMammal ~ DTD + DTR + ELEV + NDVI + 1, family = gaussian, data = high_value_of_roadkill_mammal)
Residuals:
Min 1Q Median 3Q Max
-5.0573 -1.9397 0.5857 2.0341 4.2072
Coefficients:
Estimate Std. Error t value Pr(>|t|)
(Intercept) 1.638e+00 9.191e+00 0.178 0.859118
DTD -1.558e-03 6.695e-04 -2.328 0.022898 *
DTR -2.329e-02 3.486e-03 -6.681 5.31e-09 ***
ELEV 3.929e-02 9.255e-03 4.245 6.77e-05 ***
NDVI -2.804e+01 7.650e+00 -3.666 0.000485 ***
---
Signif. codes: 0 '***' 0.001 '**' 0.01 '*' 0.05 '.' 0.1 ' ' 1
Residual standard error: 2.507 on 68 degrees of freedom
Multiple R-squared: 0.6539, Adjusted R-squared: 0.6335
F-statistic: 32.12 on 4 and 68 DF, p-value: 5.012e-15
- Results
I miss a first section about how many individuals and species you found. Also, about the total effort you performed (total km you traveled).
Reply: thanks for your advices, we have add these information in 3.1.
3.1. Spatial distributions of roadkill
You should explain in material and methods how you assessed if roadkill is random or aggregated. I suggest you use software Siriema that performs a statistical analysis to check it.
Reply: We apologize for the inconvenience, but we were unable to utilize this software for analyzing the spatial distribution of roadkill densities for different animal species. However, we have added a layer in Figure 2 that illustrates the spatial distribution of roadkill densities for various animal species. We kindly request the reviewer to assess the feasibility of this modification.
Figure 3.
It would be very interesting if you change the color of the segments identified as a roadkill hotspots. Also, add the reserve are in the maps.
Reply: We have added the boundary of Changbai Mountain Nature Reserve on the map, and in Figure 3, we have included spatial distribution maps of roadkill densities for different taxonomic groups.
3.2.1. Factors affecting roadkill
Here, I advise that instead of performing a Pearson correlation, you use a GLM.
Reply: This suggestion is excellent, and we have adopted it. We have made modifications in section 2.2.4 of the research methodology, where we have used the GLM method for variable selection.
3.2.2. Roadkill density prediction model
NDVI is in a different scale in relation to the other variables. I appeared as an important variable for all groups. I think that after scaling the predictor variables this result may change.
Reply: Thank you for the reviewer's suggestion. We have taken this into consideration. In our approach of using the GLM method to construct models, we have already standardized the dependent variable before model building. Therefore, in this case, the NDVI data has indeed been standardized.
Table 1
You didn’t add the GLMM results for vertebrates.
Reply: we have add GLMM results for vertebrates in table 2.
Conclusions
I think you should point out the segments of the highway that should receive mitigation measures.
Reply: We have supplemented the section on mitigation measures for reducing roadkill in the “Conclusion”. Specifically, we have added recommendations for the road sections where wildlife crossings should be installed, as well as the pile numbers for bridges and culverts that should undergo habitat creation for these crossings.
Some other more specific comments are in the file attached.
We have edit
Author Response File: Author Response.docx
Reviewer 2 Report
This was a well designed study investigating roadkill rates of vertebrates along a stretch of highway. The data appears well collected, although there are many queries I had around the analysis which is largely unclear, and doesn't fully consider the potential nature of the data. I have provided several points for the authors to consider about this below. In addition, some of the discussion points are speculative or correlative, but could be more explicitly tested by the data available.
My specific comments:
Line 82 – 91 – presumably all roadkill prior to the start of the study was removed to avoid confounding effects of time with the initial survey?
Line 96 – what do you define as “disturbance”? This is a relatively ambiguous, vague term.
Line 98 – 100 – how did you account for minor spatial variability? I.e. the location of a dead animal, may reflect an encounter location some distance prior to its eventual location? Did you consider buffering roadkill locations by a small amount (i..e 50m) and gaining an average of your environmental data over this zone?
Line 120 – does the Generate Near Table tool calculate straight line distances? Or along-the-road distances? Important for roadkill that it’s along the length of the road, rather than an as the crow flies distance.
Line 132-134 – Considerably more information is needed about your statistical analysis. What was the nature of your response variables distribution? What was your random factor? You’ve only listed your fixed factors. How did you validate your models? Did you perform AIC model selection to determine the most parsimonious model? How did you account for spatial-autocorrelation? You also wouldn’t have used ggplot2 to analyse a GLMM. I suspect you probably used lme4. Did you consider whether your relationships were non-linear? This is not uncommon for roadkill data.
Line 138 – I’m struggling to see a Multi-modal Distribution in Figure 2
Figure 3 – unclear if these points are all spatial roadkill, or the points above the 75 percentile in your point density. It would be helpful to show these units as this is what you are analyzing.
Line 160 – 170 – This is not good, and unclear reporting of statistical analysis. Please provide a more ecological interpretation of your results. I.e. Amphibian roadkill decreased further away from rivers. This make the information more accessible for your reader. Equally, please don’t plot every result. Only those that are significant and relevant for the reader. Similarly, your effects sizes of each variable are better reported in-text, rather than on top of each plot.
Line 184 – does that mean the results above are based on all the data? Not just the hotspots? This has not been explained in the methods, or well articulated in the results, please amend.
Line 189 – 190 – just quoting estimates is not sufficient, Presumably these are regression coefficients and so should be cited alongside their upper and lower confidence intervals. This is the case for this entire section where much more appropriate reporting of your results is required.
Line 204 – these are incredibly high R-squared values, how many data points are being considered here? It strikes me that this might be reflective of over-paramaterisation, however, I cannot adjudicate this based on the minimal information you’ve provided. Please more fully explain and report your models throughout, including the sample sizes for each of the analysis, especially these density analysis that are using a subset of the data.
Line 207 – I don’t think its appropriate, nor is there data suggesting roadkill rate is the primary cause of death of wildlife – one would think predation would be…
Line 210 – did you consider any inter-annual trends? Roadkill rates have been shown to change through time: Rendall et al. 2021: Where wildlife and traffic collide: how roadkill rates change through time….
Line 214 – amphibians need to cross the road during hibernation? I think I know what you mean, i.e. there are different critical resources on either side of the road – please explain this more explicitly.
Line 234 – rather than relating your discussion to a particular section of road that is not familiar to the reader, it would be better to relate more to your key results and how these trends might manifest in the landscape. A greater depth of ecological discussion would be good.
Line 242 – you should consider whether or not these trends are non-linear. I would expect impacts to be very localised around these landscape features, and then become almost non-existent thereafter.
Line 251 – interesting, did you consider modelling amphibian roadkill rate as a factor influence reptile roadkill rate to get at this dynamic?
Line 256 – this paragraph required references please.
Line 263 – 271 – some of these results are in contrast to previous studies where higher vegetation density has been found to be a driver of roadkill rates. It would be good to see some discussion around this that more comprehensively reflects the known literature.
Line 273 – 275 – this is false logic. The high incidence of both species does not necessarily mean they are similar. You may have found some of the trends to be similar, in which case these should be directly stated.
Line 284 – you haven’t really created prediction models – this is a subsequent analysis step where you apply your model over a broader spatial area. This would be valuable to do, but needs to be done to make this statement.
Line 290 – you say fences should be installed to prevent amphibians from crossing the road – but earlier in the discussion you state that amphibians need to cross the road to access critical resources – so what negative ramifications might there be for this management suggestion? A more nuanced discussion is required here.
The manuscript was well written, and easy to follow.
Author Response
This was a well designed study investigating roadkill rates of vertebrates along a stretch of highway. The data appears well collected, although there are many queries I had around the analysis which is largely unclear, and doesn't fully consider the potential nature of the data. I have provided several points for the authors to consider about this below. In addition, some of the discussion points are speculative or correlative, but could be more explicitly tested by the data available.
My specific comments:
Line 82 – 91 – presumably all roadkill prior to the start of the study was removed to avoid confounding effects of time with the initial survey?
Reply: Thank you for the reviewer's suggestions! In the initial surveys, we made preliminary assessments of the observed roadkill cases and only recorded fresh carcasses to avoid confounding effects from earlier mortality events. The relevant information has been supplemented in Section 2.2.1.
Line 96 – what do you define as “disturbance”? This is a relatively ambiguous, vague term.
Reply: Thank you for raising the question! In regards to disturbances, we have taken into consideration the impacts of human activities on wildlife in addition to traffic volume. These include factors such as cultivated crops, human settlements, and artificially managed plantations that require human intervention. We have categorized these factors as disturbances in our study conducted on the roads within the protected area.
Line 98 – 100 – how did you account for minor spatial variability? I.e. the location of a dead animal, may reflect an encounter location some distance prior to its eventual location? Did you consider buffering roadkill locations by a small amount (i..e 50m) and gaining an average of your environmental data over this zone?
Reply: Your question is excellent and highlights an aspect that our study may not have thoroughly considered. Based on our field investigations, the majority of roadkill incidents involve small animals, and their locations closely align with our findings. While there are a few instances with slight variations in location, the distance to the actual roadkill spots is very close (typically less than 5 meters). Given this situation, we did not implement a buffering approach for the roadkill locations for environment data. We appreciate your suggestion and will take it into consideration in future research endeavors to further improve the accuracy of our study results.
Line 120 – does the Generate Near Table tool calculate straight line distances? Or along-the-road distances? Important for roadkill that it’s along the length of the road, rather than an as the crow flies distance.
Reply: Thank you very much for your question and suggestion. In our study, we used the "Generate Near Table" tool to calculate the distances between roadkill locations and the nearest bridges, culverts, ponds, rivers, and areas of human disturbance. This tool calculates the straight-line distance between the roadkill points and other locations. We chose to use straight-line distance for the following reasons: Firstly, the design of the road is relatively straight, so the difference between straight-line distance and distance along the road is not significant. This is especially true for the K15-K20 section, which has the highest density of roadkill incidents (Figure 1), and the road is particularly straight in that section. Secondly, the maximum distance between roadkill locations and rivers or lakes is less than 800 meters, and within this distance, the road alignment doesn't vary much, so the difference between straight-line distance and distance along the road is minimal. Lastly, the "Generate Near Table" tool does not take into account the vector data of the road when calculating distances, which is a limitation of the tool. We kindly request the reviewer to evaluate the validity of the above explanation.
Line 132-134 – Considerably more information is needed about your statistical analysis. What was the nature of your response variables distribution? What was your random factor? You’ve only listed your fixed factors. How did you validate your models? Did you perform AIC model selection to determine the most parsimonious model? How did you account for spatial-autocorrelation? You also wouldn’t have used ggplot2 to analyse a GLMM. I suspect you probably used lme4. Did you consider whether your relationships were non-linear? This is not uncommon for roadkill data.
Thank you to the reviewer for the valuable suggestions regarding the research methodology. Your feedback has helped us reevaluate the nature of our data and the methods employed for data processing, enabling us to gain a deeper understanding of the underlying characteristics of our research data. After careful consideration and thorough reanalysis, we would like to provide the following response to your comments:
- After conducting tests, we found that the distribution of roadkill density, which serves as the response variable, follows a normal distribution see figures below.
Tests of Normality |
||||||
|
Kolmogorov-Smirnova |
Shapiro-Wilk |
||||
|
Statistic |
Df |
Sig. |
Statistic |
df |
Sig. |
roadkill density of Amphibian |
.159 |
198 |
.000 |
.916 |
198 |
.000 |
distance to river |
.168 |
198 |
.000 |
.892 |
198 |
.000 |
distance to bridge |
.103 |
198 |
.000 |
.925 |
198 |
.000 |
distance to disturb |
.175 |
198 |
.000 |
.909 |
198 |
.000 |
elevation |
.152 |
198 |
.000 |
.877 |
198 |
.000 |
roadkill density |
.146 |
198 |
.000 |
.934 |
198 |
.000 |
ndvi |
.110 |
198 |
.000 |
.932 |
198 |
.000 |
distance to pool |
.167 |
198 |
.000 |
.878 |
198 |
.000 |
a. Lilliefors Significance Correction |
|
|
|
|
- Random effects are particularly useful for hierarchical or nested data structures where observations are not independent but grouped by some higher-level factor. The data in our study does not exhibit a hierarchical or nested structure, and there are no distinct groups that can be considered as random factors, it is not necessary to include random effects. We focus on building a Generalized Linear Model (GLM) to analyze the main influence factors influencing roadkill density in high-density areas.
- We have perform AIC model selection by using dredge function in MuMIn pack.
- We didn’t account for spatial autocorrelation.
- We have use lme4 package for GLMM.
- Based on the visualizations that demonstrate a linear relationship between the explanatory variables and the response variable, we have included these variables in the analysis.
Line 138 – I’m struggling to see a Multi-modal Distribution in Figure 2
Reply: Thank you for the valuable suggestion. Box plots may not effectively illustrate the spatial distribution of roadkill density. In response to this, we have included an additional layer in Figure 4 to provide a more intuitive visualization of the spatial distribution of roadkill density for different species groups. We kindly request the reviewer to assess and review this addition.
Figure 3 – unclear if these points are all spatial roadkill, or the points above the 75 percentile in your point density. It would be helpful to show these units as this is what you are analyzing.
Reply Thank you for pointing out the unclear of the points in Figure 4(previous Figure 3). In the figure, the points represent locations that are above the 75 percentile in terms of point density. This approach allows us to highlight areas with higher roadkill densities for better visualization and analysis.
Line 160 – 170 – This is not good, and unclear reporting of statistical analysis. Please provide a more ecological interpretation of your results. I.e. Amphibian roadkill decreased further away from rivers. This make the information more accessible for your reader. Equally, please don’t plot every result. Only those that are significant and relevant for the reader. Similarly, your effects sizes of each variable are better reported in-text, rather than on top of each plot.
Thank you for your valuable suggestion. We have deleted 3.2.1, so this is not a question.
Line 184 – does that mean the results above are based on all the data? Not just the hotspots? This has not been explained in the methods, or well articulated in the results, please amend.
The results above are based on hotspots data The previous explanation was somewhat unclear, but we have made revisions. Thank you for bringing it to our attention.
Line 189 – 190 – just quoting estimates is not sufficient, Presumably these are regression coefficients and so should be cited alongside their upper and lower confidence intervals. This is the case for this entire section where much more appropriate reporting of your results is required.
Thank you for your suggestion. We have added the upper and lower confidence intervals of the regression coefficients in Table 2.
Line 204 – these are incredibly high R-squared values, how many data points are being considered here? It strikes me that this might be reflective of over-paramaterisation, however, I cannot adjudicate this based on the minimal information you’ve provided. Please more fully explain and report your models throughout, including the sample sizes for each of the analysis, especially these density analysis that are using a subset of the data.
Thank you very much for the reviewer's suggestion. We have added the information regarding the number of roadkill analysis points within roadkill hotspots, please check the last paragraph of 3.1. For your convenience, here is the additional content that has been included:
Among the 1366 recorded roadkill points for vertebrates 198 of them were located within roadkill hotspots.
Among the 642 recorded roadkill points for amphibians, 166 of them were located within roadkill hotspots.
Among the 131 recorded roadkill points for reptiles, 32 of them were located within roadkill hotspots.
Among the 364 recorded roadkill points for mammals, 73 of them were located within roadkill hotspots.
Line 207 – I don’t think its appropriate, nor is there data suggesting roadkill rate is the primary cause of death of wildlife – one would think predation would be…
Your suggestion is correct. We have added a qualifier as you recommended, stating that roadkill in road environments is the main factor affecting wildlife populations. This modification is appropriate. Please proceed with it. If you have any other questions or need further assistance, please let me know.
Line 210 – did you consider any inter-annual trends? Roadkill rates have been shown to change through time: Rendall et al. 2021: Where wildlife and traffic collide: how roadkill rates change through time….
Thank you for your valuable suggestion. Regarding this issue, our research group has published one paper on this topic, please check this paper: Wang Y, Yang YG, Han YS, et al. Temporal patterns and factors influencing vertebrate roadkill in China. Transportation Research Interdisciplinary Perspectives 15 (2022) 100662
Line 214 – amphibians need to cross the road during hibernation? I think I know what you mean, i.e. there are different critical resources on either side of the road – please explain this more explicitly.
Yes, our statement was indeed unclear. We have revised the sentence according to your suggestion, as follows:
The results showed the slow-moving amphibians often need to cross roads to get different critical resources on either side of the road during various life stages, such as egg-laying, foraging, and land activities..
Line 234 – rather than relating your discussion to a particular section of road that is not familiar to the reader, it would be better to relate more to your key results and how these trends might manifest in the landscape. A greater depth of ecological discussion would be good.
Thank you for your valuable suggestion. To avoid redundancy, our ecological discussion about this phenomenon was in section4.2.
Line 242 – you should consider whether or not these trends are non-linear. I would expect impacts to be very localised around these landscape features, and then become almost non-existent thereafter.
In the roadkill hotspots identified in our study, these influencing factors exhibit a linear relationship with roadkill incidents. This may be attributed to the spatial distribution patterns of wildlife and landscape types on both sides of the road. Our observational data demonstrates this effect. It is important to assess whether the impacts diminish significantly beyond these specific areas. We will explore this aspect further to provide a more comprehensive understanding of the roadkill patterns and their spatial extent in future research. Your suggestion is valuable, and we appreciate your contribution to our research.
Line 251 – interesting, did you consider modelling amphibian roadkill rate as a factor influence reptile roadkill rate to get at this dynamic?
In our current study, we did not specifically model the amphibian roadkill rate as a factor influencing the reptile roadkill rate. However, considering the potential interdependencies and ecological interactions between different taxa, such as amphibians and reptiles, it could be valuable to explore the dynamic relationships between their roadkill rates in future research. By incorporating such factors, we can gain a more comprehensive understanding of the complex dynamics and potential cascading effects within the roadkill phenomenon. Your suggestion is intriguing
Line 256 – this paragraph required references please.
We have deleted this paragraph, so this is not a problem.
Line 263 – 271 – some of these results are in contrast to previous studies where higher vegetation density has been found to be a driver of roadkill rates. It would be good to see some discussion around this that more comprehensively reflects the known literature.
Thank you for the reviewer's suggestions. After careful consideration, we believe that the high roadkill density among wildlife in road segments with low vegetation cover may be attributed to the following factors:
Road segments adjacent to rivers with low vegetation density are often colonized by shrubs and grasses, resulting in improved visibility compared to forested segments[55, 56]. This, in turn, may lead drivers to travel at higher speeds, thereby increasing the risk of road fatalities for small wildlife. Additionally, the unsuitability of shrubs or grasses in these areas as habitat for small rodent species has been established [19]. Small mammals like rodent do not exhibit long-term residency in such areas and tend to either avoid roads or rapidly traverse them when approaching these roadside areas. Furthermore, insightful research has revealed that the increased fatality rates among three rodent species in this region can be attributed to their feeding behavior, particularly their frequent consumption of plant seeds along the roadside [18].
Line 273 – 275 – this is false logic. The high incidence of both species does not necessarily mean they are similar. You may have found some of the trends to be similar, in which case these should be directly stated.
Thank you for the reviewer's suggestions. Based on the feedback, we have made the following revisions to the text:
The relationships between the roadkill density and influencing factors for amphibians and reptiles were found to be similar. The closer to rivers, bridges, culverts, and ponds, the higher the roadkill density. Conversely, the closer the proximity to human activity and the lower the elevation, the lower the overall roadkill density.
Line 284 – you haven’t really created prediction models – this is a subsequent analysis step where you apply your model over a broader spatial area. This would be valuable to do, but needs to be done to make this statement.
You are correct. To accurately evaluate the influence of environmental factors on roadkill across different taxa, conducting comprehensive prediction models and spatial analysis would be valuable. Such analyses would provide more robust and reliable insights into the relation-ship between vegetation cover and the risk of roadkill for different animal groups. Please see 3.2.
Line 290 – you say fences should be installed to prevent amphibians from crossing the road – but earlier in the discussion you state that amphibians need to cross the road to access critical resources – so what negative ramifications might there be for this management suggestion? A more nuanced discussion is required here.
Thank you for your thorough analysis and suggestions. When considering this issue, we recognize that amphibians need to cross roads to access critical resources. However, crossing roads puts them at risk of being killed by vehicles. Therefore, we propose the installation of fences to prevent amphibians from crossing the road. Additionally, these fences can help guide amphibians towards designated passages, such as bridges or culverts. This approach aims to mitigate roadkill incidents while facilitating the safe movement of amphibians. Based on your feedback, we have made the following revisions to our paper. Please check the second paragraph in CONCLUSION.
Author Response File: Author Response.docx
Reviewer 3 Report
1. A taxonomy-specific description of the roadkill mitigation method is needed.
2. Explanation needed for missing roadkill survey period.
3. Roadkill should include a list of species names and populations surveyed.
4. Seasonality is also important in roadkill analysis and requires additional analysis.
The sentences are well-structured and convey the intended meaning effectively. Consider using more precise and specific language to provide more detailed descriptions.
Author Response
- A taxonomy-specific description of the roadkill mitigation method is needed.
Reply: Thank you for the reviewer's suggestions. By further reviewing the literature, we have supplemented the mitigation measures for roadkill incidents in most roadkilled taxa in the "5. Conclusions" section. However, we think it’s better to provide the mitigation method for all animals and focal taxa, it’s unnecessary for specific taxa.
- Explanation needed for missing roadkill survey period.
Reply: Amphibians in the Changbai Mountain region hibernate from early November to March of the following year, during which no surveys are conducted. Roadkill incidents on the Ring Changbai Mountain Scenic Road were recorded annually from April to October between 2009 and 2019.
- Roadkill should include a list of species names and populations surveyed.
Reply: Thank you for the reviewer's reminder. We have made the following additions: We have included the number of species within each roadkill category and the names of species with higher mortality rates. Please refer to Section 3.1 for the detailed information.
- Seasonality is also important in roadkill analysis and requires additional analysis.
Reply: Thank you for your valuable suggestion. Regarding this issue, our research group has published one paper on this topic, please check this paper: Wang Y, Yang YG, Han YS, et al. Temporal patterns and factors influencing vertebrate roadkill in China. Transportation Research Interdisciplinary Perspectives 15 (2022) 100662
Comments on the Quality of English Language
The sentences are well-structured and convey the intended meaning effectively. Consider using more precise and specific language to provide more detailed descriptions.
Author Response File: Author Response.docx