The Industrial Application of Artificial Intelligence-Based Optical Character Recognition in Modern Manufacturing Innovations
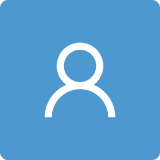
Round 1
Reviewer 1 Report
Comments and Suggestions for AuthorsThis paper proposes The Industrial Application of Artificial Intelligence-based Optical Character Recognition in Modern Manufacturing Innovations, I have the following comments:
(1) English grammar needs further revision.
(2) From the experimental results, does this article only add a preprocessing step?
(3) From the section of Experiment, the experimental results and comparative experiments in the paper are clearly insufficient, and the author needs to supplement them.
(4) The comparative experiment should include the results of the comparative experiment, and the author should add at least 4 newly published references to the comparative experiment
(5) Please add the metrics Accuracy and F1-score in the experiment.
(6) The format of uppercase or lowercase references needs to be unified.
(7) What is the source of experimental data? Is it a public dataset or collected by oneself.
(8) The following relevant references should be discussed by the author in the paper:
[1] Li, L.; Lv, M.; Jia, Z.; Ma, H. Sparse Representation-Based Multi-Focus Image Fusion Method via Local Energy in Shearlet Domain. Sensors 2023, 23, 2888.
[2] Zhang, X.; Li, W. Hyperspectral pathology image classification using dimension-driven multi-path attention residual network. Expert Systems with Applications, 2023, 230, 120615.
Comments on the Quality of English LanguageEnglish grammar needs further revision
Author Response
Please see the attachment.
Author Response File: Author Response.pdf
Reviewer 2 Report
Comments and Suggestions for AuthorsAlthough the results are presented in an appropriate way, there is a number of small comments on the article. In section 3, it is not entirely clear what the specific steps the proposed preprocessing strategy consists of.
Do the authors mean the "preprocessing" include data augmentation?
When comparing models' performances in section 3.2, do the absence of preprocessing mean the considered models haven't been fine-tuned on the prepared augmented training dataset?
I believe that adding some sentences here could noticeably improve the understanding.
Author Response
Please see the attachment.
Author Response File: Author Response.pdf
Reviewer 3 Report
Comments and Suggestions for AuthorsTitle: Review Feedback for "The Industrial Application of Artificial Intelligence-based Optical Character Recognition in Modern Manufacturing Innovations"
Dear Authors,
Thank you for the opportunity to review your paper titled "The Industrial Application of Artificial Intelligence-based Optical Character Recognition in Modern Manufacturing Innovations." Overall, the paper presents a promising advancement in the field of industrial OCR. Below are some feedback points and suggestions for improvement:
1. Introduction and Motivation:
- The introduction should be expanded to provide a clearer motivation for the research. Consider elaborating on the specific challenges faced in modern manufacturing that necessitate advanced OCR solutions, such as the need for automation, efficiency, and accuracy.
- Incorporate citations to relevant papers and studies that highlight the importance of OCR in industrial settings and showcase the potential impact of advanced AI-based approaches.
2. Research Questions (RQ) and Hypotheses:
- It would be beneficial to explicitly state the research questions addressed in the paper and present hypotheses regarding the expected outcomes. This will provide clarity regarding the objectives of the study and guide the readers through the research process. Some links of important paper to include in your motivation sections, please cite them, if you fine them relevant.
1) https://www.igi-global.com/chapter/ai-based-quality-inspection-of-industrial-products/328029
2) https://onlinelibrary.wiley.com/doi/abs/10.1002/isd2.12276
3. Methodology:
- Provide more detailed explanations of the methodologies employed, particularly in the development of the OCR system. Readers would benefit from a deeper understanding of the algorithms, techniques, and models utilized.
- Incorporate statistical testing for precision and recall to assess the performance of the OCR system rigorously. This will enhance the credibility of the reported results and ensure robustness in evaluation.
4. Exploratory Data Analysis (EDA):
- Conduct thorough exploratory data analysis (EDA) with the provided dataset to gain insights into the characteristics of the iron plate text images. Explore factors such as text size, orientation, and background variations to inform preprocessing strategies and model design.
- Present the findings of the EDA in a structured manner, utilizing descriptive statistics and visualizations to illustrate key trends and patterns observed in the data.
5. Annotated Text Image:
- Consider incorporating annotated text images or illustrations in the paper to provide a visual representation of the OCR process to the readers. Organize these visuals in a coherent manner to guide the readers through the various stages of text detection, segmentation, and recognition.
Incorporating these suggestions will enhance the clarity, rigor, and impact of your paper. Overall, the work presents a significant contribution to the field of industrial OCR, and with the suggested improvements, it has the potential to make a valuable addition to the existing body of research.
Best regards,
Author Response
Please see the attachment.
Author Response File: Author Response.pdf
Reviewer 4 Report
Comments and Suggestions for AuthorsThis paper presents and evaluates an Optical Character Recognition (OCR) system based on machine learning for reading text on iron plates. The paper describes the developing process and the data augmentation strategy. This data augmentation process is very necessary for this type of application.
Although the contribution is low, from the research point of view (just finetuning models), I think the application is very interesting and the innovation can be useful.
Comments to improve the paper:
· Increase font in fig 1 and 2
· At the end of the introduction, I’d suggest including a list of contributions.
· Increase size of figure 3 and font in all figures
· In the experiments, did you consider a k-fold strategy? I’d suggest including confidence intervals in the results, to demonstrate that the differences are significant.
· Can you explain more about the language model you used?
· Can you elaborate a bit more the analyses about the main errors of the system?
Author Response
Please see the attachment.
Author Response File: Author Response.pdf
Round 2
Reviewer 4 Report
Comments and Suggestions for AuthorsI thnk the authors have addressed my main comments. Just a minor comment. Regarding the error analysis, I'd suggest more lower analysis: types of errors (numbers, letters, type of fonts,...)
Author Response
Dear Reviewer,
Thank you for your constructive suggestion. Error analysis has been added in Figure 10, and a detailed explanation can be found on Page 14, lines 489 to 504. Please refer to our updated manuscript.
Best regards