3D Octave and 2D Vanilla Mixed Convolutional Neural Network for Hyperspectral Image Classification with Limited Samples
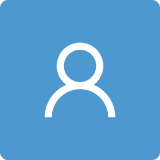
Round 1
Reviewer 1 Report
Please see the attachment.
Comments for author File: Comments.pdf
Author Response
Please see the attachment.
Author Response File: Author Response.docx
Reviewer 2 Report
This paper proposes a refined CNN architecture to deal with the 'very small sample problem' of hyperspectral classification at pixel-level, using a mixed 2D vanilla and 3D octave CNN.
The authors use four public datasets for experiments, showing a better performance than other architectures with same number of labelled samples, in special with lower number of training samples.
The paper is well written and many details are given, but some additional improvements would benefit the clarity of this paper, in my opinion:
- Training sample size is chosen to an absolute pixel number (more or less equally splitter between different categories) in two datasets and a proportion in the remaining datasets (UP and SA). I think that using always relative number of pixels would be more clear (or always absolute, but relative seems more logical for different dataset sizes).
- Figures 4-7 shows classification maps for different algorithms. FC and GT meaning is not defined in the paper or discussion.
- If authors are using open source algorithms for comparison, a reference to used algorithms would be needed for reproducibility.
- A very recent related paper has been very recently published in this journal with three common datasets and similar performance with common 0.5% training sample size figures. For example, it worths to be explained why Salinas dataset OA (Overall accuracy) is different in both papers with same number of samples 0.5% and same algorithm (HybridSN). : https://doi.org/10.3390/rs13122268,
- Selection algorithm for training pixels is not clearly explained. Is it random? If yes, are some training pixels with same class spatially closed? how close? These questions are important for reproducibility also.
Author Response
Please see the attachment.
Author Response File: Author Response.docx