Attention-Unet-Based Near-Real-Time Precipitation Estimation from Fengyun-4A Satellite Imageries
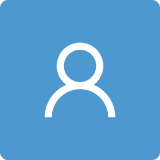
Round 1
Reviewer 1 Report
The current study apply Attention Unet to estimate spatial precipitation from satellite data.
- The content scientifically sounds.
- However, there are some points the authors must improve including its reasoning and comparative deep learning model.
- The detailed review is included in the attachment
Comments for author File: Comments.pdf
Author Response
Please see the attachment
Author Response File: Author Response.pdf
Reviewer 2 Report
(1) A brief summary. The paper is devoted to developing a new algorithm for retrieving precipitation areas and rates from satellite remote data. The approach is purely empirical, based on application of a neural network technique. The novelty of the work is in using a new ANN architecture (previously used in areas of research other than remote sensing) and in improvement of retrievals according to some statistical criteria. These make the results interesting enough to the scientific community.
(2) Areas of strength and weakness. I believe that the issues of strength and weakness of the authors' approach are not nested within the frames of the presented paper and as such they can not be considered as arguments pro or contra publishing the paper. I mean that we deal with a typical ANN approach with its generic strengths and weaknesses. On the one hand it is a generically empirical approach and one can not give solid analytical proof that it is universally applicable and guarantees reliable results. On the other hand, the performed tests, made by the authors, are quite convincing for the considered cases. Such tests are very widespread for the papers of this kind and are usually considered as a satisfactory proof of the method capabilities and advantages over other competing ones. For this reason I don't ask authors any additional questions concerning validation/verification of their approach. These questions should be addressed to the novel "scientific tradition" of using ANNs as a whole. Within this tradition the authors perform quite a solid research.
I have no objection to publishing the article after minor revisions.
(3) Specific comments
As a note, I would like to point out the following:
1) In line 265, instead of "positive" should be "negative"
2) In table 5, instead of "0.74%" should be "-0.74%"
Author Response
Please see the attachment
Author Response File: Author Response.pdf
Reviewer 3 Report
see attached file
Comments for author File: Comments.pdf
Author Response
Please see the attachment.
Author Response File: Author Response.pdf
Round 2
Reviewer 1 Report
The authors improved the manuscript following the provided comment from this reviewer.
The manuscript is good to be published in Remote Sensing.
Reviewer 3 Report
No new comments