Orthogonal Scattering Model-Based Three-Component Decomposition of Polarimetric SAR Data
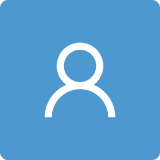
Round 1
Reviewer 1 Report
Dear authors,
Thank you for your solid contributions. I suggest removing references older than the recent 10 years and adding more novel image analysis techniques which are machine learning based ( ex, CNN and ANN ) and conducting a benchmark comparison with the proposed approach and provide that as a summary in the abstract section. I think it will it be in a much better shape and more appealing to the scientists / researchers working in the field.
Author Response
Response to Reviewer 1 Comments
Thank you for your solid contributions. I suggest removing references older than the recent 10 years and adding more novel image analysis techniques which are machine learning based (ex, CNN and ANN) and conducting a benchmark comparison with the proposed approach and provide that as a summary in the abstract section. I think it will it be in a much better shape and more appealing to the scientists/researchers working in the field.
Response: Thanks for the comment and affirmation of our work.
Recently, many novel image analysis techniques based on machine learning have been proposed [1]-[2]. They contribute to the development of image analysis. However, in general, this technology needs to be based on decomposition models or decomposition parameters. For example, the literature [1] successfully uses the X-bragg scattering model as a training model to invert soil moisture. The literature [2] successfully uses the Neumann scattering model as a training model for land-cover classification. This means that only machine approaches are used for image analysis is difficult, since there are no training models. Therefore, the interpretation of the interaction process between ground targets and SAR signals is a prerequisite for image analysis techniques based on machine learning. For this paper, our purpose is to interpret the scattering processes of ground targets.
Therefore, we consider our work to be at least parallel to image analysis techniques based on machine learning. The authors are concerned that readers maybe be misunderstood if extending the scope of the research topic too broadly in the abstract section. In such a case, the authors only add some novel image analysis techniques in the introduction section.
References
- Yin, Q.; Li, J.; Ma, F.; Xiang, D.; Zhang, F. Dual-Channel Convolutional Neural Network for Bare Surface Soil Moisture Inversion Based on Polarimetric Scattering Models. Remote Sens. 2021, 13, 4503.
- Xie, Q.; Wang, J.; Liao, C.; Shang, J.; Lopez-Sanchez, J.M.; Fu, H.; Liu, X. On the Use of Neumann Decomposition for Crop Classification Using Multi-Temporal RADARSAT-2 Polarimetric SAR Data. Remote Sens. 2019, 11, 776.
Author Response File: Author Response.pdf
Reviewer 2 Report
The authors analyzed the necessity of orthogonality between scattering models. After that proposed two mutually orthogonal rank-1 scattering models, which can degenerate to mostly current scattering models.
The mathematical record of the analyzes performed as well as the developed scattering models indicate the originality of the research. The scientific value of the study should be emphasized. However, drawings need to be corrected as they are illegible, especially the marked areas on maps.
Author Response
Response to Reviewer 2 Comments
The authors analyzed the necessity of orthogonality between scattering models. After that proposed two mutually orthogonal rank-1 scattering models, which can degenerate to mostly current scattering models.
The mathematical record of the analyzes performed as well as the developed scattering models indicate the originality of the research. The scientific value of the study should be emphasized. However, drawings need to be corrected as they are illegible, especially the marked areas on maps.
Response: Thanks for the comment. Some drawings have been corrected. Please see the revised paper. Furthermore, the scientific value of the study has been emphasized:
First, the scientific value of the study is emphasized in the abstract section. We make a copy here:
“These two characteristics of orthogonal scattering models are expected to play a positive role in large-scale applications, especially in land-cover classification.”
Second, the scientific value of the study is emphasized in the discussion section. We make a copy here:
“5.2 The Bridging Role of Orthogonal Scattering Models in Target Decompositions
There is a novel reflection symmetry approximation (RSA) algorithm proposed by An et al. [24]. Interestingly, when RSA-processed data is used as the input of the proposed decomposition, from the decomposition results, the proposed decomposition is equivalent to the nonnegative eigenvalue decomposition [36-37], which further validates the proposed decomposition. However, it is noted that these two decomposition methods are essentially different. The nonnegative eigenvalue decompositions emphasize that the scattering contributions of different scattering mechanisms should be nonnegative. The proposed method emphasizes that the scattering models in a decomposition scheme should be as orthogonal as possible. We believe that both decomposition schemes contribute to the interpretation of PolSAR images.
Furthermore, due to the volume scattering process with high polarization entropy being difficult to describe by a model with rank-1, there are only two orthogonal scattering models. However, if a rank-1 scattering model orthogonal to the proposed scattering models is found to describe the "mean" scattering process of volume scattering, in this case, three orthogonal scattering models are used to interpret the PolSAR image. This is equivalent to eigenvalue/eigenvector decomposition. Orthogonal scattering model-based decomposition provides an opportunity to build a bridge between model-based decomposition and eigenvalue-based decomposition.
5.3 The Positive Role in Land-Cover Classification
For large-scale land-cover classification from PolSAR images, a key is to separate different scattering mechanisms robustly and accurately. This means that two requirements lie ahead of the model-based decomposition: 1) the decomposition scheme should allocate the power of varying polarization states reasonably in various scenarios; and 2) the decomposition scheme should be stable. However, to describe the complex scattering processes for different scenes, increasing number of scattering models have been proposed. Even many similar scattering models are introduced into a common decomposition scheme. Unfortunately, this runs counter to the above two requirements for model decomposition. We think a possible way to meet the above two requirements is to establish orthogonal scattering models. Different ground targets are expected to be sensitive to different polarizations, which promotes the development of land-cover classification. For example, surface, double-bounce, and volume scattering processes are clearly distinguished for urban areas. It is expected to contribute to river, building, and forest classification. Furthermore, for agricultural areas, the robust decomposition result of the proposed method indicates robust classification results.”
Finally, the scientific value of the study is emphasized in the conclusion section. We make a copy here:
“The results are consistent with the expectation:
(1) The proposed decomposition is superior in allocating scattering power to different scattering mechanisms.
(2) The proposed decomposition is stable in interpreting PolSAR images.
(3) The proposed method has powerful potential in land-cover classification.
Author Response File: Author Response.pdf
Reviewer 3 Report
In general, the paper is definitely interesting, though there are some questionable statements and assumptions.
Here are some of my remarks.
1) Three-component scattering decomposition proposed by authors seems to be very similar to other well-known decompositions. First of all, there are only two so-called orthogonal models (two components) which are directly linked to surface and double-bounce scattering models. Second, to allow their approach be sensitive to volume scattering, authors added volume scattering component of Freeman decomposition, which is not orthogonal one to previous two – and authors admit the fact by themselves. So, their decomposition suffers from the orthogonality of the models in the same way as other known decompositions.
2) Line 197 – an assumption “two scatterers share the same POA and HA” is incorrect.
3) Authors declare the importance of the level of Pauli scattering vectors decorrelation for their orthogonal models in (15), but seems they do not exploit it. The fact their models 1 and 2 are orthogonal is in fact a property of Pauli decomposition.
4) In eq. (17) for the description of double bounce scattering the authors use expressions applicable for the monostatic scattering, though there is subsequent bistatic scattering from two plates of the dihedral. Well, that is not essential for the essence of the paper in total.
5) In (20) authors estimate angles POA and HA, which are independent characteristics of the scattering surface, but do not exploit it as important results of their decomposition. Why?
6) It is not clear how did they derive expressions 21 (a-d). May be some paper reference(s) will be relevant here.
7) In line 329 authors state that at the simulation stage they add noise to the original coherency matrix. Though the noise level significantly affects the quality of decomposition, authors did not demonstrate the noise influence when discussing the results at all.
Author Response
Response to Reviewer 3 Comments
In general, the paper is definitely interesting, though there are some questionable statements and assumptions.
Here are some my remarks.
(1)Three-component scattering decomposition proposed by authors seems to be very similar to other well-known decompositions. First of all, there are only two so-called orthogonal models (two components), which are directly linked to surface and double-bounce scattering models. Second, to allow their approach be sensitive to volume scattering, authors added volume scattering component of Freeman decomposition, which is not orthogonal one to previous two – and authors admit the fact by themselves. So, their decomposition suffers from the orthogonality of the models in the same way as other known decompositions.
Response: Thanks for the comment and affirmation of our work. We agree with the reviewer’s idea. The proposed decomposition suffers from the orthogonality of the models in the same way as other known decompositions.
However, for 5XD, there are four scattering models similar to the volume scattering model [1]. Furthermore, the combination of surface and cross volume scattering models has basically the same form as the volume scattering model. For 7SD, there are six scattering models similar to the volume scattering model [2]. For example, the combination of surface and helix (or mixed dipole) scattering models can formulate a similar form to the volume scattering model on the diagonal elements. However, for our proposed decomposition, only two proposed scattering models are not orthogonal to the volume scattering model. Furthermore, the estimation of the contributions of orthogonal scattering processes is more related to co-polarizations, but that of the volume scattering process is more related to cross-polarization. This can also mitigate the influence of the non-orthogonal volume scattering model on the decomposition results.
In addition to mitigating the impact of similar scattering models on decomposition results, two mutually orthogonal rank-1 scattering models can degenerate to mostly current scattering models. This contributes to the proposed decomposition being applied to different scenarios.
Second, another purpose of this paper is to convey the necessity of the orthogonality of the scattering models in the decomposition. In the future, with the encouragement of the reviewers, we will further improve our framework for orthogonal decomposition.
References
- Xiang, D.L.; Ban, Y.; Su, Y. Model-based decomposition with cross scattering for polarimetric SAR urban areas. IEEE Geosci. Remote Sens. Lett. 2015, 12, 2496-2500.
- Singh, G.; Malik, R. Seven-Component Scattering Power Decomposition of POLSAR Coherency Matrix. IEEE Trans. Geosci. Remote Sens. 2019, 57, 8371–8382.
(2)Line 197 – an assumption “two scatterers share the same POA and HA” is incorrect.
Response: Thanks for the comment. We agree with the reviewer’s idea. For the real scene, these two scatterers hardly share the same POA and HA.
However, for POA compensation [1]-[2], all the elements of the coherency matrix are rotated by the same POA. HA compensation does the same [3]-[4]. This indicates that all scatterers in a pixel share the same POA and HA.
In fact, such an assumption is forced. When it is assumed that the two scatterers have different POAs and HAs, it will introduce two model parameters, and make the decomposition framework much more complicated. Simple analytical solutions for decomposition methods are difficult to obtain. The above factors hinder the generalization of the decomposition. Therefore, we assume that both scatterers share the same POA and HA.
References
- Lee, J.S.; Ainsworth, T.L.; Wang, Y. Generalized Polarimetric model-based decompositions using incoherent scattering models. IEEE Trans. Geosci. Remote Sens. 2014, 52, 2474–2491.
- Yamaguchi, Y.; Sato, A.; Boerner, W.M.; Sato, R.; Yamada, H. Four-component scattering power decomposition with rotation of coherency matrix. IEEE Trans. Geosci. Remote Sens. 2011, 49, 2251–2258.
- Singh, G.; Yamaguchi, Y.; Park, S.E. General four-component scattering power decomposition with unitary transformation of coherency matrix. IEEE Trans. Geosci. Remote Sens. 2013, 51, 3014–3022.
- An, W.T.; Lin, M.S. A Reflection Symmetry Approximation of Multilook Polarimetric SAR Data and its Application to Freeman–Durden Decomposition. IEEE Trans. Geosci. Remote Sens. 2019, 57, 3649-3660.
(3)Authors declare the importance of the level of Pauli scattering vectors decorrelation for their orthogonal models in (15), but seems they do not exploit it. The fact their models 1 and 2 are orthogonal is in fact a property of Pauli decomposition.
Response: Thanks for the comment. We agree with the reviewer’s idea. Orthogonality is in fact a property of Pauli decomposition. However, this property is indeed utilized, and very important:
- The coherency matrix is derived from the Pauli decomposition. If Pauli scattering components are not orthogonal to each other, they cannot form a basis. In such a case, the coherency matrix cannot be applied for PolSAR image analysis.
- Furthermore, the literature [1] has elaborated on the advantage of Pauli decomposition for their orthogonality. We make a copy here:
“Apart from the physical importance these particular mechanisms have in radar imagery, this decomposition has the further advantage that the scattering mechanisms are orthogonal. This means their separation is possible even in the case of second order statistics, in which noise and depolarization effects can be introduced”
References
- Cloude, S. R.; Fortuny, J.; Lopez-Sanchez, J. M.; Sieber, A. J. Wide-band polarimetric radar inversion studies for vegetation layers. IEEE Trans. Geosci. Remote Sens. 1999, 37, 2430-2441.
(4) In eq. (17) for the description of double bounce scattering the authors use expressions applicable for the monostatic scattering, though there is subsequent bistatic scattering from two plates of the dihedral. Well, that is not essential for the essence of the paper in total.
Response: Thanks for the comment. We apologize for not noting where to use bistatic scattering. The original intention of this paper is to apply monostatic scattering throughout. For eq. (16-17), the authors want to convey to the reader that the two proposed scattering models can also be used to investigate the dielectric properties of ground targets. There is an expression, expecting that readers can easily apply orthogonal models to the inversion of soil moisture.
(5)In (20) authors estimate angles POA and HA, which are independent characteristics of the scattering surface, but do not exploit it as important results of their decomposition. Why?
Response: Thanks for the comment. We agree with the reviewer’s idea. POA and HA are independent characteristics of the surface and double-bounce scattering.
However, POA and HA are estimated by classical methods [1]-[4]. They are not our proposed method, or the focus of this paper. In such a case, POA and HA maps are not shown in this paper.
References
- Lee, J.S.; Ainsworth, T.L.; Wang, Y. Generalized Polarimetric model-based decompositions using incoherent scattering models. IEEE Trans. Geosci. Remote Sens. 2014, 52, 2474–2491.
- Yamaguchi, Y.; Sato, A.; Boerner, W.M.; Sato, R.; Yamada, H. Four-component scattering power decomposition with rotation of coherency matrix. IEEE Trans. Geosci. Remote Sens. 2011, 49, 2251–2258.
- Singh, G.; Yamaguchi, Y.; Park, S.E. General four-component scattering power decomposition with unitary transformation of coherency matrix. IEEE Trans. Geosci. Remote Sens. 2013, 51, 3014–3022.
- An, W.T.; Lin, M.S. A Reflection Symmetry Approximation of Multilook Polarimetric SAR Data and its Application to Freeman–Durden Decomposition. IEEE Trans. Geosci. Remote Sens. 2019, 57, 3649-3660.
(6)It is not clear how they derive expressions 21 (a-d). May be some paper reference(s) will be relevant here.
Response: Thanks for the comment. They are established by the correspondence of the measured matrix eq. (2) and the proposed decompositions eq. (19). In addition, to make the expression of the solution simple and clear, there is a transformation of {δ,ρ} shown in eq. (22).
(7)In line 329 authors state that at the simulation stage they add noise to the original coherency matrix. Though the noise level significantly affects the quality of decomposition, authors did not demonstrate the noise influence when discussing the results at all.
Response: Thanks for the comment. Similar to [1]-[2], noise is introduced to make the simulated data more realistic.
In addition, the influence of noise on the decomposition results has been elaborated. We make a copy here:
“For non-reflection symmetry elements, speckle results from a combination of multiplicative and additive noise components [34]. The polarization SAR echo signals from crop ar-eas generally satisfy the assumption of reflection symmetry, and the correlation between co-polarization and cross-polarization is low, which results in the estimation of non-reflection symmetry power being susceptible to speckle noise. Meanwhile, 7SD has four non-reflection symmetry scattering models to account for polarization. Their unstable estimates all affect the estimation of the volume scattering model. Importantly, they affect the volume scattering power by a factor of four. It is therefore expected that the standard deviation of the volume scattering power is larger, which is consistent with the result shown in Table 8. The unstable decomposition results derived by 5XD and 7SD show a negative impact when similar scattering models are used to describe the scattering process.”
References
- Xie, Q.H.; Zhu, J.J.; Lopez-Sanchez, J.M.; Wang, C.C.; Fu, H.Q. A Modified General Polarimetric Model-Based Decomposition Method With the Simplified Neumann Volume Scattering Model. IEEE Geosci. Remote Sens. Lett., 2018, 15, 1229-1233.
- Lee, J.S.; Pottier, E. Polarimetric Radar Imaging: From Basics to Applications; CRC Press: Boca Raton, FL, USA, 2009.
Author Response File: Author Response.pdf
Reviewer 4 Report
This manuscript proposes orthogonal scattering model-based three-component decomposition. The topic is attractive and the paper is well-written. There are two advantages of the proposed method: (1) It is superior in allocating scattering power to different scattering mechanisms. (2) It is stable in interpreting PolSAR images for different ground targets. I only have some minor comments.
1 The volume scattering model is still not orthogonal for the proposed decomposition. What impacts does it bring to PolSAR image interpretation.
2 The authors pursue the orthogonality between scattering models, will the physical meaning of the model be blurred?
3 The proposed method can only explore three scattering mechanisms. Compared with the seven-component decomposition, whether it will reduce the sensitivity of the decomposition framework to different ground targets.
4 The introduction should be improved. Several key references are missing.
Author Response
Response to Reviewer 4 Comments
This manuscript proposes orthogonal scattering model-based three-component decomposition. The topic is attractive and the paper is well-written. There are two advantages of the proposed method. (1) It is superior in allocating scattering power to different scattering mechanisms. (2) It is stable in interpreting PolSAR images for different ground targets. I only have some minor comments.
(1)The volume scattering model is still not orthogonal for the proposed decomposition. What impacts does it bring to PolSAR image interpretation.
Response: Thanks for the comment. We agree with the reviewer’s idea. There are some impacts due to the nonorthogonal volume scattering model.
The lower the orthogonality between the volume scattering model and the other two scattering models, the more serious the erroneous estimation of volume scattering power will affect the estimation of the powers of other scattering mechanisms: The incorrect estimation of volume scattering power will seriously affect the power estimation of surface scattering and double-bounce scattering. For example, for highly oriented building areas, overestimation of volume scattering power can lead to underestimation of surface and double-bounce scattering powers. However, if Sato's volume scattering model [1] is used instead of the Freeman volume scattering model, the underestimation of the surface scattering power will be reduced because the orthogonality between the volume scattering model and the surface scattering model is higher.
However, it is difficult to derive a volume scattering model orthogonal to the proposed two scattering models. The reason is that the rank of the majority of volume scattering models is three. In addition, the volume scattering process plays a critical role in the interpretation of PolSAR images. Considering the above two factors, the Freeman volume scattering model is integrated into the proposed decomposition. The proposed decomposition separates the different scattering mechanisms as much as possible while considering the volume scattering process.
References
- Sato, Y. Yamaguchi, G. Singh, and S.-E. Park, “Four-component scattering power decomposition with extended volume scattering model,” IEEE Geosci. Remote Sens. Lett., vol. 9, no. 2, pp. 166–170, Mar. 2012.
(2)The authors pursue the orthogonality between scattering models, will the physical meaning of the model be blurred?
Response: Thanks for the comment. As shown in Table 1, there are significant connections between the proposed scattering models and surface and double-bounce scattering models
Table 1. Connections between orthogonal scattering models and surface and double-bounce scattering models.
Parameters value |
Surface |
Double-bounce |
|δ| | (0,1) | (1,+∞) |
|ρ| | (1,+∞) | (0,1) |
Furthermore, the non-reflection symmetry components are regarded as generated by the rotation of orthogonal scattering models [1]. Therefore, the physical meaning of proposed models is clear.
References
- An, W.T.; Lin, M.S. A Reflection Symmetry Approximation of Multilook Polarimetric SAR Data and its Application to Freeman–Durden Decomposition. IEEE Trans. Geosci. Remote Sens. 2019, 57, 3649-3660.
(3)The proposed method can only explore three scattering mechanisms. Compared with seven-component decomposition, whether it will reduce the sensitivity of the decomposition framework to different ground targets.
Response: Thanks for the comment. As shown in [1]-[3], in fact, target decomposition is difficult to distinguish more than three single scatterers since there are only three independent polarization channels.
The seven-component decomposition [4] can improve the sensitivity of scattering models to different ground targets. However, when a large number of non-orthogonal scattering models exist in a decomposition framework, the reliability of the decomposition results will not be guaranteed. Therefore, the purpose of this paper is to extract the three dominant scattering mechanisms stably and reliably. It is expected that the orthogonal decomposition scheme plays a positive role in large-scale image interpretation and PolSAR applications.
References
- Cui, Y. Yamaguchi, H. Yamada, and S.-E. Park, "PolInSAR coherence region modeling and inversion: The best normal matrix approximation solution," IEEE Trans. Geosci. Remote Sens., vol. 53, no. 2, pp. 1048–1060, Feb. 2015.
- Colin, C. Titin-Schnaider, and W. Tabbara, “An interferometric coherence optimization method in radar polarimetry for high-resolution imagery,” IEEE Trans. Geosci. Remote Sens., vol. 44, no. 1, pp. 167–175, Jan. 2006.
- Yamada, Y. Yamaguch, Y. Kim, E. Rodriguez, and W. M. Boerner, “Polarimetric SAR interferometric for forest analysis based on the ESPRIT algorithm,” IEICE Trans. Electron., vol. E84-C, no. 12, pp. 1917–1924, Dec. 2001.
- Singh and R. Malik, "Seven-Component Scattering Power Decomposition of POLSAR Coherency Matrix," IEEE Trans. Geosci. Remote Sens., vol. 57, no. 11, pp. 8371–8382, Nov. 2019.
(4)The introduction should be improved. Several key references are missing.
Response: Thanks for the comment. Several key references have been added in the revised manuscript. Please see the revised manuscript.