EDTRS: A Superpixel Generation Method for SAR Images Segmentation Based on Edge Detection and Texture Region Selection
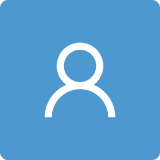
Round 1
Reviewer 1 Report
The writers have made significant efforts, yet there are various uncertainties in this work that must be addressed before release.
The title should be changed.
The writers moved right to superpixel techniques in the introduction section, with no discussion. Previous work has been done to address the speckles issue in SAR data, and certain strategies have been employed to address this issue, since different SAR applications use different techniques. So, in the recent SAR application to coastal studies, there are some novel ways to determine ocean characteristics that are based on quantum image processing, and I recommend that authors update the following references:
1-Li, Y., Shi, H., Jiao, L., & Liu, R. (2012). Quantum evolutionary clustering algorithm based on watershed applied to SAR image segmentation. Neurocomputing, 87, 90-98.
2-Gou, S., Zhuang, X., & Jiao, L. (2009, October). SAR image segmentation using quantum clonal selection clustering. In 2009 2nd Asian-Pacific Conference on Synthetic Aperture Radar (pp. 817-820). IEEE.
3-Marghany, M. (2021). Nonlinear Ocean Dynamics: Synthetic Aperture Radar. Elsevier.
In addition, there a new algorithms have been published by Marghany (2021) for ""Marghany Adaptive Modification Algorithm for lineament automatic detection in Synthetic aperture radar data"" in Marghany, M. (2021). Advanced Algorithms for Mineral and Hydrocarbon Exploration Using Synthetic Aperture Radar. Elsevier.
The authors need to discuss the matter of superpixel methods as segmentation, in fact, these techniques are not able to preserve the edge boundary of the objects.
Authors must discriminate between co-occurrence textures; statistical texture, and texture as features embedded in SAR images.The authors used entropy texture for superpixels.
The novelty of this work does not address probably.
There is no relationship between equations 1 and 2.
Implementing the Guassian filter can lead to lose edge information from SAR image. As it turn the image into blurr features.
Using Kernel window 11 X 11 pixels and lines also causes losing any object’s boundary information in SAR data.
I do not see any logical relationship between equations 1 to 9.
Figure 5 does not indicate entropy results!
In SAR data analysis cannot rely upon image intensity supposes to be normalized radar cross section equation 12 is not correct to be used here also how authors obtained equation 12 from previous equations?
Why authors then jumped to something extremely different; which is using FFT?
Figures 8e and 14f are not accurate as new methsufferssuffering from edge discontinuity.
The discussion is not well addressed.
At this stage, I cannot recommend the paper for publication as long it needs major revision.
Author Response
Dear Reviewer,
We appreciate your help and would like to thank you for your constructive and insightful comments that help to improve the quality of this paper.
We have carefully addressed all your comments and made considerable effort to improve the quality of this paper.
Our responses to your comments and questions are listed as follows.
Kind regards,
Haoran Jiang
- Regarding the title, we have changed the title to: “EGTRS: A Superpixel Generation Method for SAR Images Segmentation Based on Edge Detection and Texture Region Selection”, which reflects more clearly that the purpose of generating superpixels is for segmenting SAR images.
- Regarding the reviewer’s concern on the lack of discussion about the segmentation techniques in the Introduction section, we have cited the following work as suggested by the reviewer:
Li, Y.; Shi, H.; Jiao, L.; Liu, R. Quantum evolutionary clustering algorithm based on watershed applied to SAR image segmentation. Neurocomputing 2012, 87, 90-98.
Marghany, M. Advanced Algorithms for Mineral and Hydrocarbon Exploration Using Synthetic Aperture Radar; Elsevier: 2021.
Marghany, M. Nonlinear Ocean Dynamics: Synthetic Aperture Radar; Elsevier: 2021.
Maged, M. Developing robust model for retrieving sea surface current from RADARSAT-1 SAR satellite data. International Journal of Physical Sciences 2011, 6, 6630-6637.
- Regarding the concern on superpixel methods being not able to preserve the edge boundary of the objects, previous studies [11] [22] have shown that the edges of superpixels can in fact fit well with the edge boundaries of the objects. We have added the following sentences on paragraph 1 of page 2:
As a preprocessing before region merging, superpixel generation is able to over-segment an image, which makes the pixels inside a superpixel to have similar properties. So it preserves image information without destroying the edges of objects.
- Regarding the reviewer’s question about co-occurrence textures, statistical texture, and the texture features embedded in SAR images, we have changed the texture feature extraction method. We use gray level co-occurrence matrix to extract texture, and use principal component analysis to reduce the dimension of multidimensional features.
- Regarding the novelty of this work being not properly addressed, we have updated the contributions on Page 24 in the revised version of this paper.
“1) A new edge detection method is proposed based on 2-D entropy to eliminate the effect of noise. Using virtual points fused with region information, the resultant edges of the proposed method form a band-shaped area, which meets the requirements of generating superpixels in later stages.
2) A region selection method is proposed which combines the periodic judgment and edge constraints to select the regions for texture feature extraction. The selected regions can accurately describe the texture of the target pixel for generating superpixels.
3) A superpixel generating method is proposed which combines edge penalty and texture information. The generated superpixels always keep regular shape and high accuracy.
- Regarding the relationship between Equations 1 and 2, the Equation 1 is used for clustering while the Equation 2 is for merging information. The action of the two equations is not connected.
- Regarding the issue that may arise by using the Gaussian filter, we have rephrased the role of the Gaussian filter on page 5 in the revised version of this paper. Moreover, we have conducted simulation experiments and shown in Figure 4 (b) that the target pixel does not contain neighborhood information and the gradient is not lost.
- Regarding the use of Kernel windows pixels and lines may cause losing any object’s boundary information in SAR data, we have redrawn the action effect and action positions of Kernel windows, which is shown in Figure 3.
- Regarding the logical relationship between Equations 1 and 9, we have deleted Equations 5-9 (in the previous version of this paper), and we have revised paragraph 2-4 in page 6 to make the equations more logical and clear. Equations 2 and 3 are used to build different Gaussian templates for different regions. Equation 4 means that right Gaussian templates are used to convolve in eight directions to generate a virtual pixel in each direction. Equation 5 combines virtual points in different positions for further target detection.
- Regarding “Figure 5 does not indicate entropy results”, we redraw Figure 5. The entropy of the region within the blue box and red box in Figure 5(a) are 8.91 and 8.39, respectively. The entropy of the region within the blue box and red box in Figure 5 (b) are 7.97 and 7.20, respectively.
- Regarding Equation 12, we have changed it accordingly. Although it is not enough to rely solely on pixel intensity in SAR analysis, our work mainly focuses on segmenting SAR images by clustering pixels. Therefore, our priority consideration is pixel intensity.
- Regarding FFT, we have changed the method of texture periodicity judgment by using the autocorrelation function instead of FFT.
- Regarding Figures 8e and 14f, we have optimized and tested the edge detection method. As shown in Figure 11, our method can detect almost all of the edges.
- Regarding the discussion section, we have rediscussed the method in three aspects on Page 24 in the revised version of this paper as below:
The experimental results of several different edge extraction methods on simulated and real SAR images are shown in Figure 8, showing that most of the edges can be extracted efficiently. Due to solid texture and noise, if the threshold is set too low, too many non-edges will be detected, while if the threshold is set too high, the actual edges will not be extracted completely. Before calculating the edge value of each pixel, our method redefines its eight-connected neighborhood to reduce the influence of noise and texture. Moreover, the edge values of the pixels in the high entropy region are weakened to reduce false edges. Our results show that such a treatment is effective.
In Figure 10, the texture feature of the region selected by our method is closer to the actual texture feature value. However, the edges of objects are curved, leading to some deviations in the extracted features. We will keep focusing on this point in further work. CAS uses texture features to generate superpixels. However, its texture descriptor only focuses on the local information of the target pixel and can not accurately reflect texture features. Although the accuracy of the superpixels generated by CAS is occasionally higher than other comparison methods, it is lower than the superpixels generated by our method.
In Figure 11, most of the superpixels can conform to the edges. However, for neighboring regions with similar gray values, the situation becomes worse. In the right half of , almost all superpixel generation methods in the intersection of the two regions produce errors. However, the texture features of the two regions are quite different. We can solve the segmentation error caused by the gray similarity by integrating the texture features into the clustering. Figure 12 shows that the greater the number of superpixels, the more significant the relative increase in accuracy. However, when the number of superpixels increases, the number of pixels in a superpixel decrease, and the advantages of superpixels can not be well reflected. In the blue circle of Figure 15, the superpixels generated by our method are the same in shape and size, but they can still be distinguished when small targets appear. In three real images, the superpixels generated by most methods are challenging to fit the edges well due to the scene's complexity and noise. Although our methods are also affected, they are generally satisfactory.
- For Figures 3-7, Figure 11, and Figure 18, we have revised the figure as suggested.
Reviewer 2 Report
This paper presents a new method for superpixel generation for SAR images which improves the efficiency of processing of such images. It would be good to discuss the differences, if any, between the problelms of superpixel generation and image segmentation, since image segmentation is a well-known problem and it is not clear what the relation between the two related problems might be in the paper.
The proposed method was tested on several SAR images, all but one which were synthetic. I am a bit concerned that the synthetic SAR images are not realistic, and that more testing should be performed on actual SAR images to validate the method.
In the various tables showing the results on the different methods, the results from the proposed methods are always bolded, regardless of whether they perform better than the other tested methods. I believe that instead, only the best-performing method in each case should be written in bold. The proposed method does not always perform better than the other methods.
There were several spelling/typographical errors throughout the paper. Equation (2) appears to be incorrect. I believe the denominator in Equation (2)
2 pi sigma^2 should instead be sqrt(2 pi sigma^2).
Also in Equation (5), the matrix edteX appears to have entries with their signs switched. Finally, there are several spelling errors and the authors are asked to carefully check the paper for proper English grammar and spelling.
Author Response
Dear Reviewer,
We appreciate your help and would like to thank you for your constructive and insightful comments that help to improve the quality of this paper.
We have carefully addressed all your comments and made considerable effort to improve the quality of this paper.
Our responses to your comments and questions are listed as follows.
Kind regards,
Haoran Jiang
- Regarding the differences between superpixel generation and image segementation, we have added the following sentences in the Introduction section:
“SAR image segmentation is a critical preprocessing before image interpretation, scene recognition, and object detection, which has been becoming a research hotspot in remote sensing”,
“Pixel-based SAR image segmentation techniques are sensitive to noise and have high processing complexity. Therefore, the region fusion based on superpixel has been widely used in SAR image segmentation nowadays.”,
“Superpixel generation makes the images under over segmented, and it is a preprocessing before region merging. The pixels inside a superpixel tend to have similar properties that preserve information without destroying the edges”
In addition, we have cited new several references to enhance the description in first paragraph on page 2.
- We have added two actual SAR images from different radar systems to validate the method.
- We have improved our method in edge detection, and the proposed method always performs better than the other methods. We have recorded the data and changed the format of the tables.
- For Equation 2, it is actually correct for the 2D space, as is shown in the following references:
Kostková, J.; Flusser, J.; Lébl, M.; Pedone, M. Handling Gaussian blur without deconvolution. Pattern Recognition 2020, 103, 107264.
- Regarding Equation 5, we have changed our edge detector.
- We have corrected the spelling and grammatical errors throughout the whole paper.
- For Figures 9, 10, 19, and 20, we have revised the figure as suggested.
Reviewer 3 Report
1) What means data distance and spatial distance in (1)? What means data features in page 4, line 165? Represent their definitions in the text.
2) What is the novelty of the edge detector in 3.1.2? How do you design the operators in (5) and (6)?
3) It is suggested that the proposed segmentation method is illustrated with more details in Fig. 2.
4) Please check Eq. (12) and correct it if necessary.
5) There are four adjacent neighbors for the target pixel located in (x-1,y),(x+1,y),(x,y-1), and(x,y+1), which are ignored in Figure 3 and the value of 0 is assigned to them in (4). Why do you not consider these four closest neighbors while they are the most similar pixels to the central pixel?
6) It is suggested that for better understanding the texture region selection and to clear the explanations in lines 284-289 about convergence of AMP, insert a figure for visual comparison among periodic texture and non-periodic texture.
7) Please compare the proposed method with some recently published segmentation methods, which consider texture features and discuss the results.
Author Response
Dear Reviewer,
We appreciate your help and would like to thank you for your constructive and insightful comments that help to improve the quality of this paper.
We have carefully addressed all your comments and made considerable effort to improve the quality of this paper.
Our responses to your comments and questions are listed as follows.
Kind regards,
Haoran Jiang
- Regarding the data distance and spatial distance in Eq. (1), we have added the following description after Equation 1:
“where and represent the intensity proximity and spatiality proximity between the pixel to be clustered and the center pixel, respectively”.
We have also changed the term “data feature” in line 165 (in the previous version of this paper) to “intensity feature” to avoid any possible ambiguity.
- For the reviewer’s concern that the previous edge detector did not consider all directions, we performed local information fusion in eight directions and used the Sobel operator to extract edges in this revised version. With these changes, the quality of edge extraction has been significantly improved, as shown in Figures 11, and 12.
- We have added some details to the flow chart of the proposed method in Figure 2.
- Regarding Equation (12), we changed it to Equations (8) and (9).
- Regarding the issue of 4 pixels being ignored in the edge detection method, we have improved our edge detection method. More specificlly, we performed local information fusion in eight directions and use the Sobel operator to extract edges. After this improvement, the quality of edge extraction has been significantly improved, as shown in Figures 11, and 12.
- We changed the method of texture periodicity judgment by using the autocorrelation function instead of FFT. Therefore, we no longer need to judge whether AMP converges. Moreover, we show the autocorrelation function images of periodic and aperiodic textures in Figure 6.
- For comparing our proposed method with some recently published work which consider texture features, we have updated the description of CAS in the Introduction as below:
“Content-Adaptive Superpixel Segmentation (CAS) is also a superpixel method which extracts texture feature by a robust texture descriptor, and it integrates multiple features and introduces a cluster-based discriminability measure that automatically adjusts feature weights to segment images adaptively”.
We have also conducted experiments with CAS as a comparison method and discussed it in second paragraph on page 24.
- Regarding Figures 2, 3, 6, 7, 11, and 12, we have revised as suggested.
Reviewer 4 Report
In this paper, the Authors propose a new superpixel approach for SAR imagery. The method uses several techniques (edge detection, K-means clustering, texture analysis) to better address this so relevant problem. The methodology is clearly explained and results are compared with similar techniques, outperforming all of them in the results shown. Results are both evaluted: visually and through well-known metrics.
Although the approach is not indeed an outstanding novel one (it is composed by several pattern recognition techniques), it works well, as it resembles useful.
This reviewer has some concerns/suggestions:
- it is not clear what the Authors mean by " 10% speckle noise was added to the images..." This is not the usual way to express that images have been corrupted with speckle. The model used (gamma?, exponential law?), the number of Looks (ENL), shall be clearly stated.
- In the same line, more experiments are required to better support the approach. The usual experimental setup includes at least two SAR images from different sensors/polarizations, and ENL = 1,... 4 will suffice. Also, technical information for the SAR data is manadtory (acquisition mode, date....).
Author Response
Dear Reviewer,
We appreciate your help and would like to thank you for your constructive and insightful comments that help to improve the quality of this paper.
We have carefully addressed all your comments and made considerable effort to improve the quality of this paper.
Our responses to your comments and questions are listed as follows.
Kind regards,
Haoran Jiang
- Regarding “This is not the usual way to express that images have been corrupted with speckle”, we have updated the sentence as below,
“It is proved that using multiplicative Nakagami distribution to add noise to simulate SAR images is effective. We add speckle noise to ,, and by using multiplicative Nakagami distribution model with a variance of 0.1”.
- We have added two other actual SAR images to validate the method and added the following sentences in the first paragraph in Results section.
“The first real image is Chinalake image with a resolution of 3 meters taken by a Ku-band radar. The second real SAR image is piperiver with a resolution of 1 meter. And the third real image is from the WHU-OPT-SAR data set, which was taken by the GF-3 satellite”.
- For Figures 9, 10, 19, and 20, we have revised these figures as suggested.
Round 2
Reviewer 1 Report
I think the authors have done an excellent revision. I highly recommended for publication.
Reviewer 4 Report
All concerns were properly attended.