Learning a Fully Connected U-Net for Spectrum Reconstruction of Fourier Transform Imaging Spectrometers
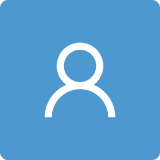
Round 1
Reviewer 1 Report
VERDICT: The paper should be accepted due to a novel data-driven way to invert interferograms. However, I am giving a major revision to account for some necessary changes that will make the manuscript more impactful.
SUMMARY: The paper tackles the problem of recovering spectral profiles from Fourier transform infrared spectrometric measurements (FTIS). The core contribution is in the form of a neural network that learns a mapping from the interferogram to the spectral profile. The paper shows that such a non-linear mapping significantly reduces the side lobes that occur due to a simple Fourier transform-based inversion. The authors have shown extensive examples to show that the proposed method is effective.
The paper is a fresh take on combining machine learning with spectral tools, and something that prior work has not looked into. The paper is well written, and the experiments are quite demonstrative.
OVERALL COMMENTS:
- I suggest a section on the effect of size of the FCUN to show how capacity affects the learned inversion. Such an experimental section will help readers to choose the desired number of parameters based on their own constraints
- Most experiments have been conducted with Gaussian noise but real world data is corrupted by Gaussian (readout) and Poisson (shot) noise. I recommend accounting for both noises.
- Along the same lines, please show results on low light (Poisson lambda <= 100), moderate light (<= 500) and high light conditions (> 1000). I recommend looking into the sensor characteristics (readout noise, quantum efficiency) of the camera that the authors have used for their real experiments.
OTHER MAJOR COMMENTS:
- Please mention figures of merit in abstract such as PSNR improvement over state-of-the-art, reduction in spectral angular mapping value, as well as reduction in computation time.
- L47 -- 'a great variety of ...'
- L81 -- please avoid using 'and so on'. You may terminate the sentence after ', remote sensing [22, ...'
- L80 - L 98 -- this reads more like prior work. You can mention that NNs have shown great promise and then move on to talk about the contributions of this paper.
- I suggest mentioning early on that the apodization function is the same as window function, which may be more accessible to readers in signal processing.
- L154 -- hyperspectral data mentioned first time without defining it
- Section 2 does a wonderful job of explaining the basics. I recommend extending it a little bit by visualizing the side lobes that are prevalent in simple FFT-based inversion.
- A pressing question is the following -- if eq. (3), (4) are physically accurate, what causes the side lobes? if the equations are imprecise, perhaps the authors can add what type of non-linearities exist. This would strongly validate the need for a learnable non-linear mapping as the non-idealities in real setups cannot be easily modeled.
- L217 -- parameters of what?
- RQE is same as MSE, which may be mentioned for accessibility
- Eq. (11) -- I recommend a different greek letter instead of \lambda, as the paper is about wavelengths and \lambda is a commonly used letter for that.
- L249 -- is unit OPD same as resolution of the system?
- L253 -- 'super imposing' has a different meaning -- usually it means to multiply two signals. I suppose the authors meant 'added'? Further, Gaussian alone is not sufficient, photon noise is a major source of noise too. in fact, FTIS has limited benefits in the presence of high photon noise.
- L295 -- minimum of training or validation loss?
- L307 -- avoid words like 'obviously'. Instead use 'It is evident from the plot that ...'
- Center table 3
- Fig 7(a) -- Refernce and FCUN result are not distinguishable. Use line styles to demarcate them easily
- Fig 8(a) -- same as 7th figure
- L340 - L350 -- the paragraph is meandering about a single point -- "FCUN can recover sharper peaks with suppressed sidelobes"
- Fig. 11 -- a wonderful result!
- Table 4 -- what about MPSDE?
- Figure 12 -- I recommend using peaky spectra such as CFL spectrum or a sum of lasers.
Author Response
Dear Editors and Reviewers:
Thank you for the reviewers’ comments concerning our manuscript entitled “Learning a Fully Connected U-Net for Spectrum Reconstruction of Fourier Transform Imaging Spectrometers” (ID: remote sensing-1548825). Those comments are all valuable and very helpful for revising and improving our paper, as well as the important guiding significance to our research. We have studied comments carefully and have made corrections which we hope meet with approval. Revised portions are marked in red on the paper. The revised manuscript is in the attachment.
We appreciate for Editors/Reviewers’ warm work earnestly and hope that the correction will meet with approval. If you think the modification is not good enough, we will continue to modify it. Once again, thank you very much for your comments and suggestions.
Author Response File: Author Response.pdf
Reviewer 2 Report
This paper proposed a spectral reconstruction method based on fully connected U-net. The contribution is adopting deep learning for spectral reconstruction, and building a dataset.
- There are some grammatic errors, and the tense use need to be harmonized.
- It is claimed that remote sensing data is used for evaluation, but the displayed image in Fig 14 and 16 are not remote sensing images.
- More reconstruction methods need to be compared.
Author Response
Dear Reviewer:
Thank you for the reviewer’s comments concerning our manuscript entitled “Learning a Fully Connected U-Net for Spectrum Reconstruction of Fourier Transform Imaging Spectrometers” (ID: remote sensing-1548825). Those comments are all valuable and very helpful for revising and improving our paper, as well as the important guiding significance to our research. We have studied comments carefully and have made corrections which we hope meet with approval. Revised portions are marked in red on the paper. The revised manuscript is in the attachment.
We appreciate for Reviewer's warm work earnestly and hope that the correction will meet with approval. If you think the modification is not good enough, we will continue to modify it. Once again, thank you very much for your comments and suggestions.
Author Response File: Author Response.pdf
Reviewer 3 Report
The authors propose a fully connected U-Net (FCUN) for spectrum reconstruction (SpecR) of Fourier transform imaging spectrometers (FTISs).
Unfortunately, the paper contains the following problems.
- All figures must contain a small paragraph explaining them. This paragraph must be placed right after the figure title.
- In the literature review part of the introduction section, the authors must explain the differences between all cited papers. These are papers 22, 23, 24, 25, 26, 27, 81 28 in lines 81,82 and papers 29, 31, 35 in line 102.
- In the literature review part, the authors must include a minimum number of 8 papers regarding FTIS.
- A section describing the architecture of a typical U-Net followed by a diagram and pseudocode must be included. This section must be placed before section 3.
- A pseudocode of the proposed method followed by a corresponding explanation must be included in section 3.
- In section 4, the authors must include a table summarizing the parameters used for the experiment runs.
- A separate discussion section must be included before the conclusion section.
- The paper language needs polishing since it contains syntax errors.
Author Response
Dear Reviewer:
On behalf of my co-authors, we thank you very much for giving us an opportunity to revise our manuscript, we appreciate the reviewer very much for their positive and constructive comments and suggestions on our manuscript entitled “Learning a Fully Connected U-Net for Spectrum Reconstruction of Fourier Transform Imaging Spectrometers” (ID: remote sensing-1548825).
We have studied the reviewer’s comments carefully and have made revisions which are marked in red in the paper. We have tried our best to revise our manuscript according to the comments. Attached please find the revised version and the point-by-point response to the reviewer’s comments, which we would like to submit for your kind consideration.
We would like to express our great appreciation to the reviewer for your comments on our paper. Looking forward to hearing from you.
Thank you and best regards.
Yours sincerely,
Tieqiao Chen.
E-mail: [email protected]
Author Response File: Author Response.pdf
Reviewer 4 Report
The paper proposes a trained deep neural network model (U-Net) for reconstructing the spectrum of Fourier transform imaging spectrometers from the interference curves. The paper is well written and has interesting results. The authors are suggested to address the following comments and modify the paper accordingly.
- It is not clear from the paper whether the results of the trained model presented in section 4.4 are part of the training set or test set. The authors need to explicitly mention the size of datasets used for training and test. Also, they need to report the accuracy of the trained model using the blind test dataset. Then specific examples from the test dataset need to be shown.
- It is mentioned in the paper that the authors used two types of datasets. However, it is not clear whether a single U-Net model is trained using the combined dataset or two different U-Net models are trained. Also, whether the test dataset is created by randomly selecting data from both datasets. The authors need to clarify these issues.
- The authors are suggested to provide the sizes of the inputs and outputs vectors of each layer of the U-Net as shown in figure 3.
- Why is the feature vector upsampled in the beginning for two layers, and then downsampled instead of adopting only downsampling layers in the beginning as shown in figure 3?
- The authors are suggested to check the entries of the 2nd column of table 1. It seems the entries of the two rows should interchange.
- The authors are suggested to show the 4 different plots of figure 9 in the same plot to bring out the qualitative comparison. Same suggestion for figures 10 and 11.
Author Response
Dear Reviewer:
On behalf of my co-authors, we thank you very much for giving us an opportunity to revise our manuscript, we appreciate the reviewer very much for their positive and constructive comments and suggestions on our manuscript entitled “Learning a Fully Connected U-Net for Spectrum Reconstruction of Fourier Transform Imaging Spectrometers” (ID: remote sensing-1548825).
We have studied the reviewer’s comments carefully and have made revisions which are marked in red in the paper. We have tried our best to revise our manuscript according to the comments. Attached please find the revised version and the point-by-point response to the reviewer’s comments, which we would like to submit for your kind consideration.
We would like to express our great appreciation to the reviewer for your comments on our paper. Looking forward to hearing from you.
Thank you and best regards.
Yours sincerely,
Tieqiao Chen.
E-mail: [email protected]
Author Response File: Author Response.pdf
Round 2
Reviewer 1 Report
The authors have extensively addressed all my concerns.
Reviewer 2 Report
The quality was improved after the revision. No more question.
Reviewer 3 Report
The authors addressed most of my comments.
Reviewer 4 Report
The authors have revised the manuscript as suggested and the provided explanations and modifications bring out the contribution and uniqueness of the proposed. The revised manuscript should be considered for publication.