Forecast of the Global TEC by Nearest Neighbour Technique
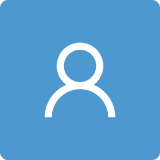
Round 1
Reviewer 1 Report
General Comments:
Overall, the manuscript is very well written, very interesting, and reports a novel method of predicting global VTEC values. The authors provide a very detailed review of previous methodologies along with their advantages and disadvantages, and compare their new method and highlight the differences. These show the authors’ deep understanding of previous works that possibly help them to design the new methodology described in detail. The authors put a lot of work and effort into the development of the new methodology using data from two solar cycles and into the validations that are also described in detail. The manuscript is certainly recommended for publication after some minor revisions focusing mainly on the figures (see detailed comments below).
Detailed Comments:
L19: “affect” should read “affects”
L22: “and” should read “or”
L45: “UPC-IonSAT” should be specified
L138: “Magnetic Field Index” should read “Magnetic Field Component” or just “Magnetic Field”
L157: “the years 2015 and 2015” Is the second 2015 correct?
L236: “VTEC should read “vertical TEC (VTEC)”
Figure 2: The map coordinates should be labelled. The color scale should be labelled as well (VTEC in TECU). The maps should be much larger. The color code should be modified if possible. The current one shows the shades of yellow, green, and blue. The scale becomes too dark at VTEC<10 TECU. There are too much dark areas in the maps where the features shown are almost invisible.
Figures 6-7-8 and 10 andA1-A2: Graphs are too small. Plots and labels are too small. Lines should be stronger in Figures 6-7.
Figure 9: There are no graph axes marked and labelled. There are no color codes and labels.
Figures 11-12 and A3: The graph axes are labelled but the font size is way too small. The plots should be larger and the lines stronger.
Author Response
General Comments:
Overall, the manuscript is very well written, very interesting, and reports a novel method of predicting global VTEC values. The authors provide a very detailed review of previous methodologies along with their advantages and disadvantages, and compare their new method and highlight the differences. These show the authors’ deep understanding of previous works that possibly help them to design the new methodology described in detail. The authors put a lot of work and effort into the development of the new methodology using data from two solar cycles and into the validations that are also described in detail. The manuscript is certainly recommended for publication after some minor revisions focusing mainly on the figures (see detailed comments below).
ANS: Thank you for your comments.
- a) L19: “affect” should read “affects”
ANS: corrected, thank you.
- b) L22: “and” should read “or”
ANS: corrected, thank you
- c) L45: “UPC-IonSAT” should be specified
ANS: We have added the following text:
(for more information about the IonSAT group, i.e., Ionospheric determination and navigation based on Satellite And Terrestrial systems group see \cite{IonSAT})
and at the references section:
\bibitem{IonSAT} Web page of IonSAT: IonSAT - Ionospheric determination and navigation based on Satellite And Terrestrial systems. Accessed on June 2 2021. [Online]. Available: \url{https://futur.upc.edu/IonSAT?locale=en}.
- d) L138: “Magnetic Field Index” should read “Magnetic Field Component” or just “Magnetic Field”
ANS: Now it reads: “Magnetic Field”, thank you.
- e) L157: “the years 2015 and 2015” Is the second 2015 correct?
ANS: Sorry for the typo, it has been corrected now it reads: “the years 2015 and 2018”.
Thank you.
- f) L236: “VTEC should read “vertical TEC (VTEC)”
ANS: Thank you for noticing the undefined acronym. Now the text reads: “vertical TEC (VTEC)”
- g) Figure 2: The map coordinates should be labelled. The color scale should be labelled as well (VTEC in TECU). The maps should be much larger. The color code should be modified if possible. The current one shows the shades of yellow, green, and blue. The scale becomes too dark at VTEC<10 TECU. There are too much dark areas in the maps where the features shown are almost invisible.
ANS: We are aware of the quality of the images in the first version of the manuscript.
1- We have recreated them, with a higher resolution, and have increased the size of the figures. The captions in the subplots are bigger and more clear. Thank you.
2- About the color code, during the preparation of the manuscript there was a debate related to either use the commonly used ‘jet’ scale, or the more perceptually adapted ‘viridis’ scale. (see attached document for the figures, taken from https://matplotlib.org/2.0.2/users/colormaps.html)
The problem with the ‘jet’ scale is that it is not monotonic with lightness, and creates perceptual ambiguities related to the placement of the maxima. Note that the middle scale in ‘jet’ (blue/yellow), seems more bright than the maximum value, i.e. the dark red, while in ‘viridis’, the change in color is proportional to the brightness.
We decided to use viridis, in order to make clear the differences at the middle/ higher part of the scale.
In section 2.4 we added the following paragraph:
Note that the figures use as color code the 'viridis' scale instead of the more usual 'jet' scale. The reason is that the 'viridis' color scheme implements a linear scale with brightness going from dark black to bright yellow linearly, while the 'jet' scale has the brighter colors at the middle of the scale (blue/yellow), and the lowest/highest values are coded with the darker colors. This non monotonicity of the relationship color/brightness creates ambiguities.
3- “The scale becomes too dark at VTEC<10 TECU.”
We have recreated the figures, and hope that now the lower part of the scale should be more easy to differentiate between levels.
4- In order not to clutter the subplots, we have added the following text to the caption:
The maps range in latitude from 90 degrees north to 90 degrees south, and in longitude from 180 degrees west to 180 degrees east. Color bars are in TEC units.
- h) Figures 6-7-8 and 10 and A1-A2: Graphs are too small. Plots and labels are too small. Lines should be stronger in Figures 6-7.
ANS: Figures 6-7-8 and 10 have been increased in size, and the details and labels are easier to read.
Figures A1-A2 have been recreated, are more clear. These figures are now located at the section ‘Materials and Methods’. In order not to clutter the subplots, we have added the following text to the caption:
The maps range in latitude from 90 degrees north to 90 degrees south, and in longitude from 180 degrees west to 180 degrees east. Color bars are in TEC units.
- i) Figure 9: There are no graph axes marked and labelled. There are no color codes and labels.
Figures 11-12 and A3: The graph axes are labelled but the font size is way too small. The plots should be larger and the lines stronger.
ANS:
1- Figure 9: There are no graph axes marked and labelled.
This has been done intentionally to highlight the area covered by the confidence margins, i.e., the regions of the map where the prediction lies inside the confidence margin. To clarify the reading of the figure we added the following text in the caption:
Forecast maps in which the basemap coincides with the global coordinates (latitude +-90 degrees, longitude +- 180), and the height shows measured TEC values. The colors distinguish, (green), the regions of the GIM map where the prediction is within the +- sigma range, and in red the regions where the prediction is outside. \emph{Green areas}: show the areas where the reference $ Forecast_{ref}$ is included in $Forecast_{Map} \pm Forecast^{Std}_{Map}$. \emph{Red areas}: areas where $Forecast_{ref}$ is outside the margin.
2- Figures 11-12 and A3: The size of the figures has been increased in size and the labels are easier to read. Also in order to adapt the paper to the format of the journal the plots have been transferred from the appendix section to the materials and methods section.
Author Response File: Author Response.pdf
Reviewer 2 Report
This paper introduces a a new method, called the Nearest Neighbour method for Global Ionospheric Maps of TEC forecasts. Actually, I have some major questions or comments about this paper.
1)The introduction is too short to include enough background of this research. I can not see any inportance of this reseach here.
2)This paper has too many sections or subsections. It is very hard to understand the connections of those small subsections. I recommend the authors to delete some small subsections and combine the similar contents, which can make this paper much more clear and readable.
3) Most of the figures are too small to recongnize or read. Readers may feel unreadable about those figures and can not obtain the results of authors' method.
I suggest that the authors should simplify the format of the original paper and maike it a little short but much clear. In such a case, I suppose to reject it in present form.
Author Response
This paper introduces a a new method, called the Nearest Neighbour method for Global Ionospheric Maps of TEC forecasts. Actually, I have some major questions or comments about this paper.
1)The introduction is too short to include enough background of this research. I can not see any inportance of this reseach here.
ANS: We have adapted the structure of the article to the format of the journal. In the introduction we have made a detailed analysis and discussion of the preceding methods of GIM prediction and explain the background of the research. This has made this section longer than usual. However, we believe it is important, as the method we propose is sufficiently different from the previous methods and has better performance, which justifies the discussion and comparison of each method.
2)This paper has too many sections or subsections. It is very hard to understand the connections of those small subsections. I recommend the authors to delete some small subsections and combine the similar contents, which can make this paper much more clear and readable.
ANS: We have reorganized the distribution of sections. We believe that by distributing the subsections into introduction, materials and methods, results and discussion, the context of each of the subsections will be better understood. It should be noted that we have tried to perform an exhaustive test in different scenarios of the method.
3) Most of the figures are too small to recognize or read. Readers may feel unreadable about those figures and can not obtain the results of the authors' method.
ANS: We agree with the comment, for this we have re-generated the figures, increasing the size, improving the quality, and the clarity of the letters/numbers in each subplot and in the captions
I suggest that the authors should simplify the format of the original paper and maike it a little short but much clear. In such a case, I suppose to reject it in present form.
ANS: First of all, we apologize for the fact that the initial organization of the article has made it difficult to read and review. We have reorganized the structure of the article and the figures to solve this issue.
As mentioned above new introduction section is longer than usual, but this is because we have made a systematic comparison of our proposed method with previous methods. This comparative is important, because due to the difference and simplicity of the model we propose, we believe it is important to provide a good justification of why it is proposed and the shortcomings of the previous methods.
The added value of this work consists in demonstrating the performance of the method at different times of the solar cycle, specific days of high activity and comparison with JASON satellite data and the standard CODE references. The results and discussion section is therefore long but serves to demonstrate that the proposed system performs well in various situations.
In addition, the method description part has been done in detail, to allow reproducibility of the results.
This is why we would like to keep the content of the article in the new format.
Author Response File: Author Response.pdf
Reviewer 3 Report
Manuscript Number: remotesensing-1583580
Full Title: Forecast of the Global TEC by Nearest neighbour technique
General comment
The paper submitted to Remote Sensing MDPI by Enric Monte-Moreno et al. proposed a method with two full years (2015 and 2018), for Global Ionospheric Maps of Total Electron Content forecasting using the Nearest Neighbour method.
The work is analyzed in original submission and is arranged in the following sections: 1. Introduction, 2. Issues regarding the TEC map prediction, 3. Precedents and limitations of the GIM forecast performances, 4. UPC-IonSAT real-time Global Ionospheric Maps and Data preprocessing, 5. NNGIM algorithm, 5. NNGIM algorithm, 6. Illustration of how the algorithm works, 7. Selection of the Benchmark, 8. Results, 9. Conclusion, Appendix A, Appendix B, Appendix C, References.
From a first reading-review, it appears to this reviewer which has not been edited according to the RS MDPI instructions for authors; with this structure, it is difficult to read and understand the methods, analyse the results, evaluate the discussion of them.
For this reviewer is unambiguous, however, that the study shows a considerable effort on the part of authors to implement the project and its potential applications. The content of the paper is potentially interesting, and with improvement, it might be published.
However, in its current form it is not acceptable, in my opinion (especially for a high quality journal like RS MDPI) for several reasons, that follow in the specific comments.
Specific comment
With regret, the paper shows some limitations that need to be deeply resolved by the authors with a new and completely revised version of the manuscript.
In particular the authors must follow these recommendations:
- read the authors' instructions and set up the paper according to the following scheme: Introduction, Materials and Methods, Results, Discussion, Conclusions. There is no firm standard way of writing an article, but the article should be readable by people in this field;
- the section “Introduction” should be literature review revised to be more in-depth and informative, with particular attention to other articles concerning similar approaches on the topic;
- third remark, figures: many of them are not comprehensible, because they are too small, with illegible captions;
- furthermore, the sequence of citations is often incorrect, and must follow the order in which they appear in the text;
- the results are not even commented with the Discussion section, which is mandatory in RS MDPI;
- the Appendices sections are not expected in the RS format and the content must be reported in the text;
- last but not least, references be revised according to the authors' instructions.
In conclusion the work deserves publication after major revisions, in my opinion. I will be available to authors for the assessment of the manuscript at the subsequent submission, I summarize in technical comment below.
Best regards
Technical comment
- line 17-31: this section is too small, I suggest to to include other articles in the literature, with particular attention to other articles concerning similar approaches on the topic;
- line 32: please insert here Materials and methods;
- line 52, “Appendix”: please remove, appendices are not required in the instructions for authors in RS MDPI;
- line 104, is still materials and methods? Please check and modify for the reader;
- line 134, [10], please check it, the sequence of citations is often incorrect, and must follow the order in which they appear in the text;
- line 163, please see previous comment 3;
- line 272, please see previous comment 3;
- line 291, see previous comment 3;
- line 416, see previous comment 3;
- figure 1, please modify the size, because the captions are unreadable for the reader;
- figure 2, please modify the size, because the captions are unreadable for the reader;
- line 448, now I believe that start “Result” section, please verify;
- figure 3, please modify the size, because the captions are unreadable for the reader;
- figure 5, please modify the size, because the captions are unreadable for the reader;
- figure 6, please modify the size, because the captions are unreadable for the reader;
- figure 7, please modify the size, because the captions are unreadable for the reader;
- figure 8, please modify the size, because the captions are unreadable for the reader;
- figure 12, please modify the size, because the captions are unreadable for the reader;
- line 669, I suggest to insert here “Discussion” section, which is mandatory in RS MPDI;
- line 705-734, appendices are not required in the instructions for authors in RS MDPI, please move into other part of the text;
- line 38-739, please check according RS instruction for authors;
- line 751, please check according RS instruction for authors;
- line 767, please check according RS instruction for authors;
- line 779, please check according RS instruction for authors.
Author Response
Full Title: Forecast of the Global TEC by Nearest neighbour technique
General comment
The paper submitted to Remote Sensing MDPI by Enric Monte-Moreno et al. proposed a method with two full years (2015 and 2018), for Global Ionospheric Maps of Total Electron Content forecasting using the Nearest Neighbour method.
The work is analyzed in original submission and is arranged in the following sections: 1. Introduction, 2. Issues regarding the TEC map prediction, 3. Precedents and limitations of the GIM forecast performances, 4. UPC-IonSAT real-time Global Ionospheric Maps and Data preprocessing, 5. NNGIM algorithm, 5. NNGIM algorithm, 6. Illustration of how the algorithm works, 7. Selection of the Benchmark, 8. Results, 9. Conclusion, Appendix A, Appendix B, Appendix C, References.
From a first reading-review, it appears to this reviewer which has not been edited according to the RS MDPI instructions for authors; with this structure, it is difficult to read and understand the methods, analyse the results, evaluate the discussion of them.
For this reviewer is unambiguous, however, that the study shows a considerable effort on the part of authors to implement the project and its potential applications. The content of the paper is potentially interesting, and with improvement, it might be published.
However, in its current form it is not acceptable, in my opinion (especially for a high quality journal like RS MDPI) for several reasons, that follow in the specific comments.
Specific comment
With regret, the paper shows some limitations that need to be deeply resolved by the authors with a new and completely revised version of the manuscript.
In particular the authors must follow these recommendations:
- read the authors' instructions and set up the paper according to the following scheme: Introduction, Materials and Methods, Results, Discussion, Conclusions. There is no firm standard way of writing an article, but the article should be readable by people in this field;
- the section “Introduction” should be literature review revised to be more in-depth and informative, with particular attention to other articles concerning similar approaches on the topic;
- third remark, figures: many of them are not comprehensible, because they are too small, with illegible captions;
- furthermore, the sequence of citations is often incorrect, and must follow the order in which they appear in the text;
- the results are not even commented with the Discussion section, which is mandatory in RS MDPI;
- the Appendices sections are not expected in the RS format and the content must be reported in the text;
- last but not least, references be revised according to the authors' instructions.
In conclusion the work deserves publication after major revisions, in my opinion. I will be available to authors for the assessment of the manuscript at the subsequent submission, I summarize in technical comment below.
Best regards
ANS:
Dear reviewer. First of all, we apologize for the major effort originated by the way the article has been organized. We have made the effort to modify the article resolving the points you mention.
The introduction in which we make a comparative study of the precedents to our work is longer than usual, (occupies 5 pages), but we consider that it is important to discuss and compare the other ways to solve the problem, because the method we propose is very different and provides predictions to longer horizons and confidence margins. In this introduction we discuss in detail why the limitations of the previous ways of dealing with the problem.
We have also generated the figures again, in a larger size to make them clearer and more readable.
A discussion section has been created in which we have organized the information related to the discussion of the results, including the explanation of why deep learning systems may have difficulties in the problem of predicting GIMs.
The format of the references has also been revised.
Technical comment
- line 17-31: this section is too small, I suggest to to include other articles in the literature, with particular attention to other articles concerning similar approaches on the topic;
ANS:
This is due to the fact that we have not followed the organization of the structure of the journal. We have modified the structure, and within the introduction section we have included the subsections that provide the appropriate information:
(a) Issues related to previous work in tec map prediction.
- b) Approaches and limitations to the GIM forecast.
In these two subsections we provide an extensive discussion of the precedents and problems encountered in this type of prediction. It is worth noting that we discuss in detail the problems related to deep learning implementations, which in contrast to our implementation, require much larger resources, have a shorter prediction horizon and do not provide confidence margins in the prediction.
The fact that the method provides confidence margins further distinguishes the method we propose, since at least to the best of our knowledge, there are no other methods that also provide confidence margins.
- line 32: please insert here Materials and methods;
ANS: We have incorporated this subsection into the introduction section, and have provided a more clear title for the section. In this subsection we enumerate previous attempts to solve the problem, and highlight problems that were encountered in papers that dealt with the topic of our own. We belief that this subsection belongs to the introduction, as we discuss the previous attempts, and frame the difficulties that are faced when doing TEC prediction.
- Previous title of the subsection: Issues regarding the TEC map prediction.
- New title of the subsection: Issues related to previous work in TEC map prediction.
- line 52, “Appendix”: please remove, appendices are not required in the instructions for authors in RS MDPI;
ANS: We have distributed the contents of the appendices in the appropriate parts of the text. We have kept the tables of hourly variation of the Kp, but for reasons of space and reading ease, we have eliminated the tables of solar flares per hour.
- line 104, is still materials and methods? Please check and modify for the reader;
ANS: Has been solved in the reorganization of the text. Thank you.
- line 134, [10], please check it, the sequence of citations is often incorrect, and must follow the order in which they appear in the text;
ANS: We have checked the order of the citations and the citations now appear in the correct order.
- line 163, please see previous comment 3;
- line 272, please see previous comment 3;
- line 291, see previous comment 3;
- line 416, see previous comment 3;
ANS: The points 6 to 9 have been solved.
- figure 1, please modify the size, because the captions are unreadable for the reader;
- figure 2, please modify the size, because the captions are unreadable for the reader;
ANS: All the figures have been generated again, and now the captions can be read.
- line 448, now I believe that start “Result” section, please verify;
- figure 3, please modify the size, because the captions are unreadable for the reader;
- figure 5, please modify the size, because the captions are unreadable for the reader;
- figure 6, please modify the size, because the captions are unreadable for the reader;
- figure 7, please modify the size, because the captions are unreadable for the reader;
- figure 8, please modify the size, because the captions are unreadable for the reader;
- figure 12, please modify the size, because the captions are unreadable for the reader;
ANS: All the figures have been generated again, and now the captions can be read.
- line 669, I suggest to insert here “Discussion” section, which is mandatory in RS MPDI;
- line 705-734, appendices are not required in the instructions for authors in RS MDPI, please move into other part of the text;
- line 38-739, please check according RS instruction for authors;
- line 751, please check according RS instruction for authors;
- line 767, please check according RS instruction for authors;
- line 779, please check according RS instruction for authors.
ANS: The above comments have been done. Thank you.
Author Response File: Author Response.pdf
Round 2
Reviewer 2 Report
The authors carefully re-orgnized the whole paper and made it much clear than the previous version. However, I still have some comments or questions about the current format.
1) Some numbers of subsections are wrong. e.g. two subsections 1.1, two subsections 2.1 ... Please correct them.
2) As for your NNGIM, can the authors give more descriptions about the paramter settings? The parameter NumNN was very important to the experiments and forecasting results. How about other parameters? It is better to show more dicsussions or any experiments to clarify the authors' results.
3) I do not understand the parameter WNeigh (Window of Neighbouring Months). Is it means that the NNGIM will choose the window for prediciton or training by defining the Wneigh? How to use this parameter to check the experiments for several days prediction or several months prediction?
4) Recently, a lot of deep learning or machine learning methods are used to predict the global TEC map. The authors may give more introductions or descriptions to show the advantages of NNGIMs or any comparisons. For example,
Rongxin Tang, Fantao Zeng, Zhou Chen, et al, The Comparison of Predicting Storm-time Ionospheric TEC by Three Methods: ARIMA, LSTM, and Seq2Seq, Atmosphere, 11(4):316, 2020.
Chen, Zhou & Jin, Mingwu & Deng, Yue & Wang, Jing-Song & Huang, Heng & Deng, Xiaohua & Huang, Chun-Ming. (2019). Improvement of a deep learning algorithm for total electron content (TEC) maps: image completion. Journal of Geophysical Research: Space Physics. 124. 10.1029/2018JA026167.
Wang, C., Xin, S., Liu, X., Shi, C., and Fan, L. (2018). Prediction of global ionospheric VTEC maps using an adaptive autoregressive model. Earth, Planetsand Space, 70(1), 1-14.
I recommend the authors to revised this paper again and make it better. It can possbily be considered to be accepted if the authors make some further major revision.
Best Wishes.
Author Response
Answer to reviewer 2.
The authors carefully re-organized the whole paper and made it much clear than the previous version. However, I still have some comments or questions about the current format.
1) Some numbers of subsections are wrong. e.g. two subsections 1.1, two subsections 2.1 ... Please correct them.
ANS:
The occurrence of multiple sections with the same numbering is an effect of the 'difflatex' application used to generate the difference latex between files. In the final version, the numbering is correct. Thank you for noticing this effect.
2) As for your NNGIM, can the authors give more descriptions about the paramter settings? The parameter NumNN was very important to the experiments and forecasting results. How about other parameters? It is better to show more dicsussions or any experiments to clarify the authors' results.
ANS:
We agree with your comment, the description of the setting and how the parameters influence is distributed in different parts of the text, which makes it difficult to have an overall perspective. To solve this problem, we have added the following text in subsection 2.2. The NNGIM forecasting algorithm: (thank you)
Parameter setting. The algorithm has two parameters to be adjusted, which are the window in months to select maps, denoted as $W_{Neigh}$ and the number of elements to calculate the mean value of the nearest neighbors, denoted as $Num_{NN}$. The criterion to adjust the parameters was to fit on a subset of the training base (the test was not used at any time for adjustment). In the case of $W_{Neigh}$, which corresponds to the neighboring months, it was observed that due to the variation of the algorithm itself, examples were always selected either from the current month or from the neighboring months. In order to limit the calculation needs, the calculation of distances was limited to the intervals determined by this variable. As for the $Num_{NN}$ variable, the result is different from the normal application of the Nearest Neighbour (NN) algorithm, in the sense that in order to compensate for the specific variability of each example used for the prediction, the number of neighbors to be used is much higher than in normal applications of the NN. In our case, the prediction error decreased monotonically until reaching a NumNNN value of about 500, producing a plateau of error with small oscillations of the error until reaching about 1500, and at this point the error starts to increase. Note that the fraction of elements used is small with respect to the total number of examples which exceeds one million.
To see the effects of adjusting these parameters, see Illustration of how the algorithm works (Subsection \ref{sec:Illustration}) and Example of forecasts at several horizons (Subsection \ref{appendix:ExampleForecastsAtSeveralHorizons}).
3) I do not understand the parameter WNeigh (Window of Neighbouring Months). Is it means that the NNGIM will choose the window for prediciton or training by defining the Wneigh? How to use this parameter to check the experiments for several days prediction or several months prediction?
ANS:
Your comment is partially answered in the previous section. Given the principle of operation of the algorithm, predictions at horizons longer than 48 hours are likely to provide the average value of the conditions in previous cycles, while the principle that the average of the nearest neighbors compensates for the specific peculiarities of each neighbor, leaving the common trend is no longer fulfilled. Since we have limited ourselves to forecasts up to 48 hours, we believe that the criterion of using the present month and the two neighboring ones is a good solution for the horizons we have considered.
The case of forecasting horizons of one month or more is very interesting, but we believe that the technique should be different from the one used in this work.
4) Recently, a lot of deep learning or machine learning methods are used to predict the global TEC map. The authors may give more introductions or descriptions to show the advantages of NNGIMs or any comparisons. For example,
Rongxin Tang, Fantao Zeng, Zhou Chen, et al, The Comparison of Predicting Storm-time Ionospheric TEC by Three Methods: ARIMA, LSTM, and Seq2Seq, Atmosphere, 11(4):316, 2020.
Chen, Zhou & Jin, Mingwu & Deng, Yue & Wang, Jing-Song & Huang, Heng & Deng, Xiaohua & Huang, Chun-Ming. (2019). Improvement of a deep learning algorithm for total electron content (TEC) maps: image completion. Journal of Geophysical Research: Space Physics. 124. 10.1029/2018JA026167.
Wang, C., Xin, S., Liu, X., Shi, C., and Fan, L. (2018). Prediction of global ionospheric VTEC maps using an adaptive autoregressive model. Earth, Planetsand Space, 70(1), 1-14.
ANS:
Thank you for the bibliographic indications. It is true that there are many publications related to TEC map prediction. In the introduction section, I dedicate almost 6 pages to discuss alternative approaches to the one presented in this paper. In the compilation of previous works and in section "4.4. Explanation of the limitation of saturating nonlinearities", I explain the principles why applications based on LSTM, Seq2seq (same limitation of LSTM), and deep learning are not suitable to perform TEC map prediction. The basic idea is summarized in Figure 15, which indicates that the input quantities are of long-tail type, and the learning of machine learning systems is based on gradient calculations. If the nonlinearities are of saturation type, the gradient is null, and therefore there is no learning precisely at the moments of interest. Regarding autoregressive models, I also make a discussion (which also affects a previous publication of ours), explaining the limitations of using past windows for prediction. There is a limit in performance beyond 6 hours of prediction.
That said, I would prefer not to specifically discuss other authors because since the technical criticism of the limitations of the systems is very harsh, the authors might feel personally blamed.
This has an explanation, the author E. Monte of this paper spent a year working on making Deep Learning based predictors, and is aware of the work and the amount of hours needed to create a system that has acceptable performance. Likewise, with systems of the autoregressive family, which apparently have a prediction wall between 6 and 12 hours.
Given the discomfort of pointing out the shortcomings of colleagues who have made a considerable and sincere effort to solve a problem, I would ask you not to quote them by making additional comparisons to those already made.
Author Response File: Author Response.pdf
Reviewer 3 Report
Dear Authors,
all the requests made by this reviewer have been made to the work, which is greatly improved.
I propose to accept in this form,
best regards
Author Response
Dear Reviewer,
Thank you for your answer,
best regards,
Enric Monte