A Multi-View Thermal–Visible Image Dataset for Cross-Spectral Matching
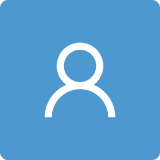
Round 1
Reviewer 1 Report
The article describes a new open access data set with RGB and thermal images. The data set is interesting. However, there are some issues with the article. In following, I make some remarks.
Do not use abbreviation (MTV) in the title. My personal opinion is that this is not a very well selected abbreviation at all (MTV=Music television is too strong brand), but it should be ok if you use it only inside the paper after defining it.
You are using a term “annotation” when you find tie points between images. I understand this, because you aim to use them for teaching neural network. However, you need to explain also that these are essentially measured tie points between RGB and thermal images. When you create your data set by utilizing manual measurements with PnP algorithm, they are not annotation points but tie points. However when you virtually compute corresponding points with non-bridge images then you might call them as annotation points.
Do not claim that your method is semi-automatic since you measure all true tie points manually. Instead, you might say that you are estimating virtually tie points between non-bridge images and thermal images. In addition, you don’t make just a few annotations (tie point measurements) if you have manually measured tie points from 898 images (pretty huge job >15*898 tie points).
In the Introduction, it remains unclear why 3D points cloud was needed. In addition, you could be clearer when you try to explain that in addition to bridge images you claim to get connection to non-bridge images.
Chapter 2 is not especially comprehensive. There are more datasets available. In addition, there are more research about algorithms to register RGB images and thermal images.
In figure 2, the legend of Step 1 has too small font. If you actually are using depth maps, you should insert an example to the workflow (now you have illustrated only a 3D point cloud). It is not clear how you select 3D points of reference model. Your image suggests that you have selected some corner points, but do you select them in 3D or from images. Is this a manual process? You need to include the description of this in the text.
You specifically mention that PSDK 102S images are utilized for making 3D model. However, you don’t mention if DJI H20T images are also within this process, it remains uncertain if you need to make a separate orientation of these images. Actually, the caption of figure 2 suggests this. Explain this clearly.
In page 7 line 186, you talk about having three kinds of images in two bands. What do you mean with ”two bands” (an RGB images have 3 bands and a thermal image has 1 band).
Page 7 line 196. Do not start a sentence with ”and”.
In page 8, you talk about generating markers. Your description how they are generated based on the constraints of point cloud visibility is not sufficient, and you need to add details. It very confusing that figure 7a shows just random 3D points that cannot be measured easily from images.
I really wonder why you didn’t make a relative orientation between thermal images and bridge images, and instead compute separate exterior orientations basing on 3D points. Or do I misunderstand something, since Hugin is designed to make relative orientations (for stitching panoramic images)? Your description can be read also in a way that you actually do both, which seems strange. Please, rewrite this part in such a way that there is no space for misunderstanding.
In page 10 line 240. ”the 2D points of the thermal related image” should most likely be RGB images.
Figure 9 is not illustrative. It’s surprising that you talk about dense matching when your task is just to find enough corresponding measurements. What you actually try to do is to project image observation vector to 3D space and from there to another image. This should be visible in your illustration. In addition this would be a good place where to show what you mean by “depth” in your research.
The major problem of the article is that equations 1 and 5 are both formally and functionally incorrect. Formally, there are uneven amount of brackets and image points should be row vectors.
Functionally, there are some critical issues. You start by turning the projection equation from image to ground which should be M=R0’inv(K)[x0 y0 1]’+T0. The first problem is that even if this looks like you can get a 3D point from 2D image observations, it does not happen. You can use this equation if you have more than 1 images to solve a 3D coordinate. Another problem is that the result M is not any longer in homogeneous coordinates. When you place the result in the projection equation of the second image, it doesn’t fit since it should be [x1 y1 1]’=K1[R –Rt;0 1][M 1]’, if you merge interior orientation matrix and projection matrix (as I assume you have done). I cannot find any justification why you multiply image observation (from the first image) with depth. In addition, you don’t explain if this is an Euclidean distance or ”scale distance” that is perpendicular to image plane. Since these are core equations of your data, there should be no doubts about these. Therefore show the full derivation of the equations or give a reference where it can be found, and of course present something that can actually work. In addition, I don’t like that you give names to new combined parameters before a reader can see what they include (e.g. R01, T01).
In page 10, you mention only the average re-projection error for the registered thermal images. You should include also standard deviation and maximum values.
You do not present any proof how well eq 1 is working (it’s not). This is crucial since all computed annotation points from non-bridge images are based on this. You should make computed projections to non-bridge images and compare the results to manually observed corresponding points.
In page 12 you describe the evaluation phase. However, you forget to mention what orientations you evaluate and against what you compare the results.
In eq 6 correct the typing of R_err
In the beginning of section 4, you should clearly explain in the beginning if you use provided annotation points for training neural networks (and if then what methods).
In page 15. ”minor human involvement” and ”small amount of manual annotation” are too optimistic in your case.
Author Response
Please see the attachment.
Author Response File: Author Response.pdf
Reviewer 2 Report
The paper proposes a semi-automatic annotation approach, which can generate large-scale and multi-view thermal-visible matching datasets with less manual intervention.
1) The advantage of this paper is that feature correspondences between the “bridge” and the query are manually annotated, which can produce a large and more accurate thermal-visible matching datasets. In addition, the geographical information of all thermal images and visible images is also provided.
2) The large-scale and multi-view datasets proposed in this paper can advance the development of deep learning in this field.
3) The paper is logically clear and the overall structure is complete.
However, there are some concerns to be further improved as well:
1) There are still some errors in the details. For example, on page 3( line 99), DTVA provides 80 aligned image pairs instead of 21 pairs.
2) The formula description is inaccurate. For example, on page 10, the explanation of formula (1) is wrong; some parameters of formula (6) are not explained.
3) Some sentences in the paper do not make sense and there are some spelling errors. For example, the name of the method in Figure 10 is "QuadTreeAttention, not "QuadAttention".
Author Response
Please see the attachment.
Author Response File: Author Response.pdf
Round 2
Reviewer 1 Report
In most parts, the Authors have managed to significantly improve the article. Unfortunately, there is still mistakes in the core of the paper leading to the incorrect equation 8. Now when you have written all equations visible, the place of error is visible. The error happens already in equation 4. You consider that the scale factor in projective space (in your notation z like in equation 6) equals to an Euclidean distance d(x,y) between an image point and corresponding 3D point. Unfortunately, this is not true. Because the use of Euclidean distance d(x, y) is not justified, it makes equations 5 and 8 incorrect.
Let’s take some numerical examples. Let’s build a virtual camera and a 3D point
K =
10 0 0
0 10 0
0 0 1
R =
1 0 0
0 1 0
0 0 1
t =
0 0 0
3D point:
M =
10 10 10
projection of 3D point M to image plane with K(R[X Y Z]’ + t gives
image_point_homogeneous =
100
100
10
image_point_cartesian =
10
10
1
Euclidean_distance =
17.32051
Euclidean distance was computed between the projection center and 3D points unlike in your figure 9 (since there is no justification to measure distances between image points and 3D points). Even in this case, 10 is not equal to 17.32051. Actually, in this simple special case if we use a perpendicular distance to image plane (distance to the scale plane in which the point lies along the z axis of the camera coordinate system) it gives the correct relationship 10=10, but I didn’t confirm if that works when we have rotations and translations. Let’s try a bit more complicated case:
R =
0.98007 0.19471 0.03947
-0.19867 0.96053 0.19471
0 -0.19867 0.98007
t =
2 5 0
K and M are the same.
image_point_homogeneous =
56.57
58.263
7.814
image_point_cartesian =
7.2396
7.4562
1
Euclidean_distance =
13.748
7.814 is not equal to 13.748.
You need to present equations that work, and to correct figure 9 to illustrate such distance that is suitable for your case.
Now I'm concerned if your results are valid if you have applied some equation that is not working.
Author Response
Please see the attachment
Author Response File: Author Response.pdf