Unsupervised Low-Light Image Enhancement via Virtual Diffraction Information in Frequency Domain
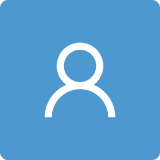
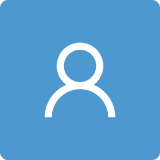
Round 1
Reviewer 1 Report
The article requires major revisions before it can be considered for publication. The following comments will help you improve the quality of your paper:
- When introducing related work, the paper briefly mentions CNN and GAN models. It would be beneficial to provide a more detailed explanation of how these specific methods are applied in low-light image enhancement. Additionally, discussing specific model techniques and mentioning the current state-of-the-art models used in the field would be valuable.
- While discussing the differences between model-driven and data-driven methods, it would be helpful to provide a clearer and more elaborate explanation of their respective strengths and weaknesses in the context of low-light image enhancement. Providing specific examples or case studies to support these points would further enhance the discussion.
- The paper could highlight the advantages and unique characteristics of the proposed model in comparison to other existing methods. For example, discussing whether the model provides better results in low-light image enhancement or exhibits enhanced robustness against noise and distortion. Additionally, mentioning the limitations or areas requiring further improvement would be valuable.
- Further discussion on how the proposed model addresses image consistency and noise issues would be valuable. Specifically, elaborating on which components or mechanisms contribute to maintaining image consistency, and explaining how the recursive gated deep convolutional neural network mitigates noise distortion and color degradation.
- There is a lack of detailed description regarding the motivations and effects of incorporating the recursive gated deep convolutional neural network, including how it enables high-order interaction and long-range modeling of image information. It could be worth exploring the effectiveness of transformer models for long-range modeling as well.
No
Author Response
Please see the attachment.
Author Response File: Author Response.docx
Reviewer 2 Report
the complexity of this method is high, I have seen many less-complexity methods that produce better quality results.
not all the mathematical equations are stated. the proposed method have gaps.
the authors need to compare their method with more contemporary methods.
the results tend to be reddish. the authors should correct the colors of the output images.
please measure the complexity using a proper method.
please give the runtimes for all the algorithms.
the future works are not clear.
its fine
Author Response
Please see the attachment.
Author Response File: Author Response.docx
Reviewer 3 Report
This paper studies the problem of image enhancement. The article has correct math formulation and a good-organized technical description. The paper's strong aspect is that a novel approach shows promising results in image enhancement cases with both global context and local details. Please find the comments and minor concerns below.
1) The network architecture needs to be described in more detail. It will be better to give a more detailed block diagram or algorithm for a proposed method.
2) In the loss function, the authors used the different balancing scales without numerical recommendations and any justification for these values.
3) The authors should have presented a complete objective quality comparison of test images to check the quality of their enhancement methodology. I recommend giving an estimation of non-reference measures whit more specified for image enhancement (for example, AME, EMEE, SDME, Visibility, TDME, BIQI, BRISQUE, or ILNIQE).
Author Response
Please see the attachment.
Author Response File: Author Response.docx
Round 2
Reviewer 2 Report
I have no further comments regarding this article.