Rethinking Representation Learning-Based Hyperspectral Target Detection: A Hierarchical Representation Residual Feature-Based Method
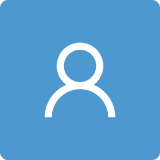
Round 1
Reviewer 1 Report
This paper focuses on Hyperspectral target detection with a hierarchical representation residual feature-based approach. Some detailed comments are listed as follows.
1. Does the model just utilize the band information (spectral feature) for feature representation? What about the spatial feature? Sparse representation had been adopted in target detection already, what is the main difference of your implementation? Why the three levels are used in band partition? Is the number of level related with the band number of different data sets?
2. Since the equation (17) should be the optimization solution of the Min function, the authors should give the specific operation of step 4 in algorithm 1.
3. In the experimental part, the author should compare your model with more recent methods. And the comparison of computation efficiency of different models should be listed too.
4. In section 4.4, the deficiencies of the proposed method should be discussed.
It's OK.
Author Response
Thank you very much for your in-detail reading of the manuscript. We would like to express our sincere appreciation and thankfulness for your insightful and valuable comments. We have made great efforts to answer your questions and further improve the quality of the manuscript by following your recommendations. We hope that the revised manuscript would be suitable to meet the publication standard of Remote Sensing. The attachment is a point-by-point response to your numbered comments. Again, we would like to express our sincere gratitude for your time and consideration. Comments to the Author: This paper focuses on Hyperspectral target detection with a hierarchical representation residual feature-based approach. Some detailed comments are listed as follows. Q1-1: Does the model just utilize the band information (spectral feature) for feature representation? What about the spatial feature? Sparse representation had been adopted in target detection already, what is the main difference of your implementation? Why the three levels are used in band partition? Is the number of level related with the band number of different data sets? Response to Q1-1: Many thanks for your valuable comments. This paper focuses on the problem of hyperspectral target detection (HTD). Hyperspectral image (HSI) can provide almost continuous spectral information to reveal the characteristics of the ground object, and the spectral resolution can reach 10 nm, which generates a big difference between different land covers and has unique advantages for target detection. As a result, this paper focuses on using spectral information of HSI for target detection. As the reviewer commented, HSI contains important spatial information. Our work has verified our idea for HTD with spectral information. Future work will consider further introducing spatial information for HTD. As the reviewer commented, sparse representation had been adopted in target detection. Our work differs from the traditional methods in the following several aspects. Firstly, traditional representation learning-based HTD methods generally follow a learning paradigm of single-layer or one-step representation residual learning and target detection on the original full spectral bands, which, in some cases, cannot accurately distinguish the target pixels from variable background pixels via such a one-round of detection process. As a result, this paper proposes to make full use of the latent discriminate characteristics in different spectral bands and the representation residual, and developed a novel level-wise band-partition-based hierarchical representation residual feature learning method for HTD. The idea is rational and its performance is verified on different HSI datasets in comparisons to some state-of-the-art methods. Since HSI contains dozens, tens, or even husbands of spectral bands. Different spectral bands might provide useful information for distinguishing different ground objects. As a result, this paper proposes to partition the original full spectral bands. The level of band-partition L can be 2i with i =0,1, 2.... This paper mainly studied the influence of L when i =0,1,2. The results show that the idea of combination of different levels of spectral band-partition helps improve the HTD performance. Although experimental results show that varying L has slight influence on the performance of proposed method on different datasets, better and more stable performance can be achieved with band-partition strategy. Thanks! Q1-2: Since the equation (17) should be the optimization solution of the Min function, the authors should give the specific operation of step 4 in algorithm 1. Response to Q1-2: Many thanks for your valuable comments. In equation (17), ‖∙‖p is the p-norm used to regularize the corresponding representation, and p can generally be 0, 1, or 2 for l0, l1, or l2-norm regularization. Different values of p will lead to different optimization problems. For example, when p=2, a closed-form solution can be achieved. As the reviewer suggested, the specific operations of step 4 have been presented in equation (17) and algorithm 1when p=2. Many thanks for your comments! Q1-3: In the experiment part, the author should compare your model with more recent methods. And the comparison of computation efficiency of different models should be listed too. Response to Q1-3: Many thanks for your valuable comments. The proposed LBHRF HTD method is evaluated in comparison to the several advanced HSI target detectors, including constrained energy minimization (CEM) [10], sparse representation-based target detector (SRD) [15], sparse representation-with binary hypothesis detector (SRBBH)[18], binary-class collaborative representation-based detector (BCRD) [16], sparse and dense hybrid representation-based detector (SDRD) [17], and single-spectrum-driven binary-class sparse representation target detector (SSBRTD)[25]. In summary, the comparing methods include classic target methods, i.e., CEM, and the state-of-the-art representation learning based methods, i.e., SRD, SRBBH, BCRD, SDRD and SSBRTD. Most of these comparing methods are relevant to our work and published in recent years. Generally speaking, as shown in Table 1, our proposed method cost more because of multi-layer hierarchical representation residual feature learning based on band-partition. We will further study the efficient computation of hierarchical representation residual features in future work, and reduce computation costs. Many thanks! Table 1. Running time (in seconds) of different methods on the AVIRIS I data set. Methods CEM SRD SRBBH BCRD SDRD SSBRTD LBHRF Time cost 7.17 4.14 6.10 6.54 331.36 13.6770 719.26 References 10. Q. Du, H. Ren and C.-I Chang, A comparative study for orthogonal subspace projection and constrained energy minimization, IEEE Transactions on Geoscience and Remote Sensing, 2003, 41(6): 1525-1529. 15.Y. Chen, N. M. Nasrabadi and T. D. Tran, Sparse Representation for Target Detection in Hyperspectral Imagery, IEEE Journal of Selected Topics in Signal Processing, 2011, 5(3): 629-640. 16.D. Zhu, B. Du and L. Zhang, Binary-Class Collaborative Representation for Target Detection in Hyperspectral Images, IEEE Geoscience and Remote Sensing Letters, 2019, 16(7): 1100-1104. 17.T. Guo, F. Luo, L. Zhang, X. Tan, J. Liu and X. Zhou, Target Detection in Hyperspectral Imagery via Sparse and Dense Hybrid Representation, IEEE Geoscience and Remote Sensing Letters, 2020, 17(4): 716-720. 18.Y. Zhang, B. Du and L. Zhang, A Sparse Representation-Based Binary Hypothesis Model for Target Detection in Hyperspectral Images, IEEE Transactions on Geoscience and Remote Sensing, 2014, 53(3): 1346-1354. 25. D. Zhu, B. Du and L. Zhang, Single-spectrum-driven binary-class sparse representation target detector for hyperspectral imagery, IEEE Transactions on Geoscience and Remote Sensing, 59(2):1487-1500, 2021. Q1-4: In section 4.4, the deficiencies of the proposed method should be discussed. Response to Q1-4: Many thanks for your valuable comments. This paper proposes to discover more discriminate information from representation residual for hyperspectral target detection, using band-partition strategy. The main deficiency of the proposed method is the estimation of background. For a fair comparison between different detectors, the widely used dual concentric window strategy is adopted to estimate the background characteristic around each test pixel. The dual concentric window strategy for background estimation is time-consuming. A universal background dictionary, which can reflect characteristics of the background will be considered in future work. Many thanks for your comments!Author Response File: Author Response.pdf
Reviewer 2 Report
the manuscript titled: Rethinking Representation Learning-based Hyperspectral Tar-2 get Detection: A Hierarchical Representation Residual Feature-3 based Method, proposes a hyperspectral target detection approach.
In general the work is well done with a good description of the theoretical aspects.I suggest a minor review before posting:
Table 1 images appear too small in this format. I suggest going up in size. Regarding the LBHRF method I suggest to the authors a richer explanation of the result obtained.
Conclusions
Given the very interesting results obtained by the authors, I suggest the authors also add a short paragraph on possible future developments.
Author Response
Thank you very much for your in-detail reading of the manuscript. We would like to express our sincere appreciation and thankfulness for your insightful and valuable comments. We have made great efforts to answer your questions and further improve the quality of the manuscript by following your recommendations. We hope that the revised manuscript would be suitable to meet the publication standard of Remote Sensing. The attachment is a point-by-point response to your numbered comments. Again, we would like to express our sincere gratitude for your time and consideration. Comments to the Author: the manuscript titled: Rethinking Representation Learning-based Hyperspectral Target Detection: A Hierarchical Representation Residual Feature-3 based Method, proposes a hyperspectral target detection approach. Q2-1: In general, the work is well done with a good description of the theoretical aspects. I suggest a minor review before posting: Response to Q2-1: Many thanks for your valuable comments. We have carefully taken the reviewer’s comments when preparing our revisions. Thanks! Q2-2: Table 1 images appear too small in this format. I suggest going up in size. Regarding the LBHRF method I suggest to the authors a richer explanation of the result obtained. Response to Q2-2: Many thanks for your valuable comments. As the reviewer suggested, the images in Table 1 have been enlarged as much as possible for better visibility. Q2-3: Conclusions. Given the very interesting results obtained by the authors, I suggest the authors also add a short paragraph on possible future developments. Response to Q2-3: Many thanks for your valuable comments. This paper revisits the prestigious representation learning-based HTD methods, and proposes to divide and congregate different levels of sub-band spectral bands combinations for multi-level and multi-layer residual feature learning and augmentation. Thus, the discriminate information that are beneficial for distinguishing targets from background can be accumulated in the obtained augmented residual feature. The proposed method mainly considers the spectral information in hyperspectral image, which can provide almost continuous spectral information to reveal the characteristics of the ground object. The spectral resolution can reach 10 nm, and it possesses dozens, tens, or even hundreds of spectral bands, which generates a big difference between different land covers and has unique advantages for target detection. Hyperspectral image also contains important spatial information. Future work will consider further introducing spatial information for hyperspectral target detection. In addition, the construction of a more efficient universal background dictionary will be studied in future work. Many thanks!Author Response File: Author Response.pdf
Reviewer 3 Report
Rethinking hyperspectral image classification, recognition of subtle differences and changes for clustering hyperspectral images, hyperspectral anomaly detection, etc. is a hot area of current research. Thus, this article deserves special attention. The authors propose a novel level-wise band-partition-based hierarchical representation residual feature (LBHRF) learning method for hyperspectral target detection. The article is interesting to read. However, it is overloaded with long acronyms and typos. Authors are encouraged to compile the Nomenclature Section and reduce the number of acronyms if they are not mentioned more than once.
Typos
L. 56-57. generalized likelihood ratio (GLRT) -> generalized likelihood ratio test (GLRT)
L. 120. show the proposed method -> show that the proposed method
L. 140. ?with representation -> ? with representation
L. 140. at and ab must not be Bold.
L. 143. coefficient a -> coefficient vector a
L. 271. y must be Bold and L must be Italic.
PP. 12-13. The probability of false alarm (PF) and probability of detection (PD) have subscripts in Figs. 8-9.
L. 396. As the Fig.8 shows, -> As Fig. 8 shows,
L. 401. in Fig.9 approach -> in Fig. 9 approach
L. 402. in Fig.9 correspond -> in Fig. 9 correspond
L. 402-403. the Fig.9 also -> Fig. 9 also
L. 445. L=0 L must be Italic.
L. 446. K=0 K must be Italic.
L. 458. in Fig.11. -> in Fig. 11.
L. 470. in Fig.11. -> in Fig. 11.
The quality of the English language is normal, but there are too many typos.
Author Response
Thank you very much for your in-detail reading of the manuscript. We would like to express our sincere appreciation and thankfulness for your insightful and valuable comments. We have made great efforts to answer your questions and further improve the quality of the manuscript by following your recommendations. We hope that the revised manuscript would be suitable to meet the publication standard of Remote Sensing. The attachment is a point-by-point response to your numbered comments. Again, we would like to express our sincere gratitude for your time and consideration. Q3-1: Rethinking hyperspectral image classification, recognition of subtle differences and changes for clustering hyperspectral images, hyperspectral anomaly detection, etc. is a hot area of current research. Thus, this article deserves special attention. The authors propose a novel level-wise band-partition-based hierarchical representation residual feature (LBHRF) learning method for hyperspectral target detection. The article is interesting to read. However, it is overloaded with long acronyms and typos. Authors are encouraged to compile the Nomenclature Section and reduce the number of acronyms if they are not mentioned more than once. Response to Q3-1: Many thanks for your valuable comments. We have carefully taken the reviewer’s comments into consideration when preparing our revisions. We have tried our best to reduce long acronyms and typos, and reduce the number of acronyms. In addition, we have carefully proofread the paper, and revised the presentation quality and language from the whole paper by the professional researchers with rich publication experience. We believe that in the current version, the presentation quality with better readability can satisfy the publication standard of such a top journal. Thank you very much! Q3-2: Typos L. 56-57. generalized likelihood ratio (GLRT) -> generalized likelihood ratio test (GLRT) L. 120. show the proposed method -> show that the proposed method L. 140. ?with representation -> ? with representation L. 140. at and ab must not be Bold. L. 143. coefficient a -> coefficient vector a L. 271. y must be Bold and L must be Italic. PP. 12-13. The probability of false alarm (PF) and probability of detection (PD) have subscripts in Figs. 8-9. L. 396. As the Fig.8 shows, -> As Fig. 8 shows, L. 401. in Fig.9 approach -> in Fig. 9 approach L. 402. in Fig.9 correspond -> in Fig. 9 correspond L. 402-403. the Fig.9 also -> Fig. 9 also L. 445. L=0 L must be Italic. L. 446. K=0 K must be Italic. L. 458. in Fig.11. -> in Fig. 11. L. 470. in Fig.11. -> in Fig. 11. Response to Q3-2: Many thanks for your valuable comments. In addition to the typos raised by the reviewer, we have carefully checked the manuscript and carefully corrected possible typos. Besides, the manuscript has been thoroughly proof-read by an experienced technical writer. The reviewer's comments have improved the quality and readability of the paper. Thank you very much!Author Response File: Author Response.pdf
Round 2
Reviewer 1 Report
It's OK now.