MASA-SegNet: A Semantic Segmentation Network for PolSAR Images
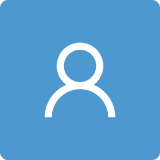
Round 1
Reviewer 1 Report
This paper proposes an algorithm aimed at enhancing the distinguishability of different scattering features in PolSAR semantic segmentation, while mitigating the impact of noise. The proposed approach consists of two main components. Firstly, the authors introduce a pre-processing method for PolSAR image data, which involves the fusion of multi-source data and pseudo-color mapping. This preprocessing step aims to enhance the differences between scattering features, thus improving the subsequent segmentation task. Secondly, the authors propose a multi-axis sequential attention segmentation network (MASA-SegNet) specifically designed for PolSAR semantic segmentation. The encoder of MASA-SegNet incorporates a feature extractor that effectively leverages PolSAR features at multiple scales. This is achieved by employing multi-axis serial attention blocks, which address inter-class similarity and intra-class variation caused by scattered noise. Additionally, the network employs a serialized residual connectivity design process to propagate spatial information, enhancing the overall spatial awareness of MASA-SegNet. The decoder of the network is responsible for performing the semantic segmentation task. To evaluate the proposed algorithm's effectiveness in semantic segmentation, the authors conduct feature visualization experiments. These experiments demonstrate the ability of the spatial sequence attention mechanism to effectively extract features and reduce noise interference. The algorithm's performance is evaluated on two large-scale public datasets, providing empirical evidence of its superiority in semantic segmentation.
In summary, this paper presents a comprehensive algorithm that combines a preprocessing method for PolSAR data and a specialized network architecture for semantic segmentation. The experimental results highlight the algorithm's ability to extract meaningful features and effectively address noise interference, showcasing its potential for PolSAR semantic segmentation tasks. The paper is interesting and holds promising application perspectives. However, there are some issues that need to be properly addressed. The major concerns include the following:
(1) The introduction lacks sufficient information and only a limited number of state-of-the-art deep learning methods are mentioned. The authors should conduct extensive research on semantic segmentation methods for PolSAR from the past 5 years. They should categorize these methods into different types, analyze the strengths and weaknesses of each category, and systematically highlight the main innovative contributions of this paper.
(2) When professional terms appear for the first time, English abbreviations are given directly, which is not easy to understand. The author needs to give the full English name and abbreviation in parentheses for easy reading and understanding.
(3) When designing the network structure diagram in Figure 3, it is important for the authors to ensure consistency in the expression of channel numbers. For example, some channel numbers are represented as 2c, 4c, 8c, while others are expressed as 256, 48. It is recommended to use a consistent and clear notation throughout the diagram. At the same time, some structures in Figure 3 are not given explanations, which is not conducive to the readers' understanding.
(4) The authors should provide an explanation as to why low-level features are selected from the features obtained from the second block rather than the lower-level features obtained from the first block. This rationale will help readers understand the design choice and the impact it has on the overall network performance.
(5) When visualizing the experimental results, it is important for the authors to include the visualization results of other methods. This will allow readers to gain a visual understanding of the results obtained by different methods.
(6) Experiments are insufficient, and only a limited number of more advanced SAR semantic segmentation methods are used for experimental comparisons. It is necessary to include a broader range of advanced methods to more effectively validate the superiority of the proposed approach. Furthermore, considering that the authors' network design incorporates multiple modules and structures, it would be beneficial to conduct ablation experiments for a more comprehensive evaluation.
(7) The authors claim to propose a preprocessing method for PolSAR image data. However, the paper lacks comparative experiments to validate the superiority of their proposed preprocessing method. Additionally, the authors do not provide accuracy comparisons or visualization data to support their claim that the network is capable of suppressing image noise.
Author Response
Please see the attachment.
Author Response File: Author Response.docx
Reviewer 2 Report
Overall, the manuscript titled " MASA-SegNet: A Semantic Segmentation Network for PolSAR Images" presents a convincing approach that addresses the challenges in satellite remote sensing imaging. The authors propose the MASA-SegNet network, which incorporates spatial position information and reduces speckle noise for semantic segmentation of PolSAR images. The manuscript provides clear improvements for a specific terrain category. I recommend publication of this article, as I believe the authors can address the minor comments and suggestions provided below.
1. Please consider adding an "Abbreviation" section at the end of the manuscript, listing all abbreviations used by the authors in manuscript.
2. It is suggested to use the same name for the terrain categories (color information) in both the FUSAR-MAP and AIR-PolSAR-Seg datasets for consistency.
3. Figures 3 to 5 should have increased font sizes or larger images to enhance visibility for readers.
4. Ensure consistent usage of script notation for equations in both the text and figures, e.g., consistently use "H" or "h," "W" or "w" in Chapter 1.2.2 and Figure 3. Maintain a uniform editing style throughout the document.
5. In line 175, please italicize "b" and "q". Similarly, in the evaluation metrics part of Chapter 2, ensure consistent italicization. Check for correct upper/lower case usage for scripts, e.g., "pij" or "Pij".
6. In the evaluation metrics part of Chapter 2, unify the use of metrics between MPA, AA, or PA.
7. Consider the term "VGG-SegNet" in line 245 as original authors used.
8. In line 259, specify "Fig. 6(a) and 6(b)" instead of "Fig. 6ab".
9. Revise to "exists" in line 259.
10. Provide titles for the rows and columns of the image arrays in Figures 6 and 7.
11. In lines 139 and 147, consider using phrases such as "as shown in" or parentheses.
12. Revise to "different scattering feature" in line 5 for improved accuracy.
13. Replace "convolutional" with appropriate context-specific terms in lines 165 and 183.
14. Ensure correct symbol for multiplication in line 211, not x in alphabet.
15. Include the pixel size for each image in Figure 5.
16. Increase the size of Figure 8 for better readability and visibility.
17. In Chapter 3.2, ensure that subtitles (1) and (2) have the same editing style as the subtitles in Chapter 1.2.2.
The English is fine, but the manuscript needs to be reviewed before final submission to incorporate the differences between the different authors who wrote the text.
Author Response
Please see the attachment.
Author Response File: Author Response.docx
Round 2
Reviewer 1 Report
It's a pleasure to accept the paper.