Combined Retrievals of Turbidity from Sentinel-2A/B and Landsat-8/9 in the Taihu Lake through Machine Learning
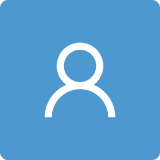
Round 1
Reviewer 1 Report
This article provides an estimation of turbidity values based on satellite images from the Sentinel-2A/B and the Landsat-8/9. Both of them are equiped with multispectral instruments. Measurements were applied to shallow lake waters of Taihu Lake in China. The autors checked three methods of atmospheric corrections for reflectance. Moreover they compared the estimated turbidity values received by machine learning methods with in-situ measurements.
In my opinion, the article contains valuable material that is worth publishing. The choice of the journal to which the authors submit their article is appropriate.
I have just a few minor comments on this article that should improve its quality before publication.
- It's not often that you find some funny mistakes in scientific articles. But one of your mistakes is funny. In place of FNU (Formazine Nephelometric Unit) you have put FUN (line 177).
- I know the table format in MDPI and I once wondered how to make a readable table without vertical lines. In my opinion, Table 1 is illegible at first glance. There is no vertical line in the middle to separate the data from the two satellites. It's worth improving. Maybe a narrow empty column in the middle would make it better.
- I don't like the caption of Table 2. You started the sentence with a lowercase letter. You don't explain what Std is, (I'm guessing it's standard deviation) and you don't refer to the contents of the table in the text. Also, the term "field turbidity" is misleading. It suggests that it is measured turbidity, while it is turbidity that has been estimated by satellite measurements.
- You should check the format of the tags you use. For example, you should use italic for Rrs (remote sensing reflectance) in your text. In lines 225 and 229 it is not italic.
- I don't like your Figure 5. You used straight line connections between the measurement points, highlighting the differences between the MSI and OLI spectra. I think it's important to show "points and lines" here. Then it will be seen that the difference in shapes results from the different arrangement of spectral channels.
- In figures 1, 7, 9 and 10 you use very different font sizes. Some are several times larger than others. Particularly small are: longitudes and latitudes, the scale of distances. Particularly large is the inscription "China" in Figure 1, which I do not criticize. According to the MDPI requirements, only one font and one size should be used in all drawings.
- I think the quality of English is good. The article is understandable. However, I am not a native English speaker, and since I always receive comments about the quality of English in my articles, I have indicated that "I am not qualified to assess the quality of English in this paper."
- I wish you success in the publication of this article and in your future work.
Author Response
Response to Reviewer 1 Comments
Point 1: It's not often that you find some funny mistakes in scientific articles. But one of your mistakes is funny. In place of FNU (Formazine Nephelometric Unit) you have put FUN (line 177).
Response 1:Thank you for your careful reading of our manuscript, we have corrected this error.
Point 2: I know the table format in MDPI and I once wondered how to make a readable table without vertical lines. In my opinion, Table 1 is illegible at first glance. There is no vertical line in the middle to separate the data from the two satellites. It's worth improving. Maybe a narrow empty column in the middle would make it better.
Response 2: Thanks for your suggestion, we have added an empty column between the two columns in Table 1.
Point 3: I don't like the caption of Table 2. You started the sentence with a lowercase letter. You don't explain what Std is, (I'm guessing it's standard deviation) and you don't refer to the contents of the table in the text. Also, the term "field turbidity" is misleading. It suggests that it is measured turbidity, while it is turbidity that has been estimated by satellite measurements.
Response 3: I’m sorry that we made sorry some mistakes in the caption of Table 2. We have changed the caption to “Statistics of turbidity from eleven stations matched with satellites. Std denotes the standard deviation”. In addition, your may misunderstood the sources of turbidity in Table 2. They are indeed measured turbidity from stations, “Sensor” in the first column represents the field turbidity matched with MSI and OLI in the same day.
Point 4: You should check the format of the tags you use. For example, you should use italic for Rrs (remote sensing reflectance) in your text. In lines 225 and 229 it is not italic.
Response 4: I’m sorry that we did not double check our manuscript before submission and thank you for your careful checking of our manuscript. We have corrected these errors in the new manuscript.
Point 5: I don't like your Figure 5. You used straight line connections between the measurement points, highlighting the differences between the MSI and OLI spectra. I think it's important to show "points and lines" here. Then it will be seen that the difference in shapes results from the different arrangement of spectral channels.
Response 5: Thanks for your suggestion, we have changed Figure 5 from a line chart to a dotted line chart.
Point 6: In figures 1, 7, 9 and 10 you use very different font sizes. Some are several times larger than others. Particularly small are: longitudes and latitudes, the scale of distances. Particularly large is the inscription "China" in Figure 1, which I do not criticize. According to the MDPI requirements, only one font and one size should be used in all drawings.
Response 6: We have used the same font and size in Figure 1, 7, 9, and 10.
Reviewer 2 Report
Turbidity is an important index of water quality monitoring. For inland waters, the traditional field sampling method has great limitations. In this paper, the machine learning method is used to invert turbidity. It's an interesting job. I have some comments on this article:
line 139: “we tested three mature AC methods: Case-2 Regional Coast Colour (C2RCC) processor, Dark Spectrum Fitting (DSF) algorithm, and Rayleigh Correction (RC).” It is important to note that RC is not the name of an algorithm and does not represent a specific algorithm. For water color remote sensing, RC has a special meaning and is a professional vocabulary, which is different from algorithms such as C2RCC and DSF.
line 147-153: I’m not sure where the RC in this manuscript come from, the authors should have explicitly written what algorithm (or software) the RC come from.
Chapter 3.1: Only the AC results of MSI-OLI intercomparisons of reflectance are shown in the manuscript, but for water color remote sensing, the in-situ data is important. The authors should show us the results of AC comparisons between in-situ and image, which are related to the robustness and credibility of the inversion algorithm.
And did the authors consider the spectral response function of the sensor when evaluating AC performance? MSI is very different from OLI.
Figure 1: Almost all the field stations in the manuscript are at the edge of the land and water. Can the spatial distribution of these points represent the entire Taihu Lake? In addition, the field point close to the land-water boundary is not suitable for the outcome tests due to the influence of the adjacency effect (even if the adjacency correction is used in the AC algorithm). For this reason, the test results of the retrieval model in this manuscript are questionable.
line 166-167: This paper uses a total training sample of 193+101 data to train the machine learning model. Can this amount of data guarantee the stability of the model? I have my doubts about the credibility of the results. Also, the manuscript is missing a lot of details about the retrieval AI model (the description in Chapter 2.5 is too sketchy).
Figure 4 shows that the higher the Rrc’ (865) value, the greater the difference between MSI and OLI. In general, the higher the reflectance values in the 865 nm band, the more turbid the water is. Therefore, do the results of Fig. 4 imply that there is a large difference between MSI and OLI results in highly turbid waters?
From Fig. 4(Rrc 865) & Fig. 5, whether the Rrc difference between MSI and OLI in the 865 band will affect the accuracy of the model. Whether different band combinations can be established to verify each other.
From Fig. 7, As shown in the screenshot, the turbidity of water bodies in the central area of Taihu Lake, MSI and OLI obtained by the model in this paper has a large difference, while the turbidity of the northern waters has little difference. It may be due to problems with the color card display, or it may be due to differences between MSI and OLI when the turbidity is greater than 150 NTU.
From Fig. 8, the fitting effect of MSI and OLI has a large deviation in the range of 150-200 NTU. Does it mean that the image of the two sensors is different in the application range of NTU?
line 350-351: The author describes it as "Turbidity was highest in summer and lowest in summer" . This should be a description error. Please check it.
line 217-218: The author will compare the Rrs processed by DSF and C2RCC with the Rrc processed by "RC". Is this comparable and on what basis?
From Fig. 3, This very well reflects the consistency of MSI and OLI, but this paper does not reflect which AC algorithm is more suitable for the waters of Taihu Lake. Could you please add clarification.
line 217-218: In this paper, a station in the lake is selected as the verification, which I think is insufficient, and multiple areas in the lake should be selected for comparison and verification.
I think the flow chart should be added in this paper to describe the work content more clearly.
Comments for author File: Comments.pdf
Author Response
Response to Reviewer 2 Comments
Point 1: line 139: “we tested three mature AC methods: Case-2 Regional Coast Colour (C2RCC) processor, Dark Spectrum Fitting (DSF) algorithm, and Rayleigh Correction (RC).” It is important to note that RC is not the name of an algorithm and does not represent a specific algorithm. For water color remote sensing, RC has a special meaning and is a professional vocabulary, which is different from algorithms such as C2RCC and DSF.
Response 1:Thank you for your suggestion, our intention is to simplify writing. We removed the abbreviation RC and replaced RC-SWIR with Rayleigh-SWIR.
Point 2: line 147-153: I’m not sure where the RC in this manuscript come from, the authors should have explicitly written what algorithm (or software) the RC come from.
Response 2: We added the Rayleigh correction used on what software:
“Rayleigh Correction is also available on the ACOLITE software.”
Point 3: Chapter 3.1: Only the AC results of MSI-OLI intercomparisons of reflectance are shown in the manuscript, but for water color remote sensing, the in-situ data is important. The authors should show us the results of AC comparisons between in-situ and image, which are related to the robustness and credibility of the inversion algorithm.
Response 3: I agree with you that in situ data is very important to verify the accuracy of the atmospheric correction algorithm. Unfortunately, we currently have no in situ data for validation. Based on the following point of view, we believe that the AC results of MSI-OLI intercomparisons of reflectance can reflect the accuracy of the atmospheric correction algorithm to a certain extent:
Due to similarities in atmospheric and aquatic conditions, examining products at n-SNO events minimizes uncertainties in the intercomparison analysis. Therefore, differences in reflectance products, to a large extent, can be attributed to differences in sensor’s absolute radiometric responses. The AC method that produces the most consistent reflectance product can be considered the highest performing method.
Collecting in situ reflectance and synchronizing it with satellite transit time is a difficult thing for us. Lack of in situ validation data has also plagued our research.
Point 4: And did the authors consider the spectral response function of the sensor when evaluating AC performance? MSI is very different from OLI.
Response 4: As mentioned in the previous question, the lack of in situ data plagued our study, as the spectral response function can only be taken into account using in situ reflectance data.
Perhaps using a coefficient to unify the LI data correction of MSI and OLI is a solution, but we found that this correction coefficient is greatly affected by time and space
Analyses limited to moderately eutrophic/turbid Taihu Lake only can reduce uncertainties in spectral response inherent to sensor-to-sensor intercomparisons.
Point 5: Figure 1: Almost all the field stations in the manuscript are at the edge of the land and water. Can the spatial distribution of these points represent the entire Taihu Lake? In addition, the field point close to the land-water boundary is not suitable for the outcome tests due to the influence of the adjacency effect (even if the adjacency correction is used in the AC algorithm). For this reason, the test results of the retrieval model in this manuscript are questionable.
Response 5:
Overall , Taihu Lake can be divided into six sub-regions, consisting of one open area and five bays. The bay areas are relatively closed, and each bay has a varying number of field stations. There is indeed a lack of a sufficient number of measured sites in open areas. However, the hydrological environment in the open area is similar and the time series of our measured data is long enough, we think that the representativeness of the data can be increased to a certain extent.
We have used the following method to eliminate the adjacency effect:
The masking keywords of “maskland”(mask out all land pixels) and “maskbath”( mask out shallow water) in the ACOLITE software were turned on to m
The water reflectance at SWIR band is zero due to the extremely high water absorption, and part of Rrc-swir can be assumed to be the effect of terrestrial aerosols
Point 6: line 166-167: This paper uses a total training sample of 193+101 data to train the machine learning model. Can this amount of data guarantee the stability of the model? I have my doubts about the credibility of the results. Also, the manuscript is missing a lot of details about the retrieval AI model (the description in Chapter 2.5 is too sketchy).
Response 6: Although our research area is only in Taihu Lake, our research objects are four satellites, and the research time spans five years and covers all seasons, so a large amount of data is essential. For machine learning models, this amount of data is appropriate. We found several references to support our idea, and their data volume is similar to ours:
https://doi.org/10.1109/TGRS.2022.3207345
https://doi.org/10.1016/j.rse.2020.111974
We added some details about machine learning models as follows:
SVR can solve the non-linear problems in low dimensional feature space by seeking a linear function in high dimensional feature space. RF establishes multiple decision trees and outputs the average predicted value for each tree. XGBoost predicts the sum of scores in multiple regression trees .
Point 7: Figure 4 shows that the higher the Rrc’ (865) value, the greater the difference between MSI and OLI. In general, the higher the reflectance values in the 865 nm band, the more turbid the water is. Therefore, do the results of Fig. 4 imply that there is a large difference between MSI and OLI results in highly turbid waters?
Response 7: We believe that the slightly higher reflectance of MSI in the red band than OLI is the main reason for the difference in high turbidity, because the red band contributes the most to the model.
The relatively low signal-to-noise ratios of MSI and OLI in the NIR bands may be the reason why the higher the Rrc’(865) value, the greater the difference between MSI and OLI.
Point 8: From Fig. 4(Rrc 865) & Fig. 5, whether the Rrc difference between MSI and OLI in the 865 band will affect the accuracy of the model. Whether different band combinations can be established to verify each other.
Response 8: We have carried out a feature-ranking assessment on the every input features. The NIR band (Rrc(865)) contributed about 10% to the models for turbidity retrievals. After our test, the model with all bands as inputs was the best model. NIR band can significantly improve the performance of turbidity retrievals via the machine learning model.
Point 9: From Fig. 7, As shown in the screenshot, the turbidity of water bodies in the central area of Taihu Lake, MSI and OLI obtained by the model in this paper has a large difference, while the turbidity of the northern waters has little difference. It may be due to problems with the color card display, or it may be due to differences between MSI and OLI when the turbidity is greater than 150 NTU.
Response 9: It’s not the problem of color card display, the range of the color bar was retained consistently for a better comparison.
Point 10: From Fig. 8, the fitting effect of MSI and OLI has a large deviation in the range of 150-200 NTU. Does it mean that the image of the two sensors is different in the application range of NTU?
Response 10: In general, a good correlation is found between turbidity and reflectance at satellite bands located in the red part of the spectrum. Therefore, when modeling with a machine learning model, the red band contributes the most to the model. However, it can be seen from Figure 5(b)that the red band of MSI is slightly higher than that of OLI, so the turbidity derived from MSI will be higher than that derived from OLI, especially in the high turbidity region.
Point 11: line 350-351: The author describes it as "Turbidity was highest in summer and lowest in summer" . This should be a description error. Please check it.
Response 11: Thank you for your careful reading of our manuscript, we have corrected this error.
Point 12: line 217-218: The author will compare the Rrs processed by DSF and C2RCC with the Rrc processed by "RC". Is this comparable and on what basis?
Response 12: The atmospheric path radiance received by a sensor at the TOA can be mainly decomposed into Rayleigh and aerosol scattering. The ultimate goal of any atmospheric correction method is to remove the effects of these two parts. According to the research of Feng et al.:
https://doi.org/10.1016/j.isprsjprs.2018.08.020
The correlation between Rrc and Rrs-swir (SWIR-based AC method SeaDAS) is high. We think Rrs and Rrc are comparable and interchangeable.
Point 13: From Fig. 3, This very well reflects the consistency of MSI and OLI, but this paper does not reflect which AC algorithm is more suitable for the waters of Taihu Lake. Could you please add clarification.
Response 13: I think you are referring to Table 3. We have stated in the second paragraph of Section 3.1 that Rayleigh-SWIR is the most suitable AC method for Taihu Lake.
Point 14: line 217-218: In this paper, a station in the lake is selected as the verification, which I think is insufficient, and multiple areas in the lake should be selected for comparison and verification.
Response 14: We wholeheartedly agree with you, but we felt that putting the other verification points in the main text would take up too much space, so we put the other sites in the appendix.
Point 15: I think the flow chart should be added in this paper to describe the work content more clearly.
Response 15: Thank you for your suggestion, we have added the flow chart in the introduction.
Reviewer 3 Report
Comments
Point 1: Revise English.
Point 2: the introduction should include a mention of the machine learning models used in the methodology. I suggest include other references about the Turbidity retrieval in another continental aquatic ecosystem, for example:
https://www.mdpi.com/2072-4292/13/16/3133
Point 3: Section M&M. subsection 2.1. Study area
I suggest increasing the font size of the map coordinates. Also, it is possible to see:
1. Presence of tributaries (away from their influence).
2. Presence of industrial effluents or urban discharges.
3. Depth.
The topography would be a plus to be added
Added brief description of Morphometric parameters measurement in the lake. example, Long maximum in km, Maximum width, Superficial area, depth, Volume m3, etc
The above allows us to argue the behavior of the variable in the study area.
Point 4. The discussion should be enriched with more refs. and specific section on the limitations of this methodology. In literature there are many works on this lake; you could incorporate more references and compare with other authors the results of the evolution per year of turbidity or its spatial distribution.
I suggest reviewing the following references:
https://www.mdpi.com/2073-4441/12/4/1035
https://link.springer.com/article/10.1007/s11707-016-0612-1
https://www.mdpi.com/2072-4292/15/10/2489
https://www.sciencedirect.com/science/article/pii/S0303243421001641
Comments for author File: Comments.pdf
Author Response
Response to Reviewer 3 Comments
Point 1: Revise English.
Response 1:I’m sorry that I am not a native English speaker, but I have tried my best to improve the English quality of the manuscript.
Point 2: the introduction should include a mention of the machine learning models used in the methodology. I suggest include other references about the Turbidity retrieval in another continental aquatic ecosystem, for example:
https://www.mdpi.com/2072-4292/13/16/3133
Response 2: Thank you for your suggestion. We have added the following to the introduction regarding machine learning:
Due to the good data mining capability, machine learning algorithms provide an alternative to describe the nonlinear relationship between objective and feature variables .
In addition, we taken your suggestion to add Rodríguez-López et al.’s work to the introduction
Point 3: Section M&M. subsection 2.1. Study area
I suggest increasing the font size of the map coordinates. Also, it is possible to see:
- Presence of tributaries (away from their influence).
- Presence of industrial effluents or urban discharges.
- Depth.
The topography would be a plus to be added
Added brief description of Morphometric parameters measurement in the lake. example,
Long maximum in km, Maximum width, Superficial area, depth, Volume m3, etc
The above allows us to argue the behavior of the variable in the study area.
Response 3: Thank you for your suggestion. We have increased the font size of the map coordinates in Figure 1, 7, 9, and 10.
We added the tributaries of Taihu Lake in Figure 1b. However, we had some problems when mapping the urban pollution and the depth of Taihu Lake. Firstly, we can not acquire industrial information because they are confidential. Then, Taihu Lake is a shallow lake, the lake’s average water depth is 1.9 m and its maximum water depth is 3 m. Therefore, the color difference of the depth map of Taihu Lake is not obvious. We found a reference to support our opinion:
https://doi.org/10.1016/j.rse.2021.112572
In the section 2.1, we added the maximum length, average width and shoreline length of Taihu Lake.
Point 4: The discussion should be enriched with more refs. and specific section on the limitations of this methodology. In literature there are many works on this lake; you could incorporate more references and compare with other authors the results of the evolution per year of turbidity or its spatial distribution.
Response 4: Due to the high correlation between turbidity and Secchi disk depth (), we refer to the results of Yin et al.
https://doi.org/10.1016/j.jag.2021.102457
They analyed the temporal and spatial variations in in Taihu Lake from 1984 to 2019. was also low in the open area and high in the five bays.
Reviewer 4 Report
Comments for author File: Comments.pdf
Author Response
Response to Reviewer 4 Comments
Point 1: The introduction part lacks a discussion on the innovation of this manuscript, and further description of its innovation is needed.
Response 1:Thank you for your suggestion, we have added the innovation of this manuscript in the introduction. Specifically, this study is innovative in the following three aspects:
1). testing the performance of three aquatic AC methods to retrieve consistent reflectance products;
2). developing a machine learning model for turbidity retrieval in Taihu Lake;
3). proposing a method for evaluating the consistency of MSI and OLI products in inland water bodies.
Point 2: The author needs to improve the quality of the figures, such as Figure 1, Figure 7, and Figure 9 to Figure 10.
Response 2: Thank you for your suggestion. Firstly, we have increased the font size of the map coordinates and used the same font and size in Figure 2, 8, 10, and 11. Then, we added the tributaries of Taihu Lake in Figure 2b.
Point 3: The label "Taihu Lake" in Figure 1(a) is not rigorous, and the position of Taihu Lake is inconsistent with the label, so reasonable modifications should be made, as shown in Figure 1(b).
Response 3: We realize our laxity, we have moved the label “Taihu Lake” to the center of the lake in Figure 2(a).
Point 4: In lines 84-86, However, the common AC methods are not yet available for optically complex inland waters and few attempts have been made to evaluate the performance of several specialized AC methods. Then, why did the author apply the AC method to the research object of this paper? How does the author explain this?
Response 4: We did not use the surface reflectance products of MSI and OLI because they were originally processed for land applications. Although these specialized AC methods for aquatic system such DSF and C2RCC have not been evaluated the performance in inland waters, they have been widely used in ocean color community. Our goal is not to develop a new AC method but to verify the performance of these well-established methods in inland water bodies.
Point 5: In lines 164-165, if the author excludes a value greater than 300NTU from the
measured data, whether the mean value and the accuracy of the data is affected.
Response 5: Firstly, excluding data with a value greater than 300NTU will hardly affect the mean of all data. In all our available data, only 4 values are greater than 300NTU. Secondly, Machine learning algorithms expect the input data to be normally distributed, so removing less data makes the model more accurate. If we use all the data to model, then the machine learning model will seriously underestimate the high turbidity part.
Point 6: In lines 184-186, the authors stated that We separated all match-ups into two subsets, 70% MSI (n=135) and OLI (n=70) samples were used to train models, and the remaining 30% MSI (n=58) and OLI (n=31) samples were used to test the performance of models. What is the basis for this division?
Response 6: In machine learning, if the proportion of the training set is large, the trained model may be closer to the model trained with the full set, while the test set is small, the evaluation result will be inaccurate, and the variance of the model will be large; if A large proportion of the test set may also lead to a large bias in the trained model, which reduces the fidelity of the evaluation. Therefore, it is common practice to use about 2/3-4/5 of the samples for training. Training set:test set can be 7:3 or 8:2.
Point 7: In lines 200-204, what is the basis for this practice? The authors need to explain its correctness?
Response 7: This creation of intercomparisons is an innovative attempt by us. Firstly, in our on-site measurement and communication with the water quality management department, we have reached a consensus that the water quality within 1km from the sampling point is considered to be the same, so we generated random points with 1 km intervals in Taihu Lake. Secondly, the OLI has a spatial resolution of 30m and MSI has a spatial resolution of 10m, 20m, and 60m, so it is more reasonable to resample the MSI.
We are not sure whether the above should be added to the manuscript
Point 8: In line 223, the authors present that all AC methods perform worst in NIR bands . Can the author explain why this phenomenon occurs?
Response 8: The NIR band was lower in energy, and there was only a very small reflection peak at 800nm, which was more affected by noise; therefore, its relative error was also relatively high.
Point 9: In lines 246-247, Each at MSI and OLI visible bands had significant correlations with turbidity and the difference of gradually reduced at NIR band . How does the author explain this phenomenon?
Response 9: We think that even for very turbid water bodies, the reflectance of water bodies in the near-infrared band is still low, so the difference of gradually reduced at NIR band.
Point 10: In lines 266-269, the authors described that MSI-derived turbidity was highly consistent with OLI-derived turbidity in clean lake water such as the north and east of Taihu Lake. However, MSI-derived turbidity was slightly higher than OLI-derived turbidity in turbid lake water such as the south and west of Taihu Lake . How do the authors explain this result?
Response 10: In general, a good correlation is found between turbidity and reflectance at satellite bands located in the red part of the spectrum. Therefore, when modeling with a machine learning model, the red band contributes the most to the model. However, it can be seen from Figure 5(b)that the red band of MSI is slightly higher than that of OLI, so the turbidity derived from MSI will be higher than that derived from OLI, especially in the high turbidity region.
Round 2
Reviewer 2 Report
Thanks for your response.
Only one comment:
The authors need to add an explanation of why the MSI results are higher than the OLI (Figure 8).
Author Response
Point 1: The authors need to add an explanation of why the MSI results are higher than the OLI (Figure 8).
Response 1:
From the performance of the verification set, the accuracy of the MSI model in the high turbidity area is higher than that of the OLI model (Figure 7). We believe that the model difference is caused the MSI results are higher than the OLI, so we want to study the reasons for this difference in accuracy.
First, we found that R’rc at each band of MSI and OLI had significant correlations with turbidity, suggesting that signals from variations in turbidity are possibly captured by spectral bands of OLI and MSI. We carried out an assessment of the decrease in the accuracy score of the models based on the training dataset to assist in the interpretation of model outcomes and variable contributions to turbidity prediction. We revealed that R’rc(665) and R’rc(704) significantly contributed to the MSI model, while R’rc(655) had considerable contributions to the OLI model. Although a red band may capture turbidity signals in lakes, the red-edge band was absent in OLI.
OLI had different radiometric (e.g., SNRs) and spectral resolutions (e.g., band number, central wavelength, and bandwidth) from MSI. In particular, OLI does not have the bands of 705 and 783 nm. These bands are critical in estimating turbidity in turbid lakes. Sufficient red-NIR bands were critical to reducing the influences of Chl-a on turbidity retrievals in lakes. It should also be noted that the accuracy of the OLI model might possibly decrease with the increase in turbidity due to sparse bands. Although machine-learning models can estimate turbidity from OLI bands, their performance was lower than that of MSI. The insufficient bands from the red to NIR domain of OLI particularly reduced its performance in retrieving turbidity in highly turbid waters. Furthermore, the broad bandwidth of OLI bands resulted in lower performance in retrieving turbidity from lakes compared with that of MSI.