A Kriging Method for the Estimation of ALS Point-Cloud Accuracy without Ground Truth
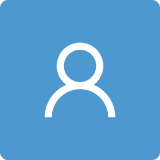
Round 1
Reviewer 1 Report
This paper describes a novel method to estimate and map the accuracy of LiDAR-derived elevation models without ground truthing. I think this could be a very useful approach, and the paper is generally well-written and convincing (though see next point). I have a few minor issues.
1. It would be good to explain in plain language how this works. If I get it correctly, you create a variogram for every point which measures the difference between that reference point and other points at increasing distance. Then you Krige that variogram, which presumably gives you some idea of the expected variation at the zero distance end of the variogram (ie at the reference point). Then you examine those values for all of the points in the defined LULC polygon and report the mean value for 5 m cells. Is that right? For me, who is not an expert in advanced spatial computation, it is the plain version that will convince me if the method is valid.
2. It seems that point density is an important factor in accurately determining error. It would have been good to discuss this issue, especially since your data is at the lower end of point densities (<1/m2), and strangely 3 times less dense than the standard described on line 35.
3. There are 20 acronyms, which makes for difficult reading. I guess this is unavoidable, but the principle should be to avoid using acronyms wherever possible.
Minor points
L16 (abstract). Acronym GT is not defined. I think this is a mistake because there is a CGT defined earlier. Use one of them.
L37. “Canopy maps” could mean quite different things. Do you mean canopy height or canopy cover or both?
L53. It would be good to know what a CGT is. What exactly does it measure and how? I presume it is a differential GPS observation of surface elevation. Also here again you are using CGT and GT almost interchangeably without explaining the difference.
L90. “mean” should be “means”.
L356. I don’t understand what the phrase “the distance at which the model levels out” means.
L375. I didn’t follow how this section reveals a comparison between OK and NSOK. For example, what does figure 6 tell you about the comparison?
Table 1. Can you add the units for each of the measures (eg is range in m?).
Figure 6 needs axis labels.
Figure 7 does not need both the plot and the table.
L398. Can you explain why there are massive holes in figure 8. I presume you made some decision about removing areas more than a certain distance from the GT points.
L401. The use of the term “error” here is confusing because you have already used it for another measure. Would it be better to call this something like the actual error, and the kriging estimate as the predicted error?
Author Response
Please see the attachment.
Author Response File: Author Response.docx
Reviewer 2 Report
The authors present the use of non-stationary ordinary kriging (NSOK) to estimate the accuracy of airborne lidar scanning (ALS) without ground truth. The reviewer would like to inquire about these comments:
1. The format of the manuscript may need to be adjusted such as the keywords (it should not have numbers behind each word), the citation when starting the sentences (should start with the authors’ name, not [number]), and the algorithms (they should not be combined with the figures).
2. Some keywords that are not presented in the abstract should not be important enough to be a keyword so please consider removing them.
3. Why did the authors decide to use one meter for increasing the checking distance? For the prediction of flood damage, the elevation of one meter might be too rough to judge if the designated location will be flooded.
4. What does “the size of the disk” mean on Line 290? Moreover, how did the authors find that 30,000 points is the optimal number for further processes?
5. The letters behind each “Segment” should be displayed as italics to emphasize such parameters.
6. The tables in the manuscript should be revised. The headers should also contain the unit. Significant figures should also be considered. The format of which wording should be bold should be consistent throughout the manuscript.
7. On Line 368, the authors mentioned that there is a distinct boundary between each LULC, and the authors also removed the main road beforehand. These criteria are critical in geographic point clouds because roads and independent trees are very common. Are there any solutions to overcome these limitations?
8. Are there any specific reasons why the authors decided to use 7 cm. as an error interval?
9. For the normal reading sequence, Figures 8d and 8c should be swapped.
10. On Line 411, why Gaussian model was determined to be the most suitable fitting model?
There are very few errors such as on Line 271, it should be "unknown" instead of "unknow".
Author Response
Please see the attachment.
Author Response File: Author Response.pdf
Round 2
Reviewer 2 Report
The reviewer would like to thank the authors for their very precise responses. After reading all of the them, the reviewer only has one small comment left. Although the authors stated that every method and parameter’s value performs well from the evaluation processes such as trial and error (L307-308), the word “well” is very subjective and should be clearly presented how the authors decided that these methods and parameters are considered “well”. At least the 30000 points from the reviewer’s previous comment number 4 should be further explained.
-
Author Response
The reviewer would like to thank the authors for their very precise responses. After reading all of them, the reviewer only has one small comment left. Although the authors stated that every method and parameter’s value perform well from the evaluation processes such as trial and error (L307-308), the word “well” is very subjective and should be clearly presented how the authors decided that these methods and parameters are considered “well”. At least the 30000 points from the reviewer’s previous comment number 4 should be further explained.
Answer:
Ordinary Kriging is computationally intensive and cannot handle all LiDAR point clouds simultaneously. Therefore, we needed to determine the maximum workload it can manage without causing a system crash. Another reason for identifying this threshold was our intention to establish an automated process with a predefined value for the algorithm.
After rigorous testing, we determined that 30,000 points represent the maximum number of elements at which OK can effectively predict elevation at 5-meter intervals. Anything beyond that leads to a system crash. This threshold was established through trial and error and is contingent upon the RAM capacity of the system in use.
It's important to note that this threshold is based on a computer with 64.0 GB RAM and an Intel Core i9-10900X CPU @ 3.70GHz. If someone uses a more robust computer or employs cloud or server resources, the threshold may vary and potentially yield better performance.
L303 to L308 have been updated accordingly.