Optimized Deep Learning Model for Flood Detection Using Satellite Images
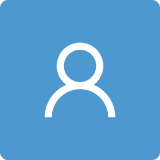
Round 1
Reviewer 1 Report (New Reviewer)
I have gone through the manuscript with interest and commend the authors for the great efforts. In order to improve I have the following minor suggestions
- in the first line of the abstract, there is need to specify the place when rain is increasing given that the trends are spatially variable
- the abstract needs to be re-crafted. It has over-focused on the methods. There is need to clearly state the objectives, include major findings as well as potential applications/benefits of the findings
- I am not sure why there is a lot of text that is highlighted in Red
- in section 3, there is repeated inconsistent use of Fig. and Figure as well as Eq. and Equation.
- in section 4.3.2 there is a table without caption (see just after line 436) which is also not cited in text
- in Figure 3, I recommend that the authors assign different letter labels to the different images instead of having 3 with same label (a), (b), or (c) for easy in-text referencing and following by the readers
- in figure 4, 5 and 6, the legend is blocking view of some of the bar plots. Place it such that all the results can be seen and read without obstruction
Author Response
I have gone through the manuscript with interest and commend the authors for the great efforts. In order to improve I have the following minor suggestions
- in the first line of the abstract, there is need to specify the place when rain is increasing given that the trends are spatially variable
Response: Thank you for your valuable comments. As per the suggestion, we have updated the first of the abstract as “The increasing amount of rain produces a number of issues in Kerala, particularly in urban regions where the drainage system is frequently unable to handle a significant amount of water in such a short period of time.”
- the abstract needs to be re-crafted. It has over-focused on the methods. There is need to clearly state the objectives, include major findings as well as potential applications/benefits of the findings
Response: We appreciate the time and effort that you have dedicated to providing your valuable feedback on my manuscript. According to the comment, we have updated the abstract by providing objectives, major findings and its potential applications.
- I am not sure why there is a lot of text that is highlighted in Red
Response: Sorry for the inconvenience. We have highlighted the portions in Red color which we have modified according to the reviewer comment.
- in section 3, there is repeated inconsistent use of Fig. and Figure as well as Eq. and Equation.
Response: Thank you for pointing this out. As per the suggestion, we have used the consistent terms for figures and equations in the revised manuscript.
- in section 4.3.2 there is a table without caption (see just after line 436) which is also not cited in text.
Response: Thank you for your useful comments. As per the suggestion, we have cited the table along with the caption (Line 452) in section 4.3.2.
- in Figure 3, I recommend that the authors assign different letter labels to the different images instead of having 3 with same label (a), (b), or (c) for easy in-text referencing and following by the readers
Response: Thank you for your important comments. As per the suggestion, we have assigned different letter labels to the different images for figure 3 at section 5.
- in figure 4, 5 and 6, the legend is blocking view of some of the bar plots. Place it such that all the results can be seen and read without obstruction.
Response: Thank you for pointing this out. We agree with this comment. According to the suggestion, we have updated the figure 4, 5 and 6 with proper visualization. Hope the reader can get better understanding from the updated images.
Reviewer 2 Report (New Reviewer)
The authors presented a flood prediction method using fusion of two deep learning method. the topic is intersting, but I couldn't follow the paper as it is. I think the authors should take the time for writting and organization of this. I recommend to reject and resubmit, because this is not similar to a standard paper.
My comment is "rejected and resubmitted". New submissions should have the following standard to be ready for review:
1- The paper should have clear research questions, and authors should explain the novelties.
2- The literature review should be summarized in each item.
3- The authors mentioned "the B=5, is the number of bands, is this correct for landsat 8? If they used B=5, why the formula of Eq. 8 GVI.
4- In the spectral indices, they can input all of the formula on a table not as this form. Please don't use the number of bands, please use the spectral name for them, as well.
5- As I see the flowchart, I am wondering if I can see a magenta ellipsoid in deep learning, what is this? the authors know what do you want to do?
6- the metrics not introduced in the manuscript.
7- Dataset description is not clearly explained in the paper.
8- The experimental setup is not provided by the authors. The loss and val loss for each of the deep learning methods should be clearly identified.
9- As I see the experimental results are only provided by the quantitative measurements and need to present the flood predictions.
10- The discussion should have a clear comparison with other studies.
11- The authors just should see the presented sdu code, some equations do not have input.
12- I see a lot of formulas about the different parts, is it necessary to take a lot of formulas that have already been published by the other researchers. Which one is for you?
Author Response
The authors presented a flood prediction method using fusion of two deep learning method. the topic is intersting, but I couldn't follow the paper as it is. I think the authors should take the time for writting and organization of this. I recommend to reject and resubmit, because this is not similar to a standard paper.
My comment is "rejected and resubmitted". New submissions should have the following standard to be ready for review:
- The paper should have clear research questions, and authors should explain the novelties.
Answer: We appreciate the time and effort that you have dedicated to providing your valuable feedback on my manuscript. According to the comment, we have provided research questions and its novelties.
- How can synthetic data be used for the construction of deep learning algorithms?
Extreme precipitation events that induce flooding are rare occurrences. Therefore, there are not enough recorded historic events for the training, testing and validation of DL algorithms. Synthetic data can be used to bridge this gap and provide enough data for training, testing and validation.
- What type of parameter configurations are best performing?
With DL algorithms there are many ‘higher order’ parameters that need to be determined. These parameters are not trained by the DL algorithms and have no physical attributes and cannot be empirically determined. Therefore, many combinations of parameters need to be tested to determine the optimal configuration for the specific problem.
- What is the performance of the algorithms and is this persistent when validated on historic data?
Final performance of the algorithms will determine applicability for the prediction of pluvial flooding in an urban environment. The DL algorithms are also tested on available historic data. This can give an indication if the DL algorithms trained on synthetic data, could be applied in a real-world environment.
- The literature review should be summarized in each item.
Response: Thank you for your useful comments. According to the comment, we have summarized the literature review at the end of section 2.
The following information’s are updated at section 2,
“According to the aforementioned research, the information that was retrieved from the data typically exceeds the limitations of the measurements, even if the satellite data has a high degree of uncertainty. However, the data has not been properly analysed in order to determine how various remote sensing techniques and analysis were used to locate the flooded area. As a result, till now, so many approaches have existed for evaluating remote sensing-based systems. Unsupervised deep learning algorithms are more reliable because they are faster, use less training data, take less time to run and also provide superior computing efficiency. Therefore, a hybrid deep learning model named as DHMFP-CHHSSO is proposed for flood detection which provides greater performance with a lower data count and processing time for improved fast flood mapping. The suggested technique helps the other cities at risk of urban flooding by employing satellite data to reduce flood risk of transportation design and urban infrastructure development in addition to for flood detection”.
- The authors mentioned "the B=5, is the number of bands, is this correct for landsat 8? If they used B=5, why the formula of Eq. 8 GVI.
Response: Thank you for pointing this out. According to the comment, we have updated the spectral bands at section 3.2.
- In the spectral indices, they can input all of the formula on a table not as this form. Please don't use the number of bands, please use the spectral name for them, as well.
Response: Thank you for your important comments. According to the comment, we have provided the spectral name of the bands presents in this research at section 3.2.
The following information’s are updated at section 3.2,
“13 spectral bands: Coastal aerosol, Blue, Green, Red, Vegetation Red Edge, Vegetation Red Edge, Vegetation Red Edge, NIR, Vegetation Red Edge, Water vapour, SWIR-curris, SWIR, SWIR”.
- As I see the flowchart, I am wondering if I can see a magenta ellipsoid in deep learning, what is this? the authors know what do you want to do?
Response: Thank you for the valuable suggestion. You have raised an important point here. As per the comment, we have updated the figure 1 with proper visualization. Hope the reader can get better understanding from the updated image.
- the metrics not introduced in the manuscript.
Response: Thank you for your valuable comments. According to the comment, we have provided the metrics used for the calculation at section 5.
- Dataset description is not clearly explained in the paper.
Response: Thank you for your useful comments. According to the comment, we have updated the dataset description with proper information at section 3.1.
- The experimental setup is not provided by the authors. The loss and val loss for each of the deep learning methods should be clearly identified.
Response: Thank you for the valuable suggestion. You have raised an important point here. As per the comment, we have provided the experimental setup for this research at section 5. Further, we have provided loss and val loss for proposed method alone at section 5.8.
- As I see the experimental results are only provided by the quantitative measurements and need to present the flood predictions.
Response: Thank you for this suggestion. It would have been interesting to explore this aspect. As per the reviewer’s comment, we have presented the results of flood predictions at section 5.4.
- The discussion should have a clear comparison with other studies.
Response: We are grateful to the reviewers for their insightful comments on my paper. As per the comment, we have updated the discussion by providing a clear comparison with other studies at section 5.9.
The following information’s are updated at discussion,
“In this study, a novel Deep Hybrid Model for Flood Prediction (DHMFP) based CHHSSO- is suggested for flood prediction. The proposed CHHSSO based on DHMFP is compared to a number of existing models, including DBN, RNN, LSTM, SVM, Bi-GRU, SSOA, HHO, COOT, AOA, CD, and FCNN. The improved K-means has attained dice-score (0.863), Jaccard coefficient (0.889), and segmented accuracy (0.894) for the segmentation study. The final extracted feature set is used to perform hybrid classification using CNN and Deep ResNet classifiers to get the final feature set. The CHHSSO algorithm then adjusts the weight of CNN and Deep ResNet. For that analysis, the proposed DHMFP receives the following values from the results: sensitivity = 93.48%, specificity = 98.29%, accuracy = 94.98%, FNR = 0.02%, and FPR = 0.02%. The effectiveness of DHMFP flood detection in satellite images is investigated using a statistical analysis which is elaborated at Table II. Those analysis clearly demonstrates that the suggested DHMFP maintains the error rate of 0.034 despite the values of DBN (0.113), RNN (0.132), LSTM (0.119), SVM (0.124), Bi-GRU (0.144), SSOA (0.170), HHO (0.131), COOT (0.153), AOA (0.123), CD (0.163), and FCNN (0.226). Furthermore, for the ablation study, the proposed DHMFP-CHHSSO displays better performances in terms of sensitivity (0.932), specificity (0.977), accuracy (0.952), False Negative Rate (0.0858), and False Positive Rate (0.036) respectively. Hence, from the overall analysis, it is determined that proposed DHMFP is sufficient for flood detection in satellite images when compared with other existing models”.
- The authors just should see the presented sdu code, some equations do not have input.
Response: Thank you for pointing this out. We agree with this comment. According to the suggestion, we have updated the pseudocode with proper input data.
- I see a lot of formulas about the different parts, is it necessary to take a lot of formulas that have already been published by the other researchers. Which one is for you?
Response: Thank you for the valuable suggestion. You have raised an important point here. We have highlighted the new (novel) concept of this research at section 4. The citations which we have used in this research will be useful for the readers to get better understanding about the equations and terms existed for the algorithms.
Reviewer 3 Report (New Reviewer)
1. Lines 49-50, it is the most important factor!!
2. 2. Literature Review, rearrange it to a chronological order.
3. Line 229, what is your input data format? Is it just a JPG image format from the web page or you got both of the original imageries from Landsat 8 and Sentinel 2 accordingly as inputs?
4. Line 470, fig. should be Fig.
5. Line 493, like Line 470.
6. Line 505, like lines 470 and 493.
7. Line 521, table I should be Table 1.
8. Line 534, like Table 1.
9. Line 542, like Table 1 and 2.
10. Line 553, fig. 7 should be Fig.7.
11. Kindly read it throughly because I saw many missed typos.
Comments for author File: Comments.pdf
Looks good, but it needs to be rearranged a bit with many missed typos.
Author Response
- Lines 49-50, it is the most important factor!!
Response: Thank you for your important comments. Yes, that is the most important, based on that we have analyzed the literature and provided a solution for it accordingly.
- Literature Review, rearrange it to a chronological order.
Response: Thank you for your useful comments. As per the comment, we have arranged the literature review in a chronological order.
- Line 229, what is your input data format? Is it just a JPG image format from the web page or you got both of the original imageries from Landsat 8 and Sentinel 2 accordingly as inputs?
Response: Thank you for pointing this out. According to the figure 1 (Line 232), we have provided the input data as an image which is available in the dataset link. According to that, we have processed our research work.
- Line 470, fig. should be Fig.
Response: Thank you for pointing this out. As per the comment, we have updated the term as Fig. in the updated manuscript.
- Line 493, like Line 470.
Response: Thank you for pointing this out. As per the comment, we have updated the line 539 according to 516 in the updated manuscript.
- Line 505, like lines 470 and 493.
Response: Thank you for pointing this out. As per the comment, we have updated the line 551 according to 516 in the updated manuscript.
- Line 521, table I should be Table 1.
Response: Thank you for pointing this out. As per the comment, we have updated the term as Table 1 in the updated manuscript.
- Line 534, like Table 1.
Response: Thank you for pointing this out. As per the comment, we have updated the term as Table 2 in the updated manuscript.
- Line 542, like Table 1 and 2.
Response: Thank you for pointing this out. As per the comment, we have updated the term as Table 3 in the updated manuscript.
- Line 553, fig. 7 should be Fig.7.
Response: Thank you for pointing this out. As per the comment, we have updated the term as Fig. 7 in the updated manuscript.
- Kindly read it thoroughly because I saw many missed typos.
Response: Thank you for this suggestion. As per the comment, we have eliminated all the typo errors in the updated manuscript.
Reviewer 4 Report (New Reviewer)
In this paper, authors explored the Flood Detection with deep learning methods and Satellite Images. It is informative. However, I have some specific comment as followed:
1) Abstract. The structure of the abstract should be adjusted. Normally, abstract should contain three organized parts, including 1) background, significance or weakness, 2) research contents and method, and 3) result. However, the second part is too lengthy and not concise. While, the third part should be strengthened.
2) This is not a review article, the literature summary appears abrupt as a single paragraph, and the length is too long. I suggest focusing on analyzing the shortcomings of research in the literature and using this as a basis to introduce the research focus and theme of this article
3) All the pictures in the article are not clear, it is suggested to increase the clarity. The annotation font for the horizontal and vertical coordinates in the figures are too small.
4) For the result section, the analysis is too simple and not deep enough.I suggest you should make a thorough analysis of the results.
5)The article uses many algorithms and models, but there are too many conventional concepts introduced to these methods. The reason for using these models are unclear about their applicability and uniqueness to the research topic
Author Response
In this paper, authors explored the Flood Detection with deep learning methods and Satellite Images. It is informative. However, I have some specific comment as followed:
1) Abstract. The structure of the abstract should be adjusted. Normally, abstract should contain three organized parts, including 1) background, significance or weakness, 2) research contents and method, and 3) result. However, the second part is too lengthy and not concise. While, the third part should be strengthened.
Response: We are grateful to the reviewers for their insightful comments on my paper. As per the comment, we have updated the abstract with specified structure.
The following information’s are updated at abstract part,
Background: The increasing amount of rain produces a number of issues in Kerala, particularly in urban regions where the drainage system is frequently unable to handle a significant amount of water in such a short period of time. Meanwhile, standard flood detection results are inaccurate for complex phenomena and cannot handle enormous quantities of data. In order to overcome those drawbacks and enhance the outcomes of conventional flood detection models, deep learning techniques are extensively used in flood control.
Research method: Therefore, a novel Deep Hybrid Model for Flood Prediction (DHMFP) with Combined Harris Hawks Shuffled Shepherd Optimization (CHHSSO) based training algorithm is introduced for flood prediction. Initially, the input satellite image is preprocessed using the median filtering method. Then the preprocessed image is segmented using the Cubic chaotic map weighted based K-means clustering algorithm. After that, based on the segmented image, features like Difference Vegetation Index (DVI), Normalized Difference Vegetation Index (NDVI), Modified Transformed Vegetation Index (MTVI), Green Vegetation Index (GVI), and Soil Adjusted Vegetation Index (SAVI) are extracted. The features are subjected to hybrid model for predicting floods based on the extracted feature set. The hybrid model includes models like CNN (Convolutional Neural Network) and Deep ResNet classifier. Also, to enhance the prediction performance, the CNN and Deep ResNet models are fine-tuned by selecting the optimal weights by Combined Harris Hawks Shuffled Shepherd Optimization (CHHSSO) algorithm during the training process.
Result: This hybrid approach reduces the number of errors while increasing the effectiveness of deep neural networks with more neural layers. From the result analysis, it clearly shows that the proposed work has obtained the sensitivity (93.48%), specificity (98.29%), accuracy (94.98%), False Negative Rate (0.02%), and False Positive Rate (0.02%) on analysis. Furthermore, for the ablation study, the proposed DHMFP-CHHSSO displays better performances in terms of sensitivity (0.932), specificity (0.977), accuracy (0.952), False Negative Rate (0.0858), and False Positive Rate (0.036) respectively.
2) This is not a review article, the literature summary appears abrupt as a single paragraph, and the length is too long. I suggest focusing on analyzing the shortcomings of research in the literature and using this as a basis to introduce the research focus and theme of this article.
Response: Thank you for this suggestion. It would have been interesting to explore this aspect. As per the comment, we have updated the literature review with proper information. Further, we have coordinated the shortcomings of research to introduce the research focus and theme of this article at the end of section 2.
3) All the pictures in the article are not clear, it is suggested to increase the clarity. The annotation font for the horizontal and vertical coordinates in the figures are too small.
Response: Thank you for the valuable suggestion. You have raised an important point here. As per the reviewer’s comment, we have updated all the figures with high resolution quality. Further, we have improved the annotation font for the horizontal and vertical coordinates in the figures.
4) For the result section, the analysis is too simple and not deep enough. I suggest you should make a thorough analysis of the results.
Response: We appreciate the time and effort that you have dedicated to providing your valuable feedback on my manuscript. According to the comment, we have improved the analysis section by providing training and validation accuracy/loss at section 5.8. Further, we have provided thorough analysis of the results in the discussion part (section 5.9).
5)The article uses many algorithms and models, but there are too many conventional concepts introduced to these methods. The reason for using these models is unclear about their applicability and uniqueness to the research topic
Response: Thank you for the valuable suggestion. You have raised an important point here. We have highlighted the new (novel) concept of this research at section 4. The citations which we have used in this research will be useful for the readers to get better understanding about the equations and terms existed for the algorithms.
Cited References:
[26] Yuqin, S.; Liu, J. An improved adaptive weighted median filter algorithm, IOP Conf. Series: Journal of Physics: Conf. Series, 2019, 042107 doi:10.1088/1742-6596/1187/4/042107
[27] Saptarshi, C.; Paul, D.; Das, S.; Xu, J. Entropy Weighted Power k-Means Clustering, Proceedings of the 23rdInternational Conference on Artificial Intelligence and Statistics (AISTATS) 2020, Palermo, Italy. PMLR: 2020, 108.
[28] Hui, L.; Wang, X.; Fei, Z.; Qiu, M.; The Effects of Using Chaotic Map on Improving the Performance of Multiobjective Evolutionary Algorithms, Hindawi Publishing Corporation Mathematical Problems in Engineering, 2014. http://dx.doi.org/10.1155/2014/924652
[29] https://www.l3harrisgeospatial.com/docs/broadbandgreenness.html
[30] Driss, H.; Millera, J.R.; Pattey, E.; Zarco-Tejadad, P.J.; Ian B.S.; Hyperspectral vegetation indices and novel algorithms for predicting green LAI of crop canopies: Modeling and validation in the context of precision agriculture, Elsevier Inc. doi: 10.1016/j.rse.2003.12.013.
[31] Martinez JC, De Swaef T, Borra-Serrano I, Lootens P, Barrero O, Fernandez-Gallego JA. Comparative leaf area index estimation using multispectral and RGB images from a UAV platform. InAutonomous Air and Ground Sensing Systems for Agricultural Optimization and Phenotyping VIII 2023 Jun 13 (Vol. 12539, pp. 56-67). SPIE.
[32] Zijie J.W.; Turko, R.; Shaikh, O.; Park, H.; Das, N.; Hohman, F.; Kahng, M.; Horng D. CNN EXPLAINER: Learning Convolutional Neural Networks with Interactive Visualization, arXiv:2004.15004v3 [cs.HC] 2020.
[33] Ramayanti S, Nur AS, Syifa M, Panahi M, Achmad AR, Park S, Lee CW. Performance comparison of two deep learning models for flood susceptibility map in Beira area, Mozambique. The Egyptian Journal of Remote Sensing and Space Science. 2022 Dec 1;25(4):1025-36.
[34] Aiden, N.; He, Z.; Wollersheim, D. Pulmonary nodule classification with deep residual networks, Int J Comput Assist Radiol Surg. 2017, 10, 1799-1808. doi: 10.1007/s11548-017-1605-6.
[35] Liu J, Liu K, Wang M. A Residual Neural Network Integrated with a Hydrological Model for Global Flood Susceptibility Mapping Based on Remote Sensing Datasets. Remote Sensing. 2023 May 6;15(9):2447.
[36] Jackson J, Yussif SB, Patamia RA, Sarpong K, Qin Z. Flood or Non-Flooded: A Comparative Study of State-of-the-Art Models for Flood Image Classification Using the FloodNet Dataset with Uncertainty Offset Analysis. Water. 2023 Feb 24;15(5):875
Round 2
Reviewer 2 Report (New Reviewer)
As I see the paper improved but need another revision to improve.
1- Please bring the citation for the k means description by citing to following references.
https://doi.org/10.1007/s12517-017-3072-3
https://doi.org/10.1016/j.ins.2010.10.016
2- Please bring all of the Vegetation indices and other indices in a Table not in separate subsections. I already told this matter.
3- As I see the discussion is not complete, please compare the proposed method with other methods.
4- Please proofread the paper and check the similarity.
Author Response
Reviewer 2:
As I see the paper improved but need another revision to improve.
1- Please bring the citation for the k means description by citing to following references.
https://doi.org/10.1007/s12517-017-3072-3
https://doi.org/10.1016/j.ins.2010.10.016
Response: We appreciate the time and effort that you have dedicated to providing your valuable feedback on my manuscript. According to the reviewer comment, we have updated the citation for the k means description by citing the references ([28], [29]) in the updated manuscript.
Cited References:
[28] Moghimi A, Khazai S, Mohammadzadeh A. An improved fast level set method initialized with a combination of k-means clustering and Otsu thresholding for unsupervised change detection from SAR images. Arabian Journal of Geosciences. 2017 Jul; 10:1-8.
[29] Ghosh A, Mishra NS, Ghosh S. Fuzzy clustering algorithms for unsupervised change detection in remote sensing images. Information Sciences. 2011 Feb 15;181(4):699-715.
2- Please bring all of the Vegetation indices and other indices in a Table not in separate subsections. I already told this matter.
Response: We are grateful to the reviewers for their insightful comments on my paper. As per the reviewer suggestion, we have updated all of the Vegetation indices and other indices in a Table format (Table 1) at section 3.4.
3- As I see the discussion is not complete, please compare the proposed method with other methods.
Answer: Thank you for this valuable suggestion. It would have been interesting to explore this aspect. As per the reviewer’s comment, we have updated the discussion part with existing method ([22]) results at section 5.9 in the revised manuscript.
Cited Reference:
[22] Drakonakis GI, Tsagkatakis G, Fotiadou K, Tsakalides P. OmbriaNet—supervised flood mapping via convolutional neural networks using multitemporal sentinel-1 and sentinel-2 data fusion. IEEE Journal of Selected Topics in Applied Earth Observations and Remote Sensing. 2022 Mar 1; 15:2341-56.
4- Please proofread the paper and check the similarity.
Response: Thank you for your valuable time and observation. You have raised an important point here. As per the reviewer’s comment, we have done a thorough proofreading to improve the quality of the manuscript. Further, we have checked the similarity of this manuscript which shows 5%.
Round 3
Reviewer 2 Report (New Reviewer)
The authors well addressed my concerns.
This manuscript is a resubmission of an earlier submission. The following is a list of the peer review reports and author responses from that submission.
Round 1
Reviewer 1 Report
This research is useful for flood management. However, there are several issues that need to be corrected before being considered for publication, with the following issues:
1) The title is inconsistent with the research presented. The authors should change the term flood prediction to flood detection instead.
2) In Literature Review, only 8 main research papers were presented, which is too few. The authors should further review the literature and research on flood detection.
3) In the literature review content, there is still no presentation of the results of each research in terms of validity. including the limitations of each previous study.
4) The author must be careful about the wrong words because it will affect the quality of the research presented, such as flood, but in the research, it is written as food.
5) Topic 2.1 Review should not be a separate topic but should be included in the literature review section.
6) The equations are used without any changes. The author should present it in the literature review section instead of the methodology section, as this will confuse the reader with lengthy processes such as the vegetation index equation.
7) Figures 1 and 2 need to improve the image resolution. and adjust the font size in the image
8) Figure 1 is not mentioned and explained in the research. The author should elaborate because this is a research framework for the proposed research.
9) Fig. 3 presentation must improve the resolution and image size to reflect the difference in the presentation of the results using each algorithm.
10) Lack of presentation of final output e.g. flooded map
11) The accuracy of the detection image by learning percentage is not significant. It should be presented using testing data and validation data would be more appropriate and reliable.
12) How does this research examine and prevent overfitting problems of image detection or classification?
13) Lack of discussions compared to previous research in terms of validity and strengths of the research.
14) In the summary, the author does not need to describe the process. but should summarize the strengths and limitations including future research
15) The number of research papers cited is too small. Research papers should present at least 40 references.