TPH-YOLOv5++: Boosting Object Detection on Drone-Captured Scenarios with Cross-Layer Asymmetric Transformer
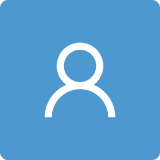
Round 1
Reviewer 1 Report
The author carried out a target detection experiment on UAV images based on the deep learning method. From the experimental results for a specific data set, the performance of the proposed model is acceptable. But from the perspective of writing, there are still some problems to be solved,
1) The paper uses a lot of abbreviations, which may sometimes confuse readers. For example, in Line 4, TPH appears before the explanation of Line 6. Line 472, the cosine lr schedule should be explained. It is recommended that the author check the full text again to make the paper more standardized.
2) The title of this paper is the THP++ model, but the paper introduces a large number of THP models, which makes people feel puzzled. If the two models are good at handling different detection tasks, then the roles of the two models should be equal. If THP is only a module or part of THP++, then THP should not be emphasized too much. In other words, I think that the author did not handle the relationship between the two models well, and there is a certain inconsistency between the title and the content of the paper. The current version is not conducive to the promotion of the method, but also affects the understanding of readers. It is recommended to refer to the papers published in RS journals, such as the following part but not limited to this.
https://doi.org/10.3390/rs15020371
https://doi.org/10.3390/rs14133109
https://doi.org/10.3390/rs15020539
3) Regarding Figures, the position of the picture in the paper is too random and often placed in the middle of a paragraph of text. It is recommended to adjust the figures uniformly after the first cited paragraph. In addition, the font of some text in the figures is too small, as shown in Figure 4, it is recommended to increase the font in the picture, which only needs to be 1-2 sizes smaller than the text.
4) The form of Tables is also too casual. It is also recommended to refer to the requirements of recently published papers and journals and try to unify the form of Tables in the full text.
5) The conclusion section of the paper suggests stressing the main work and highlights. The current way of writing is somewhat inverted. For example, the author first introduces the proposed model, followed by the most important improvements, and what are the main achievements of the latter model.
Author Response
Please see the attachment.
Author Response File: Author Response.pdf
Reviewer 2 Report
In this paper, the authors propose a new method for object detection on drone-captured images based on YOLOv5. The method called TPH-YOLOv5++ uses two Transformer Prediction Heads (TPH), introduced in the authors' previous method TPH-YOLOv5, but also uses a new Cross-layer Asymmetric Transformer (CA-Trans) to improve small and densely packed objects detection.
The paper is well written and well organized, but there are some shortcomings that should be corrected:
In Abstract there are too much sentences written in the first person.
Introduction, line 23 – words „disaster relief“ are duplicated
Introduction, line 97 – authors mentioned that TPH-YOLOv5 won 5th place in VisDrone2021 DET challenge, while on other places in text (e.g. abstract) they declared 4the place in this challenge
Section 2 - In Figure 2 upsampling operation is not presented correctly
Section 2 - In Figure 3, upsampling and downsampling operations should be marked more clearly
Section 2 /Section 3 - For a better understanding of the TPH-YOLOv5++ architecture, it would be useful to add labels for the outputs from individual function blocks (f1, f2''...and others) to figures 3 and 6.
Section 3 - Equation 7 does not give a solution for f2'' as given in line 399, but for p2.
Section 3 – Text in line 419 should be changed to “… is (a, b) in which a ≥ 2 and b ≥ 2, then we need pad zeros…“
Section 4, subsection 4.1.1 – Authors should provide information about image resolution for datasets used
Section 4, subsection 4.1.3 - Authors should define evaluation metrics AP50, AP75, AR1, AR10, AR100 and AR500
Section 4 - What is TPH-YOLOv5+ms-testing in Table 7?
Section 4 - The dataset for which the data is presented should be added in the caption of Table 8
Author Response
Please see the attachment.
Author Response File: Author Response.pdf
Reviewer 3 Report
Here are some suggested edits to make the feedback more polished:
1. While the proposed method uses existing popular modules from the literature in a specific application, it would be beneficial for the authors to provide a more detailed explanation of how these modules were specifically chosen to address the unique challenges of drone detection. This would help to demonstrate the insights of the chosen modules and highlight the novelty of the proposed approach.
2. While the paper highlights the advantages of the proposed approach, such as improved detection speed and performance on drone-captured datasets, it would be helpful for the authors to provide a more detailed analysis of the potential drawbacks and limitations of the proposed approach. Such analysis would help to better understand the practical implications of the proposed approach and its potential limitations in real-world scenarios.
3. It would be beneficial for the authors to include some important recent works in vision transformers, such as [1][2][3], in their literature review. Including these works would help to provide a more comprehensive overview of recent advances in vision transformers and demonstrate how the proposed approach fits into the current state of the art.
[1] Xu, Q., Hu, H., Liu, C., & Wang, X. (2022). V2X-ViT: Vehicle-to-everything cooperative perception with vision transformer. In European Conference on Computer Vision (ECCV).
[2] Li, Y., Zhou, J., Wang, J., Zhang, S., & Liu, Y. (2022). CoBEVT: Cooperative bird's eye view semantic segmentation with sparse transformers. In Conference on Robot Learning (CoRL).
[3] Liu, X., Chen, K., Chen, S., Zhang, Z., & Wang, J. (2022). Maxvit: Multi-axis vision transformer. In European Conference on Computer Vision (ECCV).
Author Response
Please see the attachment.
Author Response File: Author Response.pdf