Integrating Active and Passive Remote Sensing Data for Mapping Soil Salinity Using Machine Learning and Feature Selection Approaches in Arid Regions
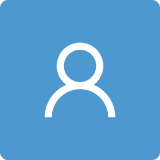
Round 1
Reviewer 1 Report (New Reviewer)
The authors investigated the Integrating Active and Passive Remote Sensing Data for Mapping Soil Salinity using Machine Learning and Feature Selection Approaches in Arid region. Though the manuscript is well written but, in some cases, the methodological options are not well justified. Considering my observations as follows, I suggest that the paper undergo major revision before considering it for publication.
Comments
1. The necessity and innovation of the article should be presented in the introduction. Also, please add the recent literature related to soil salinity prediction using Machine Learning approaches
2. There is no clear explanation of the LR, RF, SVR and BPNN models. How were these two models calculated? What are the model parameters? How were these models calibrated? Which software was used for the calculation?
3. Which methods were applied to evaluate machine learning models?
4. What was the proportion of training, testing, and validation datasets for machine learning models?
5. The conclusion can be improved by highlighting the innovation content of the paper, future research directions, and recommendations for policy formulation.
6. Line 218: “15 spectral indices were calculated…..” please don’t start a line numeric number. You can write “Fifteen spectral indices were calculated…..”
7. In the Table 2 there is lot abbreviation such as WDVI, TNDVI, SAVI etc. What is the meaning of those abbreviation. The abbreviation that appears first write the full meaning.
8. Line 118-123: Please omit or remove these lines for the from the introduction section. Or, you can place it in the methodology section.
9. I would strongly recommend to check the English grammar with a native English speaker.
Author Response
1. The necessity and innovation of the article should be presented in the introduction. Also, please add the recent literature related to soil salinity prediction using Machine Learning approaches
- We agreed with the reviewer; three new sections were added to the introduction. Lines 106-129, 130-139 and 140-149.
- The added references were published between the years 2021 and 2023.
2. There is no clear explanation of the LR, RF, SVR and BPNN models. How were these two models calculated? What are the model parameters? How were these models calibrated? Which software was used for the calculation?
- We added more information on the used ML models in lines 266 - 269 and 337 - 344
3. Which methods were applied to evaluate machine learning models?
- We added information in lines 160-161 in addition to section 3.1. Modeling assessment and Figure 4
4. What was the proportion of training, testing, and validation datasets for machine learning models?
-
The 3K-fold cross-validation method was used to training, testing, and validation datasets.
5. The conclusion can be improved by highlighting the innovation content of the paper, future research directions, and recommendations for policy formulation.
- New sections were added in the Conclusion; Lines 504-514 and 551-568
6. Line 218: “15 spectral indices were calculated…..” please don’t start a line numeric number. You can write “Fifteen spectral indices were calculated…..”
- Adjusted according to the reviewer's comment
7. In the Table 2 there is lot abbreviation such as WDVI, TNDVI, SAVI etc. What is the meaning of those abbreviation. The abbreviation that appears first write the full meaning.
- a new paragraph was added; lines 256-264
8. Line 118-123: Please omit or remove these lines for the from the introduction section. Or, you can place it in the methodology section.
-
The paragraph has been deleted.
9. I would strongly recommend to check the English grammar with a native English speaker.
- the English of this manuscript was checked by an English native speaker
Reviewer 2 Report (New Reviewer)
1.Supplement the background knowledge of soil salinity inversion by penetrating the surface with sar and optical data.
2.It should not only discuss the accuracy of the model, but also supplement the description and analysis of soil salinity after inversion.
3.The accuracy comparison of the same type of research should be supplemented in the paper discussion.
4.Conclusion writing needs to be optimized.
Author Response
1. Supplement the background knowledge of soil salinity inversion by penetrating the surface with sar and optical data.
-
Sentinel_1 C-band data were used in this study which has low penetration characteristics
2.It should not only discuss the accuracy of the model, but also supplement the description and analysis of soil salinity after inversion.
- New paragraph was added in the Discussion section lines:496-506'
3.The accuracy comparison of the same type of research should be supplemented in the paper discussion.
- We relocated this suggestion to the introduction section; lines 106-149.
4. Conclusion writing needs to be optimized.
-
The conclusion has been edited as suggested
Reviewer 3 Report (New Reviewer)
Advantages of using remote sensing technology for mapping soil salinity include saving time, wide coverage, are faster than ground methods, and facilitate long term monitoring. This study aims to improve soil salinity prediction by using a combined data collection consisting of Sentinel-1 C radar data and Sen-tinel-2 optical data acquired simultaneously via integrating radar and optical sensor variables. This is an interesting study, which can get various attentions for international academic community. Previously, these remotely sensed data have been successfully used for monitoring and mapping soil salinity for decades with mixed results. Many researchers have used different techniques to monitor and map soil salinity using remote sensing data. The new approach proposed in this study focuses on feature selection strategies and regression learning. This is an great method and interesting study. However, I believe some key details about method and results are missing in current version. Regarding the current version, I recommend a moderate revision before possible publication in Remote Sensing. Here are my major concerns:
1. In this study, the authors selected 47 selected variables as input for the new approach. However, did the authors do any statistical investigation on the correlation between the 47 variables? For example, variable A and variable B, both comes from the 47 variables, but are strong correlated. Under this condition, It is not necessary to include all the two variables in the input variable set.
2. Multispectral images has limited diagnostic capability. For example, direct mapping of soil salinity with multispectral imagery had major limitations that arise, especially where there are no salt features on the soil surface and where saline soils are dominated by halophyte plants. Additionally, satellite sensors may get confusing reflectance, when non-saline soils are confused with bare, extremely saline areas. In the case of Landsat imagery, the spectral resolution of Landsat was insufficient due to the difference between the spectra of saline land and waterlogged land not being sufficient to allow spectral separation, as well as the variable spectral response of saline soil. Regarding the SAR and Sentinel-2 multispectral images used in this study, any similar problems were observed in the study?
Author Response
1- In this study, the authors selected 47 selected variables as input for the new approach. However, did the authors do any statistical investigation on the correlation between the 47 variables? For example, variable A and variable B, both comes from the 47 variables, but are strong correlated. Under this condition, It is not necessary to include all the two variables in the input variable set.
-
We investigated the correlation between each variable and the salinity values using filter methods (Pearson coefficient, Spearman coefficient, entropy, and F-tests), finally, we chose the most correlated variables.
2. Multispectral images has limited diagnostic capability. For example, direct mapping of soil salinity with multispectral imagery had major limitations that arise, especially where there are no salt features on the soil surface and where saline soils are dominated by halophyte plants. Additionally, satellite sensors may get confusing reflectance, when non-saline soils are confused with bare, extremely saline areas. In the case of Landsat imagery, the spectral resolution of Landsat was insufficient due to the difference between the spectra of saline land and waterlogged land not being sufficient to allow spectral separation, as well as the variable spectral response of saline soil. Regarding the SAR and Sentinel-2 multispectral images used in this study, any similar problems were observed in the study?
- We agree with the reviewer, lines 81-89 clarify this matter in addition to the general discussions in this research
Reviewer 4 Report (New Reviewer)
This paper is very well presented and coherent. Great work!
Author Response
Thank you for your kind words and positive feedback! It's great to hear that the paper was well-presented and coherent. We appreciate your comments and are thrilled to know that you found the paper to be of high quality.
Round 2
Reviewer 1 Report (New Reviewer)
I have been brought in a second time to review this manuscript. The authors answered all my previous comments. At its present form, the article has improved significantly. Therefore, I recommend to publish this article.
Reviewer 2 Report (New Reviewer)
Accept in present form
Reviewer 3 Report (New Reviewer)
Dear editor,
I've checked the revised version. The major concerns addressed in last round review have been fixed. The reviesed version is fine for publication in RS.
This manuscript is a resubmission of an earlier submission. The following is a list of the peer review reports and author responses from that submission.
Round 1
Reviewer 1 Report
This study constructed a robust and flexible spatial prediction model using a combination of optical and SAR satellite data to assess and map soil salinity in the northern Nile Delta of Egypt, as an example of the arid ecosystem. Overall, the manuscript covers an interesting topic, and is very well written. But there are still some questions:
1. What is the reason for taking 100 samples from 89 fields as ML training and testing samples? Are these samples sufficient to reflect all soil salinity classification in the northern Nile Delta?
2. The latitude and longitude grids in Figures 5-7 should not be clipped to region.
3. In the discussion, please compare the accuracy of the method for predicting soil salinity in this paper with relevant conclusions from other publications.
4. Line 408-409 should be deleted.
5. Line 438-445, the format of the unit needs to be corrected.
Reviewer 2 Report
The submitted research manuscript focus on predicting soil salinity based on feature selection strategies and regression learning.
The topic is relevant and timely. The manuscript is well-structured overall, but it needs many modifications that we will point out in the comments sections below.
1- This paper aims to construct a robust spatial prediction (regression) model to map saline soils. Therefore, the introduction should be modified and focusing more about assessment of machine learning models and used feature selection strategies.
2 the proposed soil salinity classes (section 2.1) in the methods should have a reference or based on a study that the authors already conducted it.
3 Additional details about the soil sample (EC, histograms, Standard deviation, Variance...) should be added in order to understand the context and extent of salinization in the investigation area.
4 the recommendation in the conclusion are useless since they are not related to the paper content and objectives.
5 A new conclusion should be developed in order to synthesize the outcomes of this research work, demonstrate the importance of the proposed models and methods, and to propel the readers to a new view of the subject.
6 Section 4 ( discussion) does not include a comprehensive discussion of your results. You describe your results but not deeply analyze some finding and compare those against previous research. I recommend a standard discussion of your results.
The Authors may need to define the problem (and research questions) better and provide a research design the intends to fill the scientific gap (if any). A research article is completely different from an engineering/industry report.