Comparative Study on Remote Sensing Methods for Forest Height Mapping in Complex Mountainous Environments
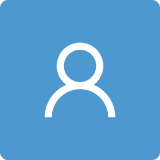
Round 1
Reviewer 1 Report
Forest canopy height is one of the key parameters for estimating carbon sink. Since the satellite-based radar can only obtain discrete canopy heights, this paper integrates optical remote sensing image data based on two different prediction models (parametric model and non-parametric model) to finally obtain continuous canopy heights. (By comparing the inversion accuracy of the two prediction models, it is concluded that the optimal feature random forest regression has relatively high accuracy, and the empirical function regression model is not suitable for canopy height inversion under complex terrain conditions because it does not consider topographic and meteorological factors, resulting in poor inversion accuracy.) However, there are still minor issues to be solved:
(1) Line52: Can you briefly introduce the difference between the two data formats of satellite-based lidar
(2) The paper mentions that the study area has typical horizontal and vertical zonality, and I would like to ask the authors how to select the dominant tree species type to complete the canopy height inversion (is the dominant tree species selected based on the zonality characteristics of the study area or based on the number distribution characteristics of tree species?)
(3) Line144: ATL03 data are vulnerable to interference from solar background noise, resulting in a large number of noise photons mixed in its signal photons. I would like to ask the authors how to deal with this problem.
(4) What is the main selection of feature variables.
(5) How to choose the upper and lower thresholds of noise segmentation?
(6) What is the basis for choosing MDA as the evaluation variable importance?
(7) The selection of high-precision training samples and the adjustment of specific parameter models are the main factors to improve the accuracy of prediction models.
(8) Line309: How were the ground measurement data points obtained to verify the accuracy of the inversion in the article?
(9) Can you give a brief description of some of the characteristics of the selected dominant tree species?
(10) The article mainly wants to solve the problem of integrating continuous data from discrete data, and then using two regression prediction models for inversion, detailing the two models and analyzing the results.
(11) Line206: Which is the spatial clustering method used? How do the different clustering models affect the results?
Author Response
Please see the attachment.
Author Response File: Author Response.pdf
Reviewer 2 Report
This study compared the performance of parametric and non-parametric models in predicting forest canopy height of different tree species. I think this study is meaningful, however, some details of data processing and modeling is not clear. In addition, there are some writing problems that need to be clarified. Some specific problems and comments are seen as the following.
COMMENTS:
Lines 13-15: suggest author add the data you use here.
Line 24: The full name of R2 should be given.
Lines 46-51: These two sentences are important, I think some references should be given to support it.
Line 59: You can't assume that all readers know ICESat, so please give the full name when it first appears in the article. In addition, ICESat instead of ICESAT. Check the whole manuscript.
Lines 75-92: ICESat-2 is the main data in this study, I think you can add some introduction to ICESat-2 here.
Lines 135-136: Please add the full name when it first appears in the manuscript.
Line 138: ICESat-1 to ICESat.
Line 159: What is the method of synthesis: maximum, minimum or median ?
Lines 173-175: Forest survey data are only used for spatial distribution maps of tree species ? You should add more information about the validation data.
Line 253: I feel that the full name should come before the abbreviation. Please check the whole manuscript.
Line 272: Again, please add full names for R2 and RMSE when they first appear in the manuscript.
Table 3: “Number of validation samples” is 200. But you write in line 283 that the number of all samples is 200. Please check.
Lines 314-318: Please clarify if the data used for modeling here is consistent with section 4.1.
Line 318: The ratio is 3:7 ? Please check.
Lines 327-328: How the importance ranking is combined: Sum, average or other?
Table 4: RMSE missing units.
Line 372: The 50 ground-measured canopy height data include five dominant tree species ? If it is, independent validation of different tree species could be considered in this study.
Author Response
Please see the attachment.
Author Response File: Author Response.pdf