Optimization of Remote-Sensing Image-Segmentation Decoder Based on Multi-Dilation and Large-Kernel Convolution
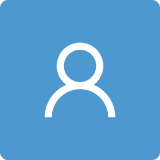
Round 1
Reviewer 1 Report
Comments and Suggestions for AuthorsThe reviewed article is interesting and well-written. Its publication does not raise any doubts in my mind.
Minor editorial errors indicated in the text of the article in the attached PDF file require correction.
I believe that two issues need to be clarified:
1. Computational requirements of the proposed algorithm in relation to the older ones compared in the final table (Table 3).
2. Discussion regarding the universality of the proposed algorithm. Are the two data sets used to test it clear evidence that it will be better in every situation?
Comments for author File: Comments.pdf
Author Response
Reviewer 1
Review Report Form
Comments and Suggestions for Authors
The reviewed article is interesting and well-written. Its publication does not raise any doubts in my mind.
Minor editorial errors indicated in the text of the article in the attached PDF file require correction.
I believe that two issues need to be clarified:
- Computational requirements of the proposed algorithm in relation to the older ones compared in the final table (Table 3).
- Discussion regarding the universality of the proposed algorithm. Are the two data sets used to test it clear evidence that it will be better in every situation?
Reply to Reviewer 1:
We grateful thank for your review. Your detail review comments really help us a lot to improve our manuscript.
- When designing our decoder, we considered some necessary measures to reduce the computational requirements at the algorithm level. However, in the actual deployment of the algorithm, we found that the computational requirements were not reduced to our expectations, so we will not be able to pay too much attention to the computational requirements in this paper. We also made corresponding modifications that mentioned computational requirements. In the next phase of our work, we will focus on the computational requirements of this decoder. Thank you for your advice.
- We tested our decoder on the Vaihingen dataset and Potsdam dataset. The results in Table 3 show that our algorithm does not show the best performance only in the test of "Tree" on the Vaihingen dataset, but is the best in the other tests. But it still beats its performance on older algorithms. It can be said that our decoder has better performance in the vast majority of scenarios.
Editorial errors mentioned in the PDF files have been corrected. Finally, we want to express our appreciation to the reviewer again.
Yours Sincerely,
Guohong Liu, Cong Liu, Xianyun Wu, Yunsong Li, Xiao Zhang, and Junjie Xu
Author Response File: Author Response.pdf
Reviewer 2 Report
Comments and Suggestions for AuthorsLand cover segmentation is a fundamental task, and this paper proposes an improved large kernel-based semantic segmentation framework for it. Even though this paper has done much work, there are still many issues and content that need to be revised and explained.
1. Extensive editing of the English language is required. For example, the formulation of the title ‘An improved large kernel-based remote sensing land cover segmentation algorithm’ is inappropriate.
2. The abstract and conclusions need to be considered for rewriting as they do not fully describe the contribution of this work. Besides, the abbreviations should be avoided from appearing in the abstract.
3. The introduction to related work is lacking, including a large number of existing segmentation methods involving multi-scale fusion and attention mechanisms.
4. In the section Methods, there should be an overall description and a segmentation network diagram for the proposed segmentation network.
5. The used network structures, such as the large kernel convolution, multi-dilation rate convolutional fusion, and channel and spatial attentions, were all proposed by predecessors. What are your contributions?
6. In the section Experiment, all comparison methods are in the field of computer vision. There are many methods applied to the remote sensing community that need to be referenced and compared.
7. In the presentation of segmentation results, such as from the ablation experiments, it is difficult to determine the role of each module solely from the segmentation map. The authors are suggested to analyze them from the feature maps and attention maps.
8. In addition, the visual samples for the experimental are lacking, which is insufficient to reflect the effectiveness of the proposed method.
Comments on the Quality of English LanguageExtensive editing of English language required.
Author Response
Reviewer 2
Comments and Suggestions for Authors
Land cover segmentation is a fundamental task, and this paper proposes an improved large kernel-based semantic segmentation framework for it. Even though this paper has done much work, there are still many issues and content that need to be revised and explained.
- Extensive editing of the English language is required. For example, the formulation of the title ‘An improved large kernel-based remote sensing land cover segmentation algorithm’ is inappropriate.
- The abstract and conclusions need to be considered for rewriting as they do not fully describe the contribution of this work. Besides, the abbreviations should be avoided from appearing in the abstract.
- The introduction to related work is lacking, including a large number of existing segmentation methods involving multi-scale fusion and attention mechanisms.
- In the section Methods, there should be an overall description and a segmentation network diagram for the proposed segmentation network.
- The used network structures, such as the large kernel convolution, multi-dilation rate convolutional fusion, and channel and spatial attentions, were all proposed by predecessors. What are your contributions?
- In the section Experiment, all comparison methods are in the field of computer vision. There are many methods applied to the remote sensing community that need to be referenced and compared.
- In the presentation of segmentation results, such as from the ablation experiments, it is difficult to determine the role of each module solely from the segmentation map. The authors are suggested to analyze them from the feature maps and attention maps.
- In addition, the visual samples for the experimental are lacking, which is insufficient to reflect the effectiveness of the proposed method.
Reply to Reviewer 2:
We grateful thank your review. Your detail review comments really help us a lot to improve our manuscript.
- We apologize for the English editing problems in this article. We have corrected the obvious English grammar problem in this paper and changed the title to " Optimization of Remote Sensing Image Segmentation Decoder Based on Multi-dilation and Large Kernel Convolution".
- We have revised the abstract and conclusions to make our contribution more prominent. Abbreviations that should not appear in the abstract are also removed. We have made changes to the abstract from line 12 to line 30, and also revised the conclusion from line 414 to line 426.
- We have supplemented the introduction with some work related to multi-scale fusion and attention mechanisms. Thank you for your advice. We have made changes from line 35 to line 69.
- We have added the overall description and a segmentation network diagram in the section Methods, which indeed makes the structure and purpose of our article clearer. Thank you for your advice. We have made changes from line 95 to line 118.
- We apologize for the lack of clarity in the description of our contributions due to our editorial problems. It is true that these network structures have been proposed by predecessors and have made remarkable achievements. However, these network structures can still produce interesting chemical reactions between them. We noticed that few previous studies have proposed combining their characteristics, so we modified the decoder and got positive results. Thank you for your careful analysis and suggestions on our work. We have re-described our contributions from line 77 to line 88.
- We apologize for the oversight in comparing the performance of algorithms. It is true that there are many efficient and interesting methods in the field of remote sensing. However, the approach we have adopted in this paper is the design of the decoder for remote sensing object segmentation tasks based on a deep learning encoder-decoder model, without proposing a complete encoder-decoder structure. Therefore, it is more meaningful that we have chosen to compare only deep learning-based remote sensing object segmentation algorithms. In future work, we are very willing to attempt to design a complete and efficient network architecture, thereby making our work more referentially valuable in the field of remote sensing.
- Your suggestion is very reasonable. However, limited by time arrangement and workload, we cannot analyze and compare a large number of feature maps and attention maps in this paper, but we can start from the theoretical perspective and provide some evidence appropriately. Thank you very much for your advice and understanding. We have made changes from line 363 to line 374.
- Your suggestion is very reasonable, and we have added the necessary visual samples as a supplement to make our conclusion more convincing. We have made changes from line 375 to line 393.
Finally, we would like to express our apologies for the omissions and problems in our article and our thanks to the reviewers for their suggestions again. Through the analysis and modification of these suggestions, the quality of our manuscript has been greatly improved.
Yours Sincerely,
Guohong Liu, Cong Liu, Xianyun Wu, Yunsong Li, Xiao Zhang, and Junjie Xu
Author Response File: Author Response.pdf
Reviewer 3 Report
Comments and Suggestions for AuthorsLand cover segmentation, a fundamental task within the domain of remote sensing, boasts a broad spectrum of application potential. The paper should provide a more detailed interpretation of results and indicate potential directions for future research. Here are a few comments and suggestions:
Further citations and references are needed in the Introduction. For instance, for the first pargrap: The importance of land cover segmentation and the use of image semantic segmentation algorithms need citation.
Indeed, the title "Results" would typically suggest an account of the outcomes derived from your implemented method. If this section instead provides details about the datasets used and how your model was implemented, a more fitting title might be needed. You can reorganize the paper and add move the details about the datasets and implementation into the "Methodology". Then use the "Results" section to discuss the outcomes and findings of your research. If reorganizing the paper isn't feasible, you could adjust the section's title to better reflect its content, i.e., "Implementation and Datasets".
In your discussion, aside from describing the results of the study, it would be beneficial to further elucidate why your method was able to attain these outcomes. For instance, how the MDCFD decoder and the LKSHAM module led to performance improvements in specific cases. Besides, when reporting the specific improvement percentages, citing some baselines or other related works for comparison with your methods could help readers better understand these numbers. Additionallly, further detailing how the redesign of the convolution kernel not only minimized computational demands but also elevated model segmentation efficiency across varying scales through tailored convolution kernel strategies can provide more clarity about your methodology. Consider discussing some potential limitations or challenges, which will add depth and comprehensiveness to your discussion.
Comments on the Quality of English Language
English is fine for me.
Author Response
Reviewer 3
Comments and Suggestions for Authors
Land cover segmentation, a fundamental task within the domain of remote sensing, boasts a broad spectrum of application potential. The paper should provide a more detailed interpretation of results and indicate potential directions for future research. Here are a few comments and suggestions:
Further citations and references are needed in the Introduction. For instance, for the first pargraph: The importance of land cover segmentation and the use of image semantic segmentation algorithms need citation.
Indeed, the title "Results" would typically suggest an account of the outcomes derived from your implemented method. If this section instead provides details about the datasets used and how your model was implemented, a more fitting title might be needed. You can reorganize the paper and add move the details about the datasets and implementation into the "Methodology". Then use the "Results" section to discuss the outcomes and findings of your research. If reorganizing the paper isn't feasible, you could adjust the section's title to better reflect its content, i.e., "Implementation and Datasets".
In your discussion, aside from describing the results of the study, it would be beneficial to further elucidate why your method was able to attain these outcomes. For instance, how the MDCFD decoder and the LKSHAM module led to performance improvements in specific cases. Besides, when reporting the specific improvement percentages, citing some baselines or other related works for comparison with your methods could help readers better understand these numbers. Additionallly, further detailing how the redesign of the convolution kernel not only minimized computational demands but also elevated model segmentation efficiency across varying scales through tailored convolution kernel strategies can provide more clarity about your methodology. Consider discussing some potential limitations or challenges, which will add depth and comprehensiveness to your discussion.
Reply to Reviewer 3:
We grateful thank your review. Your detail review comments really help us a lot to improve our manuscript.
- Thank you for your meticulous review. We have already added references to the importance of land cover segmentation and the use of image semantic segmentation algorithms in the introduction. We have made changes from line 35 to line 69.
- We have carefully analyzed your comments and adjusted the Methods section and Results section. Thank you for your advice. We have made changes from line 261 to line 394.
- We have described in more detail how the MDCFD and LKSHAM modules work. In addition, in the analysis of performance indicators, the baseline indicators are also explained. As for computational demands, we also make a more in-depth analysis from the theoretical perspective. We have made changes from line 95 to line 118 and from line 395 to line 412. Thank you for your detailed suggestions and understanding.
Finally, we want to express our appreciation to the reviewer again. The reviewer’s suggestion does improve the quality of our manuscript a lot.
Yours Sincerely,
Guohong Liu, Cong Liu, Xianyun Wu, Yunsong Li, Xiao Zhang, and Junjie Xu
Author Response File: Author Response.pdf
Round 2
Reviewer 2 Report
Comments and Suggestions for AuthorsThe authors have made revisions to most of the comments and suggestions provided, but there are still some issues that need to be considered before acceptance.
(1) The introduction and related work should be elaborated in a larger context, and a large number of important relevant references should be added.
(2) Suggested comparison methods in remote sensing fields should be based on the deep learning frameworks with encoder-decoder structures, rather than traditional active learning methods.
(3) I still believe that the author needs to analyze the feature maps and attention maps to verify the effectiveness of the proposed method.
Comments on the Quality of English LanguageMinor editing of English language required.
Author Response
Dear Editor,
We really appreciate for all the reviewers’ comments and suggestions. Thanks for their great contribution to this manuscript. updated the contact email addresses. The following is our reply to reviewer’s comment and suggestion.
Reviewer 2 Round 2
Comments and Suggestions for Authors
The authors have made revisions to most of the comments and suggestions provided, but there are still some issues that need to be considered before acceptance.
(1) The introduction and related work should be elaborated in a larger context, and a large number of important relevant references should be added.
(2) Suggested comparison methods in remote sensing fields should be based on the deep learning frameworks with encoder-decoder structures, rather than traditional active learning methods.
(3) I still believe that the author needs to analyze the feature maps and attention maps to verify the effectiveness of the proposed method.
Reply to Reviewer 2:
We grateful thank your review. Your detail review comments really help us a lot to improve our manuscript.
- To enhance the depth and breadth of the introduction, we have conducted a more comprehensive review of the related work, with a significant number of additional references incorporated. The specific revisions can be found in lines 36 to 67 of the document.
- Following the excellent suggestions, we have made additional enhancements in Table 3, incorporating extra methods for comparative analysis with our approach.
- Thank you for your suggestions. We have included an analysis of feature and attention maps in the 'Visual Results' section, thereby further elucidating the roles of the various modules within our decoder
Finally, we would like to express our sincere gratitude once again to the reviewers for their invaluable suggestions. The quality of our manuscript has been significantly enhanced, thanks to the constructive feedback provided by the reviewers.
Yours Sincerely,
Guohong Liu, Cong Liu, Xianyun Wu, Yunsong Li, Xiao Zhang and Junjie Xu