Decomposition-Based Soil Moisture Estimation Using UAVSAR Fully Polarimetric Images
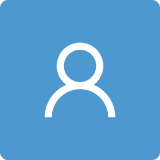
Round 1
Reviewer 1 Report
Review of “Decomposition Based Soil Moisture Estimation Using UAVSAR Fully Polarimetric Images” by Akhaven et al. for the journal “Agronomy”.
I read this manuscript with great interest and was impressed with the methodological approach and findings, and the quality of the writing. I am not a radar polarimetry expert but I am familiar with radar remote sensing for soil moisture retrieval and empirical modelling methods (including with machine learning). As a non SAR polarimetry expert, I appreciated the clear explanation of the different polarimetric decompositions that were used. The testing dataset used seems reliable and effective and I have no major criticism of this paper. I have recommended to accept with minor revisions. Below I detail my main questions/criticisms, followed by a list of minor comments or corrections.
Main comments:
- It is explained briefly, in one location, that a 75% training and 25% validation dataset was used. I would appreciate more clarity around this. Was a random split created for each of 50 iterations of each model? Are all accuracy metrics based on the 25% validation dataset? I was very surprised to the see the “all features” models have the highest cross-validated accuracy this is quite unusual and suggests no over-fitting even in these “all feature” cases. It implies to me that the initial selection of features to reduce redundancy worked well. Overall, please re-iterate and emphasize that all accuracy metrics were based on the cross validation. I am not sure that 50 iterations was required – a 4-fold cross-validation would suffice.
- There is very little reporting or discussion of variable importance. This is a key strength of the RF method and there is a sentence in the conclusion about which variables were most important, but no reporting or discussion of this information. I highly recommend to include variable importance metrics from the RF models and improve discussion of these results.
- The finding that surface roughness is important for the prediction soil moisture is not surprising but important, nonetheless. There is relatively little discussion of this, including the fact that it leads to an upscaling problem whereby you can’t produce such high-accuracy soil moisture retrieval anywhere except where you have surface roughness estimates at the field measurement sites. I feel this warrants additional discussion and perhaps some suggestions of how the surface roughness issue could be resolved for broader scale soil moisture retrieval (e.g. a landcover transfer function/lookup table approach, or estimation for very high resolution bare earth DEMs)?
- Why was Pearson correlation used instead of Spearman rank correlation, for the pre-screening of feature correlations? It is quite possible that key correlations were missed because of this. It is also at odds with the justification provided by the authors’ that the machine learning algorithms deal effectively with non-linearities. It is therefore implied that there are probably non-linear relationships in this dataset (probably because of non-normal distributions) and therefore it doesn’t make sense to use Pearson correlation. The correlation analyses should be rerun with Spearman rank and results updated to reflect any changes (hopefully few to none, but must be verified).
- Related to (4) above, some mention of the variable distributions should be included and justification provided for not transforming them (i.e. not required for the particular machine learning algorithms that were chosen). But of course, for Pearson correlation, they should have been transformed, hence the need to use Spearman rank instead.
- Please confirm and clarify that all model R2 values are cross-validated R2 values (same with RMSE and MAE)? Similarly the term accuracy should only be used for cross-validated metrics otherwise it would be misleading. I hope that all reported R2 values are cross-validate though, correct?
Minor comments:
R2 values are reported to three decimals of precision. This seems a bit optimistic to me. Suggest to round to two decimals throughout.
What statistical software was used for the analyses? It should be explained along with any relevant packages, with citations.
Line 103: What is the metric of soil moisture estimation accuracy reported here (RMSE?)
Line 143: Explain what kind of probe this is (i.e. based on what technology). What is its precision and accuracy? How is it calibrated?
Line 149: You must have collected GPS position of observations so please explain with what GPS model and with what accuracy.
Line 183-184 Unclear sentence please re-write.
Line 293 Unclear what the accuracy metric here is.
Line 262: Identify the three feature selection methods here by name, even if you are explaining them later.
Line 264: These are essentially just correlation matrices I am not sure “Heatmap” is necessary wording since it is more general and can be applied to other types of data. If you disagree, then please define properly to justify why these are called Heatmaps (and why capitalized?) – e.g. maybe because the plot also attempts to group clusters of features, which is useful.
Line 266 “In some studies” – funny wording – features that are highly correlated are going to be redundant, period. Not just in some studies. Please reword.
Line 279 – 284 Note - here you are referring to accuracy but I am not sure if this refers to cross-validated accuracy or not. I would suggest to refer to the model R2 as “goodness of fit” and the cross-validated R2 and RMSE as accuracies. Also, you are explaining aspects of the modelling steps before you have introduced the main modelling steps (this part is about the pre-selection process based on correlation analysis) so it gets confusing here.
Line 302 The ordering of methods is a bit odd because here you are referring to the ML algorithms but you have already spent a fair bit of time explaining the feature selection process based on model accuracies (unless I mis-understood – either way, some re-structuring or clarification is required).
Line 326 You don’t really need to normalize the feature data in this way for RF so please explain why it was done.
Line 334-335 This is the first mention of using a training and validation dataset hence my previous confusion and comments around this issue.
Line 336 As noted previously – did you re-generate the training and validation set each time out of 50? I am not sure why you didn’t just use 4-fold cross validation. How did you choose 50 and what is the purpose over a k-fold approach? Please justify.
Line 368-370 Remove!
Figure 4 caption As noted consider removing “Heatmaps” descriptor and reminder that this should be based on spearman r not Pearson r.
Table 6 caption As noted previously, I am very surprised that “all features” accuracies were the highest which suggests that either these accuracies are not cross-validated accuracies, or the initial redundancy reduction process worked well. Please make sure these are cross-validated. If so, great!
Figure 5 and caption: Is the red line a 1:1 line or line of best fit? It is hard to tell. Both should be shown, and this should also be explained in the caption. Any important deviations from 1:1 line should be noted in results/discussion.
Line 474-475 Unclear wording please fix.
Line 478 “acted well in non-linear relationship” I find this unclear.
Line 504-505 Here you mention feature importances but this is not reported or discussed anywhere else.
Literature Review/Discussion sections: I believe there is at least one paper by K. Millard that looks at soil moisture retrieval with SAR polarimetry. Please make sure this statement is accurate and include any missing references by this or any other authors where warranted. Would it make sense to tabulate all of the research on the topic of soil moisture retrieval with SAR polarimetry? That would provide a nice, quick reference for the background info on soil moisture retrieval with RADAR and the specific methods used plus reported accuracies.
Author Response
Please see the attached file.
Author Response File: Author Response.pdf
Reviewer 2 Report
Comments This manuscript proposes a method of exploring the sensitivity of different scattering mechanisms extracted from Freeman-Durden, Van Zyl, and H/A/α decompositions to soil moisture in corn, wheat, and soybean fields. They used three feature selection algorithms including Trial and Error, Backward, and Forward feature selection algorithms are tested and compared to determine the best algorithm and most useful features. They asserted that the most accurate soil moisture estimates are derived from the Random Forest regression algorithm for soybeans, with a correlation of determination (R2) of 0.868, root mean square error (RMSE) of 0.041 m3m−3, mean squared error (MSE) of 0.001 m3m−3, and mean absolute error (MAE) of 0.030 m3m−3. Emphasizing that the feature selection also impacted results. Moreover, they found that the features identified as the most effective deliver the highest soil moisture accuracies. The presented statistical parameters are sufficient to evaluate the methods used. The description of the results is sufficient. They discuss and the conclusions do not fully satisfy me. I suggest to divide the discussion and conclusions. The discussion should be supplemented with the results of other authors, even those presented in the introduction. Synthetically formulated conclusions are welcome.Author Response
Please see the attached file.
Author Response File: Author Response.pdf
Reviewer 3 Report
Using thousands of ground soil moisture points and surface roughness information, the authors evaluate the ability of decomposition variables from L-band SAR polarimetric images to predict soil moisture. The authors employ three feature selection algorithms and two machine learning algorithms to evaluate the importance of the polarimetric decomposition variables to predict soil moisture. They find that soil moisture under soybean, corn, and wheat cover can be predicted with high accuracy from SAR polarimetric images and roughness variables.
I believe that with revision, this paper is appropriate for publication in Agronomy journal and it should be of interest to readers of this journal.
GENERAL COMMENTS
- When (date or month) was the UAVSAR remote sensing conducted? It is not clear from reading if only a one-time image was used. In the abstract, you mention a time series of polarimetric UAVSAR data is acquired (lines 18 – 19). Please clarify this in Sections 2.2 and 2.3. If a time series of images is used, please elaborate on how it was used.
- The final data size is not provided. Please explicitly give the total number of samples somewhere in the data description. From reading section 2.3 it seems that there were 44 fields (excluding canola, pasture, and forage fields) leading to a total of 2,112 samples by my calculation. That is,
(soybeans + wheat + corn) * sampling points * replicates =
(19 + 16 + 9) * 16 * 3 = 2112.
Is this correct? - L-band SAR backscatter is affected by vegetation cover (you also mention this in lines 456-457, 461-462). You have accounted for the different vegetation types by building separate models, but you have not accounted for the different growth stages. Given the effect of vegetation cover do you think it would have been a good idea to include vegetation metrics such as LAI or NDVI? This seems relevant given that you had already collected information in this regard (lines 159 – 167).
- Given that RF handles categorical variables very well, did you consider building a model that can work in all three land cover types using crop type as an input (either SB, WH, or CO)?
- I suggest you do not use the MSE metric. It is redundant with RMSE. The RMSE is more appropriate since it is in the same units as the output (i.e., m3 m-3). In addition, the MSE values are not useful at the three decimal places rounding you provide (all MSE’s ~0.001). I suggest you include a measure of bias such as the mean error (ME).
- Once you identify the optimal inputs required using the feature selection approaches, the rest of the results and discussion should focus on that ‘optimal-input’ model, otherwise, it defeats the purpose of doing feature selection in the first place. I believe it is unnecessary to report detailed results from each of the three feature selection approaches. Doing so would reduce figures 5 and 6 to three panels each and help make the results and discussions more concise.
- Similarly, once you identify the best algorithm (i.e., RF) it is best if you continue the discussion with only the best algorithm (except in Section 3.4). Bringing NN to the discussion elsewhere unnecessarily complicates the reading.
- Given that the main objective of this work is stated to be the exploration of the importance of different scattering mechanisms extracted from different decomposition methods (lines 107 – 108), it is important that you provide a ranking of the variable importance across the models. You have listed four of the most important features in the last paragraph of the manuscript (lines 503 – 504). It would be much more appropriate if you could have reported and highlighted this earlier and discussed this more.
- Section 3.5 (Comparison with other studies) needs some more work to clarify the comparisons and how this study differs from the other studies.
SPECIFIC COMMENTS
Please find additional specific comments in the attached PDF file.
Comments for author File: Comments.pdf
Author Response
Please see the attached file.
Author Response File: Author Response.pdf