An Improved Lightweight Network for Real-Time Detection of Apple Leaf Diseases in Natural Scenes
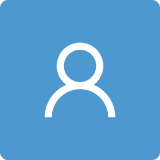
Round 1
Reviewer 1 Report
The paper shows some very good results. However, I have a two remarks about it:
1. The methodology is very long. You don't need to explain in details "well documented and known methodologies", it's sufficient to cite it.
- For example - Augmentation. This is an essential step in convolution NN, but i would argue that you don't need the lengthy explanation about it.
- Same goes for annotation. It doesn't influence the results what format the annotation is, Nowadays there are many parsers that can generate/translate annotations from one format to another. Thus i would suggest remove the picture 2.
- Repeating myself here again, reconsider removing the formulas that you don't reference but you have already placed in the manuscript. I think that F1 and mAP are enough.
2. My main concern is here: the overall structure of the paper.
- As a research paper reader, I'd expect the paper to follow the kind of IMRAD format (Introduction, Methods, Results, and Discussion). I had difficulty understanding when the paper was explaining results or methodologies. And I understand that in more technical papers like this one, there is no clear line between the two, still better structure is needed.
- Example: Is Distance-Intersection over Union something you have developed or you are describing it from other research - so from the YOLOX-Nano? If the later, then consider it making it more obvious and clearly draw relation to why you need to mention it and how will it influence results.
- Your titles are: 1. Introduction, 2. Construction of multi-scene..., 3. Dedection model for ..., 4. Implementation details... 5. Conclusions. I can understand that for you makes sense, but for someone that wants to read the paper, there is no clear structure what is methodology, what is result.
I think that if you would just structure better the paper, this will be a much pleasant read and an excellent paper.
PS: Consider reading this:
https://en.wikipedia.org/wiki/IMRAD
Author Response
1、Thanks to your advice, I have made some adjustments to the "well documented and known methodologies" section of the article. For example, in the datasets section, the “data processing” chapter is now used to replace “data annotation” and “data enhancement”, and is only briefly introduced. And the unnecessary images have also been removed. But I think it may be necessary for formulas like Precious and Recall, which will be used later in the table, and the mAP and F1 are calculated based on them.
2、According to your suggestions, the structure of the article has been optimized. The article is now structured as 1. Introduction, 2. Materials and Methods, 3. Results and Discussion, 4. Conclusions, and each small section has also been improved. I think it might be clearer.
Reviewer 2 Report
This study proposes an object detection-based approach for the identification of apple diseases. They proposed a lightweight model based on the architecture of YOLOX-Nano Network.
The proposed work has shown significant results in identifying the diseases of Apple. The manuscript is well written and can be considered for publication. But before that, I have some observations regarding the manuscript such as:
1. In the abstract, the authors should mention the proposed model i.e. YOLOX-ASSANano prior to mentioning its results.
2. In the manuscript, the authors mentioned that they applied image augmentation after the annotations task. If this is the case then how did they handle the bounding box coordinates for the newly generated images (esp. by flipping, scaling, rotating, cropping)?
3. In methodology, kindly itemize and explain properly the improvements done in each module (wherever applicable) as it is not clear. And also refine figure 3 with more clarity about the modules/blocks used in the network.
4. It is suggested to add a new section as 'Results and Discussion' or something like that to present the results and discuss their implications and put the subsections 4.4-4.7 into this section.
5. Authors are suggested to provide a performance comparison of their work with the previous studies done on similar kinds of datasets. For this, they can follow this article https://doi.org/10.1038/s41598-022-10140-z
6. The conclusion needs to be improved a bit by both language and content. Also, discuss the limitations of this study along with the future scope.
Author Response
1、Your comments are much appreciated and we have made a little change to the abstract of the paper. YOLOX-ASSANano is presented before presenting the detailed improvements.
2、For HSV transformations, this mainly involves changes to the hue, saturation and exposure of the image and does not affect the position of the target in the image. For flipping, scaling, rotating, cropping and mosaic operations, the position of the target in the image is changed, so the label in the corresponding xml file needs to be changed as well. For example, if the image is flipped up or down, the label box needs to be labels[:,2]=1-labels[:,2]; if the image is flipped left or right, the label box needs to be labels[:,1]=1-labels[:,1]; similarly for all enhancements where the position of the target is changed, the label is recalculated and the coordinates.
3、We have optimised Figure 3 to minimise the use of abbreviations to represent modules. And some adjustments have been made to the description of the Methods section, which may be clearer. The main improvements in our work include four aspects: The asymmetric shuffleblock is proposed using the idea of feature fusion; The introduction of the SA attention mechanism in the CSP module for refining intermediate features; DSC is replaced with BSConv as an efficient building block for our backbone network. Faster convergence and better performance were achieved using CIoU loss.
4、Following your suggestion, I have optimized the structure of the article to follow the kind of IMRAD format (1. Introduction, 2. Materials and Methods, 3. Results and Discussion, 4. Conclusions). The subsections 4.4-4.7 have been placed in the third section “Results and Discussion”. And some other sections have also been improved.
5、Thank you for your suggestion, it is very important to compare the proposed model with other methods. In the study, we have compared the performance with previous studies done on the public dataset PlantDoc, F1 and mAP results are compared in Section 3.3, and visual analysis is carried out in Section 3.4.
6、According to your suggestion, we have made some optimisations in the Section "Discussion". It may summarise our work more clearly.
Overall, thank you very much for your suggestions, and please do not hesitate to contact me if there are any other suggestions later.