Breast Cancer Histopathological Image Recognition Based on Pyramid Gray Level Co-Occurrence Matrix and Incremental Broad Learning
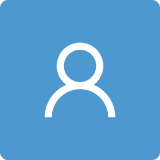
Round 1
Reviewer 1 Report
The authors report on the development of deep learning-based breast cancer histopathological image recognition algorithm. The paper itself is well-organized and -written and can be published in present form.
Author Response
Thank you for your recognition of the research content of this paper. For the literature you mentioned, the author has classified and summarized the literatures in the introduction, please check it.
Reviewer 2 Report
Well organized and an interesting report.
Author Response
Thank you for your approval of the research content of this paper. The author has spell-checked the manuscript for English language and style.
Reviewer 3 Report
The authors introduced a novel method for breast cancer tumor classification from histopathological image data.
Below are my comments:
Figure 5 needs to have labels of the steps depicted (as described in the text)
Through several experiments, it is verified that when the number of feature node windows is 200, the number of feature nodes in each window is 100, the number of enhancement nodes is 800, and the number of addition enhancement nodes reaches 10,000, a better recognition effect and a higher real-time performance can be obtained. – what is the range used for each parameter to validate the best setting?
A (Adenosis) as an example: there are 1066 images in the test set, the number of images correctly classified by the network is 1013, the number of images incorrectly classified as F (Fibroadenoma) is 11, accounting for 0.13% of the total test set; the number of images incorrectly classified as PT (Phyllodes Tumor) is 10, accounting for 0.12% of the total test set... , - this sentence looks incomplete
Error report looks busy, instead can be summarized as a table: accuracy, precision, recall F1 score
Have alternative models from the comparative analysis been tuned?
Is code implementation available?
The patients sample size is too small for such a heterogeneous condition and external dataset validation is highly recommended.
Author Response
Please see the attachment.
Author Response File: Author Response.docx
Reviewer 4 Report
This work is not enough contribution and innovation. However, the problem statement and motivation could be stronger or more clearly highlighted.
1. The existing literature should be classified and systematically reviewed, instead of being independently introduced one-by-one.
2. The abstract is too general and not prepared objectively. It should briefly highlight the paper's novelty as what is the main problem, how has it been resolved and where the novelty lies?
3. For better readability, the authors may expand the abbreviations at every first occurrence.
4. The author should provide only relevant information related to this paper and reserve more space for the proposed framework.
5. However, the author should compare the proposed algorithm with other recent works or provide a discussion. Otherwise, it's hard for the reader to identify the novelty and contribution of this work.
6. The descriptions given in this proposed scheme are not sufficient that this manuscript only adopted a variety of existing methods to complete the experiment where there are no strong hypothesis and methodical theoretical arguments. Therefore, the reviewer considers that this paper needs more works.
The algorithm presented has not any novelty.
7. The related works section is very short and no benefits from it. I suggest increasing the number of studies and add a new discussion there to show the advantage. Following studies can be added.
a. Dilated semantic segmentation for breast ultrasonic lesion detection using parallel feature fusion
b. Breast-cancer detection using thermal images with marine-predators-algorithm selected features
c. A quantization assisted U-Net study with ICA and deep features fusion for breast cancer identification using ultrasonic data
d. Breast Cancer Mammograms Classification Using Deep Neural Network and Entropy-Controlled Whale Optimization Algorithm
e. Breast Cancer Detection and Classification using Traditional Computer Vision Techniques: A Comprehensive Review
8. The manuscript is not well organized. The introduction section must introduce the status and motivation of this work and summarize with a paragraph about this paper.
Author Response
Please see the attachment.
Author Response File: Author Response.pdf
Reviewer 5 Report
This manuscript proposes a breast cancer histopathological image recognition method based on pyramid gray level co-occurrence matrix (PGLCM) and incremental broad learning (IBL) model. Evaluation on BreaKHis dataset proved its effectiveness.
Major comments:
1. Missing references, e.g.,
The references corresponding to Line 34-36
2. In data augmentation step, have the authors ever tried to add expansion / shrinkage in one direction? Although Gaussian pyramid enables multi-scale feature extraction, it couldn’t solve expansion / shrinkage in one direction.
3. Most of the methods selected for comparison in Table 3 are not new. Please try to compare with more state-of-the-art methods.
4. Please add more detailed explanation why the proposed method is only applicable to BreaKHis dataset (Line 363).
Author Response
Please see the attachment
Author Response File: Author Response.pdf
Reviewer 6 Report
In order to provide valuable results for computer-aided diagnosis (CAD) of breast cancer, this paper proposes a method for recognizing histopathological images of breast cancer based on PGLCM-IBL. In the proposed method, the authors establish a pyramid gray level co-occurrence matrix (PGLCM) model for feature extraction and an incremental broad learning (IBL) model for classification.
Before the Editor makes a decision, I suggest that the authors must take into account the following corrections:
1. The title should be rewritten to be friendly.
2. The author should explain the novelty clearly in the abstract and conclusion.
3. The abstract is very long, should be reduced
4. The Introduction section must be more concise.
5. The paper should be carefully revised for punctuation, grammar, spelling mistakes and sentences structuring.
6. Punctuations are used randomly. Insert comma or full stop after every equation accordingly.
7. What are the advantages of the proposed new model?
8. The authors should include scientific reasoning for graphical results in details.
If the authors take into account all these corrections, then, without doubt, this manuscript deserves to be published.
Author Response
Please see the attachment.
Author Response File: Author Response.docx
Round 2
Reviewer 4 Report
The paper is relatively improved. However, the study still needs to conduct statistical validation to verify the results. So, I recommend using the ANOVA test to apply.
Author Response
Please see the attachment.
Author Response File: Author Response.pdf
Reviewer 5 Report
Comments to the Author
This manuscript proposes a breast cancer histopathological image recognition method based on pyramid gray level co-occurrence matrix (PGLCM) and incremental broad learning (IBL) model. Evaluation on BreaKHis dataset proved its effectiveness.
Major comments:
1. The authors updated the title of the manuscript, however, the current title “Breast Cancer Histopathological Image Recognition Based on Combined Model” is too general and vague. The title should highlight the specific techniques used in the proposed framework.
2. It’s better to describe with letters/metric names rather than resultant numbers in Line 258-272
Author Response
Please see the attachment.
Author Response File: Author Response.docx
Round 3
Reviewer 4 Report
The paper is well revised and can be accepted.