Simultaneous Estimation of Vehicle Mass and Unknown Road Roughness Based on Adaptive Extended Kalman Filtering of Suspension Systems
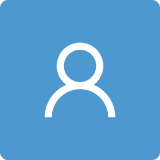
Round 1
Reviewer 1 Report
1- The novelty of the methodology is not well established (all the presented ideas are already developed in previous papers), the main focus of the paper should be the forgetting factor.
2-Authors have to improve the bibliography on the previous work. Similar works and interested studies are not cited this paper.
3- Xr in the model refers to the road profile, but the authors state that it is the road roughness (the expression between the road profile displacement and roughness must be expressed). 4- Page 4: authors state that Xb and Xw are the sprung and unsprung mass displacement (which is exact) then states that the sprung masse is X5 (false it is the tire stiffness in the model kit) and unsprung mass Xw (false)? That must be corrected. 5- In equation (10) authors use different unintroduced notations (from literature obviously) such as Xs, Cs and Ks which are in direct conflict with the notations in equation (2). 6- The idea of the forgetting factor is quite interesting, but must be properly described and mathematically introduced. It must be the focus of the paper.17- How the forgetting factor tacks the time varying parameters (and which ones) must be re-described properly.
8- In page 8, authors state that using a variance weighting approaches improves the filter converges, based on which analysis? It is a strong statement that needs to be backed with at least a proof.
1- Simulation scenarios are interesting and implementation since it is the well-known Kalman filter structure, which can be easily implemented on a real vehicle (a good point for the proposed methodology).
10- The robustness analysis section shows the performance of the proposed filter but does not give an explanation; authors must consider providing a more complete analysis.
Author Response
Please refer to the attachment.
Author Response File: Author Response.pdf
Reviewer 2 Report
Your applied methodology is very usefull in the context of electric vehicles (EVs) and autonomous vehicles (AV). For EVs this information e.g. can be used for a more precise range prediction and for AVs the informations is e.g. important for the several assistance systems. Hence, your method has a lot of benefits for upcoming as well as modern vehicles.
Here are some points of critism:
- Please check the size of the text inside the diagrams => please use in all diagrams the same size.
- Please insert some more information about the sensors as well as the sensor fusion you are using.
- In the past, a lot of science publication has been published in the context of vehicle mass estimation. Therefore, insert some more information or data to show the advantages of your system compared to the start of the art.
- Please present some measurement errors of other systems for vehicle mass estimation => is your system more precise compared to other methods / systems?
- Does metheodology parameters have an influence on your estimation? If yes: how can you handle this problem?
- Please extend your conclusion.
Author Response
Please refer to the attachment.
Author Response File: Author Response.pdf