Research on Joint Extraction Model of Financial Product Opinion and Entities Based on RoBERTa
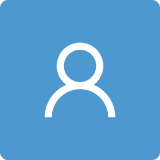
Round 1
Reviewer 1 Report
The joint extraction of entities and opinions is an interesting task, which is facilitated by the use of complex deep neural network architectures with multiple inputs and outputs. A very similar task is aspect-based sentiment analysis, which replaces named entities with aspects and opinions with sentiment.
I have noticed several typos in the whole text. A careful proofreading is needed.
On line 158 authors state that the model output is of size t>n, but they do not define how much bigger t is from n. Is it n+1, given that in the output the count starts from 0?
The idea of forwarding the outputs of the internal BERT layers to the TextCNN is straightforward and, if it improves performance, meaningful.
What can be explained in more details is the intuition behind equation 7.
What is interesting to examine in the task and dataset at hand is the complexity of the NER and classification task. The large ratio of neutral labels is not very helpful since it results in a highly unbalanced dataset. The small number of entity types, on the opposite, simplifies the task.
The selection of the hyper-parameters is neither theoretically nor experimentally justified. It would be useful to have at least a grid search approach for the best set of parameters.
The experimental evaluation compares the proposed joint model with the joint model of [25]. It is necessary in the related work section or in section 3 to explain how the baseline joint model works.
The statistical significance of the improvement achieved with the proposed model has to be examined using a t-test.
There are a few works that are missing from the related work section:
- Zhao, L., Li, L., Zheng, X., & Zhang, J. (2021, May). A BERT based sentiment analysis and key entity detection approach for online financial texts. In 2021 IEEE 24th International Conference on Computer Supported Cooperative Work in Design (CSCWD) (pp. 1233-1238). IEEE.
- Batra, S., & Rao, D. (2010). Entity based sentiment analysis on twitter. Science, 9(4), 1-12.
Author Response
Please see the attachment.
Author Response File: Author Response.pdf
Reviewer 2 Report
The paper described an original approach to joint text classification and named entity recognition. The originality of the approach lies in the utilization of CLS embeddings from all layers, not only the last one. The authors showed that this approach improved the performance. Most of the improvement was observed in the text classification task.
The proposed modification was evaluated on a single dataset, thus, it is difficult to evaluate the generality of this approach.
I have only one major concern regarding the reported results. The authors mentioned the split of the data into training and testing sets and about 10-fold cross-validation. However, tables 3 and 4 report only single values. The tables should be complemented by average values and standard deviations for the reported configurations. Please
Author Response
Please see the attachment.
Author Response File: Author Response.pdf
Round 2
Reviewer 1 Report
The authors have addressed all my comments.