Transform-Based Feature Map Compression Method for Video Coding for Machines (VCM)
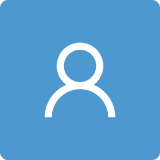
Round 1
Reviewer 1 Report
This paper presented a transform-based feature map compression approach for VCM, which achieved a considerable improvement compared with the anchor in the MPEG-VCM test. The proposed method achieved the same performance in machine vision tasks while keeping a lower bit rate.
1. Matrix used in PCA like TGB and TGM do not specify how they were obtained through training.
2. As shown in Figure 9 (b), the proposed method is inferior to the benchmark model at high bit rates.
3. The process of combining the low-level feature maps in Figure 4 was not verified by ablation experiments.
4. More related references (below) prefer to be added:
i) Chen, Sien, Jian Jin, Lili Meng, Weisi Lin, Zhuo Chen, Tsui-Shan Chang, Zhengguang Li, and Huaxiang Zhang. "A new image codec paradigm for human and machine uses." arXiv preprint arXiv:2112.10071 (2021).
ii) Chen, Zhuo, Kui Fan, Shiqi Wang, Lingyu Duan, Weisi Lin, and Alex Chichung Kot. "Toward intelligent sensing: Intermediate deep feature compression." IEEE Transactions on Image Processing 29 (2019): 2230-2243.
Author Response
Please see the attachment (Response to Reviewer 1 Comments).
Author Response File: Author Response.pdf
Reviewer 2 Report
This paper proposes a transform-based feature map compression method for video coding for machines (VCM) to enhance machine recognition through video information compression. It utilizes a principal component analysis (PCA)-based compression methodology for multi-level feature maps extracted from the feature pyramid network (FPN) structure. The proposed method eliminates the need for a separate PCA process by employing a generalized basis matrix and mean vector derived from channel correlations. It achieves further compression by amalgamating high-dimensional feature maps, taking advantage of spatial redundancy. The proposed VCM encoder does not incur any compression loss and only requires compressing the coefficients for each feature map using versatile video coding (VVC). Experimental results demonstrate superior performance over previous PCA-based feature map compression methods, achieving an 89.3% BD-rate reduction for instance segmentation tasks.
The abstract is informative and well-described. The introduction is relevant. The authors describe previous works and their limitations compared with the proposed method. The objectives and the structure of the paper are also described. The second section provides an adequate description of the proposed transform-based feature map compression method. Figures and tables are very useful. Please check that all figures are inserted into the main text close to their first citation. If possible to increase the size of the figures to become more readable. Experimental results are well-analyzed using informative tables and figures. In the conclusions, the paper does not explicitly mention any limitations or drawbacks of the proposed method. It would be useful to mention some limitations. In addition, it would be useful to highlight the importance of the research and briefly mention the implications of the proposed method.
According to my point of view, this paper can be published even in its current form.
Author Response
Please see the attachment (Response to Reviewer 2 Comments).
Author Response File: Author Response.pdf
Reviewer 3 Report
The authors propose a principal component analysis (PCA)-based compression methodology for multi-level feature maps, extracted from the feature pyramid network (FPN) structure. The paper is interesting and in a good way. However, the manuscript must be proofread by a native speaker. Also, the paper needs to present the related work, and a discussion with the comparison of the results. It is difficult to understand the improvements of the proposed method.
The manuscript contained some grammatical mistakes. It must be proofread by a native speaker.
Author Response
Please see the attachment (Response to Reviewer 3 Comments).
Author Response File: Author Response.pdf
Round 2
Reviewer 3 Report
The authors considered the previous comments, and the paper can be accepted