Brainstorming Will Never Be the Same Again—A Human Group Supported by Artificial Intelligence
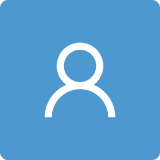
Round 1
Reviewer 1 Report
The paper "Brainstorming Will Never Be The Same Again – Human Group Supported by Artificial Intelligence" presents an interesting approach to modifying the brainstorming process using artificial intelligence (AI), specifically OpenAI's GPT-3.5-turbo model, to generate and categorize ideas. The system design of the proposed software tool, "kresilnik," and its successful application in generating ideas for a regional development plan are discussed.
However, several critical weaknesses and limitations in this study seriously compromise its validity and applicability. Firstly, the paper does not adequately clarify the selection criteria and methodology for determining "good" or "useful" ideas. This subjective assessment raises serious questions about the validity of the study and the conclusions drawn.
Secondly, while the paper discusses comparisons between human and AI-generated ideas and mentions statistical tests performed, it does not adequately discuss or account for potential biases or confounding factors in these comparisons. The varying 'temperatures' of the AI model could introduce unpredictable variability that is not accounted for in the analysis.
Thirdly, there is an inherent assumption that the entropy of ideas, the time needed for their generation, and their length are meaningful metrics for the quality or usefulness of these ideas. However, these assumptions are not adequately justified or supported with empirical evidence. For example, an idea that is long and complex might not necessarily be a good one.
Fourthly, the scope of the study is limited to a very specific use case, i.e., a regional development plan for Gorenjska region. This makes it hard to generalize the findings to other areas and subjects of brainstorming.
Five, there is no comparison with other AI chatbots and Large Language models such as CLAUDE, Bard and ChatGPT. Without such comparison it is difficult to evaluate the results of this study.
Lastly, the paper overlooks potential ethical and practical considerations of using AI in brainstorming and decision-making processes. For example, the potential misuse of AI in these contexts, the transparency and explainability of AI-generated ideas, and the possibility of AI dominating or biasing the brainstorming process are not adequately addressed.
In conclusion, while the paper presents a novel approach to brainstorming using AI, significant limitations in its methodology, scope, and consideration of relevant factors undermine its conclusions and applicability, it is currently immature and presents only the results of work in progress.. Future studies should aim to address these limitations, provide clearer methodologies for idea selection, and consider broader and more diverse contexts for brainstorming.
Author Response
Please see the attachment.
Author Response File: Author Response.pdf
Reviewer 2 Report
The authors proposed an approach for using one of the ChatGPT's machine learning models as a tool for injecting new ideas into the human brainstorming process. The authors presented the experimental results, mainly focused on investigating the influence of parameter values on the quality of obtained ideas. The proposed approach is not compared experimentally with other decision-making approaches. Furthermore, there are no analyses of other approaches. The authors have focused only on trendy ChatGPT but have not considered any research on collective intelligence decision-making. Please address the following issues: 1. The paper practically does not have a related research review. Please add such a section and review the related research works, for example, the works on collective intelligence decision-making. 2. How does your approach relate to collective intelligence decision-making approaches? 3. Can your approach be integrated with the collective intelligence approaches? 4. Please add a list of original contributions in the Introduction section. 5. Please provide the details of the machine learning model used and its configuration. 6. Please analyze the weak points of the proposed approach. 7. Please provide analysis of the explainability of your model's proposed ideas.
Please improve the English language when it comes to grammar and style.
Author Response
Please see the attachment.
Author Response File: Author Response.pdf
Round 2
Reviewer 1 Report
Figure 2: in the algorithm, how do you determine the uniqueness of idea? Present a formal definition of uniqueness and a set of criteria that can be employed.
Figure 4: source code examples should be moved to Appendix.
Figure 5: the presented interface is not in English, international readers can not understand it.
Figure 7: word cloud analysis does not contribute to the understandability of results. Instead, compare word histograms.
Some new numerical results have been presented in the revised version but without any statistical analysis and evaluation. The authors need to perform thorough statistical analysis and testing to support their claims as follows:
- For the coefficient of determination (r2) values also provide the p-values.
- Figure 11, Figure 12: use skewness and kurtosis to evaluate and compare symmetry of distributions.
- To test for exponentiality of distributions, you can use the Lillerfors test, which is designed specifically for that purpose., see: A Henze, N. and Meintanis, S.G. (2005): 'Recent and classical tests for exponentiality: a partial review with comparisons'. Metrika, vol. 61, pp. 29–45.
Author Response
Please see the attachment.
Author Response File: Author Response.pdf
Reviewer 2 Report
The authors have addressed most of the issues, so the paper can be accepted.
Author Response
We would like to thank, once again, the reviewer for all the insightful comments in the first round. We have made additional changes to the paper according to the suggestions of Reviewer 1 in the second round. With the best hopes and regards, Andrej Škraba & Franc Lavrič.
Round 3
Reviewer 1 Report
The authors need to discuss the limitations of this research and any bias in the interpretations of its results and implications.
Author Response
Please see the attachment.
Author Response File: Author Response.pdf