Machine Learning for Imaging-Based Cancer Diagnostics
A special issue of Cancers (ISSN 2072-6694). This special issue belongs to the section "Cancer Causes, Screening and Diagnosis".
Deadline for manuscript submissions: closed (23 February 2023) | Viewed by 7500
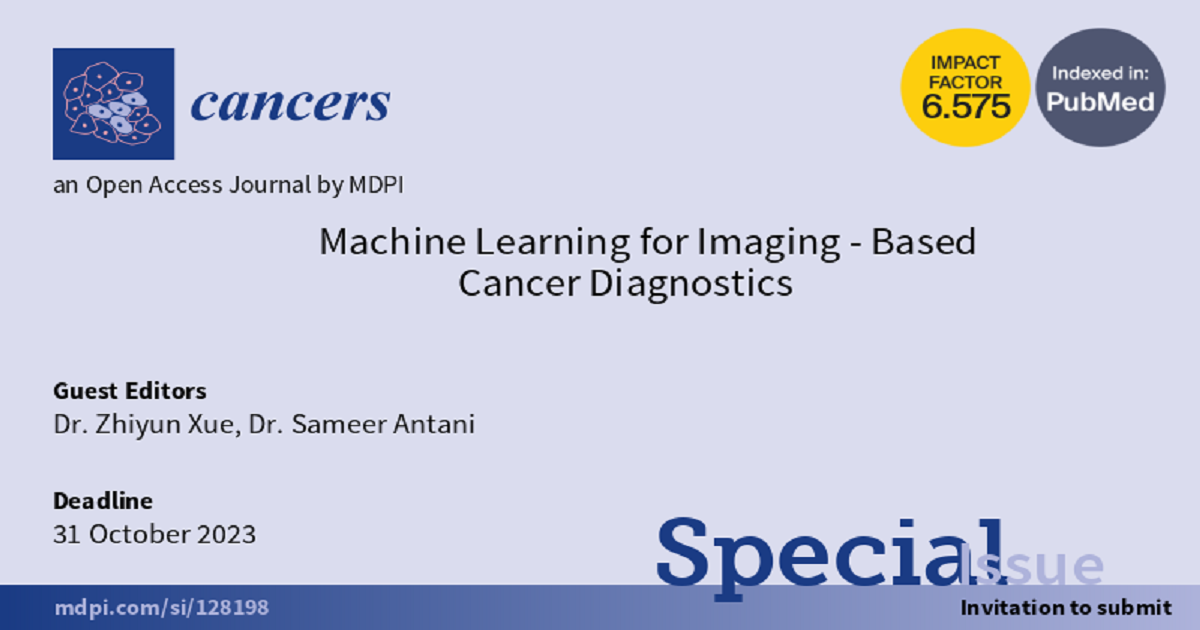
Special Issue Editors
Interests: cancers; machine learning; artificial intelligence; image processing; computer vision; biomedical informatics; data science
Special Issues, Collections and Topics in MDPI journals
Interests: machine learning; artificial intelligence; medical image analysis; image informatics; multimodal data analysis; data science; NCI (cervical cancer)
Special Issues, Collections and Topics in MDPI journals
Special Issue Information
Dear Colleagues,
Medical imaging has been ubiquitously used in clinical point-of-care and plays an important role in cancer screening, diagnosis, and treatment. There are many types of medical imaging modalities, for example, MRI, CT, X-ray, PET, mammography, ultrasound, colposcopy, microscopy, endoscopy, and fluoroscopy. Innovations in machine learning and computer-aided techniques to medical imaging in combination with various omics applications can provide valuable information to clinicians to aid in decision-making as well as improve the quality and efficiency of medical care. Although promising results have been demonstrated in the literature, especially with deep learning techniques, a great deal of work and effort remain to further advance this research field. Progress has been limited by the lack of sufficiently large and diverse datasets —especially those enriched by multiple expert annotations, relatively high intra- and inter-observer variance in annotations, inadequately defined truth standards, poor correlation in annotations among data collected at different sources, and noisy labels. Further, advances are also limited by poor image quality control, insufficiently meaningful and usable AI model explanations that adversely impact clinical interpretation of machine predictions, inadequate integration with non-imaging biomarkers, and data imbalance due to the varying prevalence of cases, among others. Through this Special Issue, we aim to highlight advances in machine learning and artificial intelligence methods that address or overcome some of the listed challenges and limitations toward improving the state of the art in image-based cancer diagnostics, treatment, and predictive risk assessment.
We are pleased to invite you to submit your related original research articles and reviews to this Special Issue, “Machine learning for imaging-based cancer diagnostics”, of the open-access MDPI journal Cancers (impact factor: 6.639). This Special Issue aims to promote and advance machine learning techniques in medical applications, especially regarding the use of intelligent medical imaging to aid the diagnostics, therapeutics, and predictive risk assessment of cancers of various organs. Research areas may include (but are not limited to) the following:
- Computer-aided diagnosis;
- Medical image analysis;
- Medical image reconstruction;
- Medical image registration;
- Medical image enhancement;
- Medical image segmentation;
- Medical image classification;
- Medical image fusion;
- Medical image retrieval;
- Image-guided interventions and surgery;
- Network interpretability and explainability for medical applications;
- Visualization in medical imaging;
- Automatic medical data cleaning;
- Statistical pattern analysis for medical applications;
- Multi-model medical data analysis;
- Medical data bias mitigation;
- Multiomics with imaging applications for cancer diagnostics and therapeutics.
We look forward to receiving your contributions.
You may choose our Joint Special Issue in Current Oncology.
Dr. Zhiyun Xue
Dr. Sameer Antani
Guest Editors
Manuscript Submission Information
Manuscripts should be submitted online at www.mdpi.com by registering and logging in to this website. Once you are registered, click here to go to the submission form. Manuscripts can be submitted until the deadline. All submissions that pass pre-check are peer-reviewed. Accepted papers will be published continuously in the journal (as soon as accepted) and will be listed together on the special issue website. Research articles, review articles as well as short communications are invited. For planned papers, a title and short abstract (about 100 words) can be sent to the Editorial Office for announcement on this website.
Submitted manuscripts should not have been published previously, nor be under consideration for publication elsewhere (except conference proceedings papers). All manuscripts are thoroughly refereed through a single-blind peer-review process. A guide for authors and other relevant information for submission of manuscripts is available on the Instructions for Authors page. Cancers is an international peer-reviewed open access semimonthly journal published by MDPI.
Please visit the Instructions for Authors page before submitting a manuscript. The Article Processing Charge (APC) for publication in this open access journal is 2900 CHF (Swiss Francs). Submitted papers should be well formatted and use good English. Authors may use MDPI's English editing service prior to publication or during author revisions.
Keywords
- machine learning
- deep learning
- cancer diagnosis
- medical image analysis
- enhancement
- segmentation
- registration
- classification
- network explanation