Applied Artificial Intelligence in Digital Horticulture: Practices and Innovations
A special issue of Horticulturae (ISSN 2311-7524). This special issue belongs to the section "Postharvest Biology, Quality, Safety, and Technology".
Deadline for manuscript submissions: 5 December 2024 | Viewed by 1396
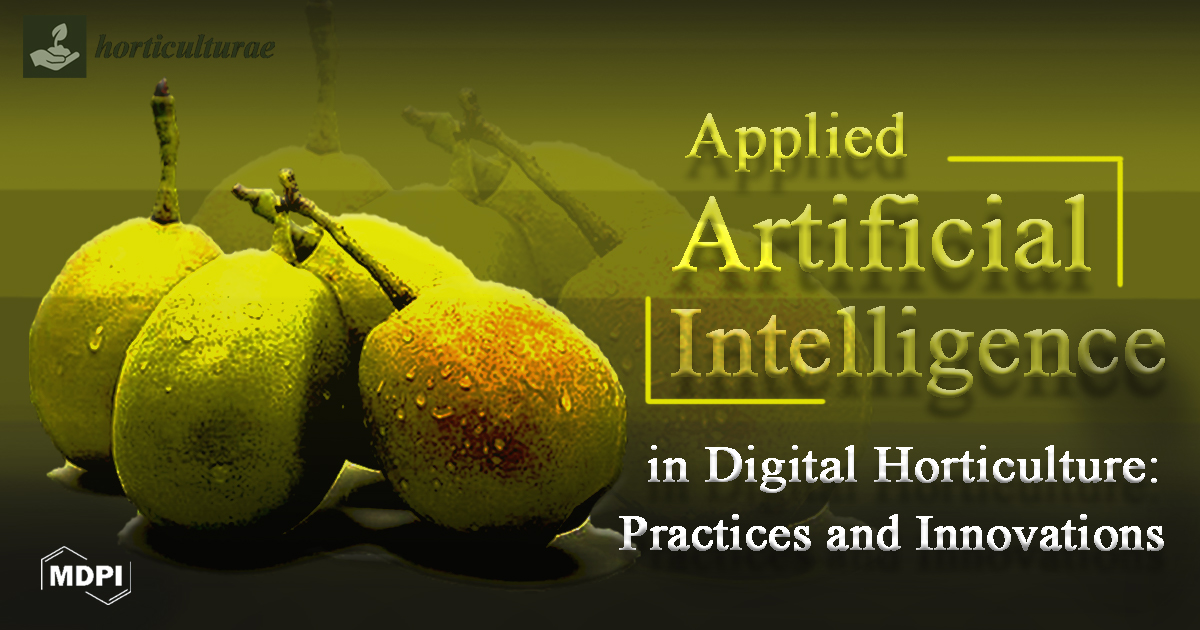
Special Issue Editors
Interests: sustainable agriculture; fruit quality; non-destructive detection; machine learning
Interests: intelligent agriculture; post-harvest; horticulture; nut
Interests: sustainable agriculture; post-harvest; fruit ripening; non-destructive detection
Interests: water erosion; sediment transport; hydrology; environmental modeling; numer-ical methods; water resources
Special Issues, Collections and Topics in MDPI journals
Special Issue Information
Dear Colleagues,
In the development of modern agriculture, artificial intelligence has achieved initial results in the field of horticulture, and the progress of its research and application continues to promote the development of precision agriculture. The integrated application of artificial intelligence has involved many links such as monitoring, forecasting, decision-making and execution, forming an intelligent monitoring and management system. Although the application of artificial intelligence in digital gardening is promising, the challenges cannot be ignored.
This Special Issue titled "Applied Artificial Intelligence in Digital Horticulture: Practices and Innovations" focuses on exploring the application and innovation of artificial intelligence technology in the field of digital horticulture, aiming to promote the progress and sustainable development of agricultural science and technology. This Special Issue introduces the technological innovations of artificial intelligence in plant growth monitoring, automatic management, precision irrigation, non-destructive testing and evaluation of fruit and vegetable quality, intelligent perception control systems and cloud platform construction. The journal encourages original research across disciplines, with a particular emphasis on research methods that combine theory and practice. Scholars from all walks of life are welcome to contribute to promote the development of artificial intelligence in digital horticulture, in order to achieve an efficient and intelligent agricultural production system.
Dr. Yang Liu
Prof. Hong Zhang
Prof. Dr. Haipeng Lan
Dr. Silvio José Gumiere
Guest Editors
Manuscript Submission Information
Manuscripts should be submitted online at www.mdpi.com by registering and logging in to this website. Once you are registered, click here to go to the submission form. Manuscripts can be submitted until the deadline. All submissions that pass pre-check are peer-reviewed. Accepted papers will be published continuously in the journal (as soon as accepted) and will be listed together on the special issue website. Research articles, review articles as well as short communications are invited. For planned papers, a title and short abstract (about 100 words) can be sent to the Editorial Office for announcement on this website.
Submitted manuscripts should not have been published previously, nor be under consideration for publication elsewhere (except conference proceedings papers). All manuscripts are thoroughly refereed through a single-blind peer-review process. A guide for authors and other relevant information for submission of manuscripts is available on the Instructions for Authors page. Horticulturae is an international peer-reviewed open access monthly journal published by MDPI.
Please visit the Instructions for Authors page before submitting a manuscript. The Article Processing Charge (APC) for publication in this open access journal is 2200 CHF (Swiss Francs). Submitted papers should be well formatted and use good English. Authors may use MDPI's English editing service prior to publication or during author revisions.
Keywords
- artificial intelligence
- digital gardening
- automated management
- non-destructive testing
- intelligent perception
Benefits of Publishing in a Special Issue
- Ease of navigation: Grouping papers by topic helps scholars navigate broad scope journals more efficiently.
- Greater discoverability: Special Issues support the reach and impact of scientific research. Articles in Special Issues are more discoverable and cited more frequently.
- Expansion of research network: Special Issues facilitate connections among authors, fostering scientific collaborations.
- External promotion: Articles in Special Issues are often promoted through the journal's social media, increasing their visibility.
- e-Book format: Special Issues with more than 10 articles can be published as dedicated e-books, ensuring wide and rapid dissemination.
Further information on MDPI's Special Issue polices can be found here.