Journal Description
Journal of Sensor and Actuator Networks
Journal of Sensor and Actuator Networks
is an international, peer-reviewed, open access journal on the science and technology of sensor and actuator networks, published bimonthly online by MDPI.
- Open Access— free for readers, with article processing charges (APC) paid by authors or their institutions.
- High Visibility: indexed within Scopus, ESCI (Web of Science), dblp, Inspec, and other databases.
- Journal Rank: JCR - Q2 (Computer Science, Information Systems) / CiteScore - Q1 (Control and Optimization)
- Rapid Publication: manuscripts are peer-reviewed and a first decision is provided to authors approximately 19 days after submission; acceptance to publication is undertaken in 5.6 days (median values for papers published in this journal in the second half of 2024).
- Recognition of Reviewers: reviewers who provide timely, thorough peer-review reports receive vouchers entitling them to a discount on the APC of their next publication in any MDPI journal, in appreciation of the work done.
Impact Factor:
3.3 (2023);
5-Year Impact Factor:
3.2 (2023)
Latest Articles
Socio-Organisational Challenges and Impacts of IoT: A Review in Healthcare and Banking
J. Sens. Actuator Netw. 2025, 14(3), 46; https://doi.org/10.3390/jsan14030046 - 24 Apr 2025
Abstract
►
Show Figures
The Internet of Things (IoT) is transforming how organisations and individuals connect and interact with digital ecosystems, especially in sectors like healthcare and banking. While technological benefits have been widely discussed, the societal and organisational impacts of IoT adoption remain underexplored. This study
[...] Read more.
The Internet of Things (IoT) is transforming how organisations and individuals connect and interact with digital ecosystems, especially in sectors like healthcare and banking. While technological benefits have been widely discussed, the societal and organisational impacts of IoT adoption remain underexplored. This study aims to address this gap by conducting a systematic literature review (SLR) of 110 peer-reviewed publications from 2012 to 2024 across four major academic databases. The review identifies and categorises the key applications of IoT, its social and organisational drivers, and the challenges of its implementation within the healthcare and banking sectors. The analysis reveals that critical barriers to IoT adoption include security, privacy, interoperability, and legal compliance, alongside concerns around workforce displacement and trust. This study also introduces the 5Cs framework—connectivity, continuity, compliance, coexistence, and cybersecurity—as a practical lens for addressing these challenges. The findings highlight the need for responsible IoT integration that balances innovation with ethical, social, and organisational accountability. Implications of this research inform policymakers, practitioners, and researchers on how to design human-centric and socially sustainable IoT strategies in sensitive sectors.
Full article
Open AccessArticle
Coherence Analysis for Vibration Monitoring Under High Variability Conditions: Constraints for Cultural Heritage Preventive Conservation
by
Claudia Pirrotta, Anna Maria Gueli, Carlo Trigona and Sebastiano Imposa
J. Sens. Actuator Netw. 2025, 14(2), 45; https://doi.org/10.3390/jsan14020045 - 21 Apr 2025
Abstract
The development of reliable sensor networks for vibration monitoring is essential for the preventive conservation of buildings and structures. The identification of natural frequencies is crucial both for sensor network planning, to ensure optimal placement, and for operation, to detect frequency shifts that
[...] Read more.
The development of reliable sensor networks for vibration monitoring is essential for the preventive conservation of buildings and structures. The identification of natural frequencies is crucial both for sensor network planning, to ensure optimal placement, and for operation, to detect frequency shifts that may indicate structural damage. However, traditional frequency detection methods, such as peak picking of the Spectrum or Power Spectral Density (PSD), are highly dependent on structural and environmental conditions. In highly variable vibrational environments, such as cultural heritage sites, stadiums, and transportation hubs, these methods often prove inadequate, leading to false modal identification. This study applies coherence analysis to vibrational measurements as a more reliable alternative that overcomes the limitations of traditional frequency extraction techniques. To evaluate its effectiveness, Magnitude-Squared Coherence (MSC), Squared Cross-Spectrum (SCS), and Wavelet Coherence (WC) were tested and compared with PSD analysis. Vibrational data were collected from a sensor network deployed at the Civil Museum of Castello Ursino (Catania, Italy), a site characterized by high structural complexity and variable visitor-induced vibrations. Results demonstrate that coherence analysis surpasses the limitations of traditional frequency identification techniques, with SCS and WC outperforming MSC in distinguishing resonance frequencies and providing a more stable and reliable frequency estimation. This approach enhances sensor network design by improving frequency detection, ensuring data reliability, and supporting long-term monitoring through instrumental drift detection, thus strengthening structural health monitoring in heritage sites.
Full article
(This article belongs to the Section Actuators, Sensors and Devices)
►▼
Show Figures
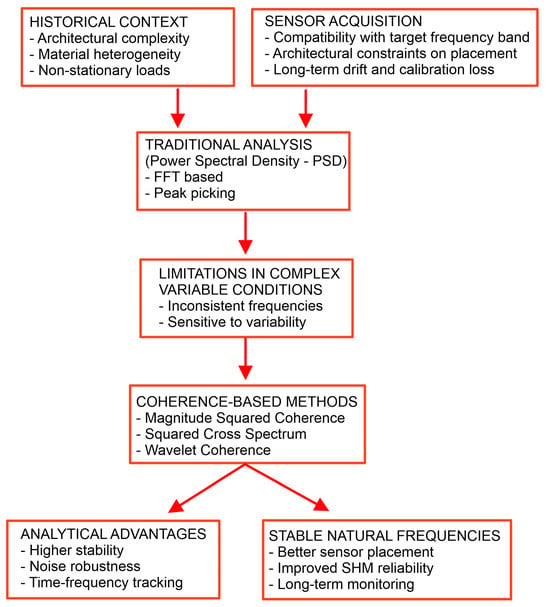
Figure 1
Open AccessArticle
Advanced Diagnosis of Cardiac and Respiratory Diseases from Chest X-Ray Imagery Using Deep Learning Ensembles
by
Hemal Nakrani, Essa Q. Shahra, Shadi Basurra, Rasheed Mohammad, Edlira Vakaj and Waheb A. Jabbar
J. Sens. Actuator Netw. 2025, 14(2), 44; https://doi.org/10.3390/jsan14020044 - 18 Apr 2025
Abstract
►▼
Show Figures
Chest X-ray interpretation is essential for diagnosing cardiac and respiratory diseases. This study introduces a deep learning ensemble approach that integrates Convolutional Neural Networks (CNNs), including ResNet-152, VGG19, EfficientNet, and a Vision Transformer (ViT), to enhance diagnostic accuracy. Using the NIH Chest X-ray
[...] Read more.
Chest X-ray interpretation is essential for diagnosing cardiac and respiratory diseases. This study introduces a deep learning ensemble approach that integrates Convolutional Neural Networks (CNNs), including ResNet-152, VGG19, EfficientNet, and a Vision Transformer (ViT), to enhance diagnostic accuracy. Using the NIH Chest X-ray dataset, the methodology involved comprehensive preprocessing, data augmentation, and model optimization techniques to address challenges such as label imbalance and feature variability. Among the individual models, VGG19 exhibited strong performance with a Hamming Loss of 0.1335 and high accuracy in detecting Edema, while ViT excelled in classifying certain conditions like Hernia. Despite the strengths of individual models, the ensemble meta-model achieved the best overall performance, with a Hamming Loss of 0.1408 and consistently higher ROC-AUC values across multiple diseases, demonstrating its superior capability to handle complex classification tasks. This robust ensemble learning framework underscores its potential for reliable and precise disease detection, offering significant improvements over traditional methods. The findings highlight the value of integrating diverse model architectures to address the complexities of multi-label chest X-ray classification, providing a pathway for more accurate, scalable, and accessible diagnostic tools in clinical practice.
Full article
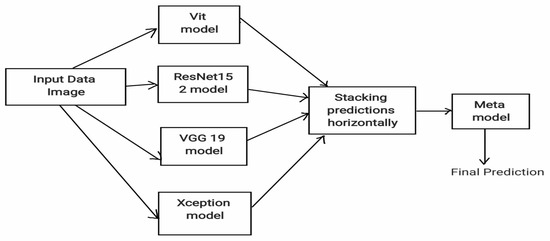
Figure 1
Open AccessArticle
Priority-Based Data Flow Control for Long-Range Wide Area Networks in Internet of Military Things
by
Rachel Kufakunesu, Herman C. Myburgh and Allan De Freitas
J. Sens. Actuator Netw. 2025, 14(2), 43; https://doi.org/10.3390/jsan14020043 - 16 Apr 2025
Abstract
The Internet of Military Things (IoMT) is transforming defense operations by enabling the seamless integration of sensors and actuators for the real-time transmission of critical data in diverse military environments. End devices (EDs) collect essential information, including troop locations, health metrics, equipment status,
[...] Read more.
The Internet of Military Things (IoMT) is transforming defense operations by enabling the seamless integration of sensors and actuators for the real-time transmission of critical data in diverse military environments. End devices (EDs) collect essential information, including troop locations, health metrics, equipment status, and environmental conditions, which are processed to enhance situational awareness and operational efficiency. In scenarios involving large-scale deployments across remote or austere regions, wired communication systems are often impractical and cost-prohibitive. Wireless sensor networks (WSNs) provide a cost-effective alternative, with Long-Range Wide Area Network (LoRaWAN) emerging as a leading protocol due to its extensive coverage, low energy consumption, and reliability. Existing LoRaWAN network simulation modules, such as those in ns-3, primarily support uniform periodic data transmissions, limiting their applicability in critical military and healthcare contexts that demand adaptive transmission rates, resource optimization, and prioritized data delivery. These limitations are particularly pronounced in healthcare monitoring, where frequent, high-rate data transmission is vital but can strain the network’s capacity. To address these challenges, we developed an enhanced sensor data sender application capable of simulating priority-based traffic within LoRaWAN, specifically targeting use cases like border security and healthcare monitoring. This study presents a priority-based data flow control protocol designed to optimize network performance under high-rate healthcare data conditions while maintaining overall system reliability. Simulation results demonstrate that the proposed protocol effectively mitigates performance bottlenecks, ensuring robust and energy-efficient communication in critical IoMT applications within austere environments.
Full article
(This article belongs to the Special Issue Security and Smart Applications in IoT and Wireless Sensor and Actuator Networks)
►▼
Show Figures
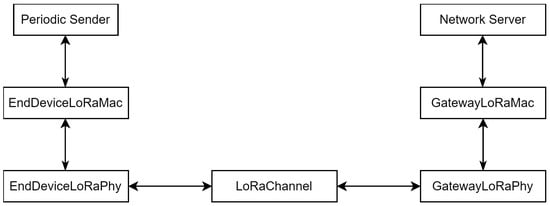
Figure 1
Open AccessArticle
Enhancing Sensor-Based Human Physical Activity Recognition Using Deep Neural Networks
by
Minyar Sassi Hidri, Adel Hidri, Suleiman Ali Alsaif, Muteeb Alahmari and Eman AlShehri
J. Sens. Actuator Netw. 2025, 14(2), 42; https://doi.org/10.3390/jsan14020042 - 14 Apr 2025
Abstract
►▼
Show Figures
Human activity recognition (HAR) is the task of classifying sequences of data into defined movements. Taking advantage of deep learning (DL) methods, this research investigates and optimizes neural network architectures to effectively classify physical activities from smartphone accelerometer data. Unlike traditional machine learning
[...] Read more.
Human activity recognition (HAR) is the task of classifying sequences of data into defined movements. Taking advantage of deep learning (DL) methods, this research investigates and optimizes neural network architectures to effectively classify physical activities from smartphone accelerometer data. Unlike traditional machine learning (ML) methods employing manually crafted features, our approach employs automated feature learning with three deep learning architectures: Convolutional Neural Networks (CNN), CNN-based autoencoders, and Long Short-Term Memory Recurrent Neural Networks (LSTM RNN). The contribution of this work is primarily in optimizing LSTM RNN to leverage the most out of temporal relationships between sensor data, significantly improving classification accuracy. Experimental outcomes for the WISDM dataset show that the proposed LSTM RNN model achieves 96.1% accuracy, outperforming CNN-based approaches and current ML-based methods. Compared to current works, our optimized frameworks achieve up to 6.4% higher classification performance, which means that they are more appropriate for real-time HAR.
Full article
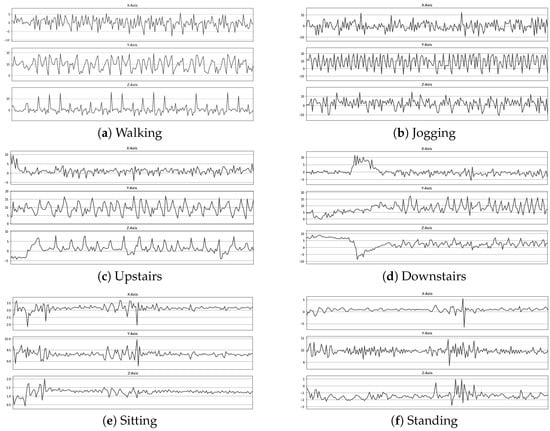
Figure 1
Open AccessArticle
Lossless Compression with Trie-Based Shared Dictionary for Omics Data in Edge–Cloud Frameworks
by
Rani Adam, Daniel R. Catchpoole, Simeon J. Simoff, Zhonglin Qu, Paul J. Kennedy and Quang Vinh Nguyen
J. Sens. Actuator Netw. 2025, 14(2), 41; https://doi.org/10.3390/jsan14020041 - 9 Apr 2025
Abstract
►▼
Show Figures
The growing complexity and volume of genomic and omics data present critical challenges for storage, transfer, and analysis in edge–cloud platforms. Existing compression techniques often involve trade-offs between efficiency and speed, requiring innovative approaches that ensure scalability and cost-effectiveness. This paper introduces a
[...] Read more.
The growing complexity and volume of genomic and omics data present critical challenges for storage, transfer, and analysis in edge–cloud platforms. Existing compression techniques often involve trade-offs between efficiency and speed, requiring innovative approaches that ensure scalability and cost-effectiveness. This paper introduces a lossless compression method that integrates Trie-based shared dictionaries within an edge–cloud architecture. It presents a software-centric scientific research process of the design and evaluation of the proposed compression method. By enabling localized preprocessing at the edge, our approach reduces data redundancy before cloud transmission, thereby optimizing both storage and network efficiency. A global shared dictionary is constructed using N-gram analysis to identify and prioritize repeated sequences across multiple files. A lightweight index derived from this dictionary is then pushed to edge nodes, where Trie-based sequence replacement is applied to eliminate redundancy locally. The preprocessed data are subsequently transmitted to the cloud, where advanced compression algorithms, such as Zstd, GZIP, Snappy, and LZ4, further compress them. Evaluation on real patient omics datasets from B-cell Acute Lymphoblastic Leukemia (B-ALL) and Chronic Lymphocytic Leukemia (CLL) demonstrates that edge preprocessing significantly improves compression ratios, reduces upload times, and enhances scalability in hybrid cloud frameworks.
Full article
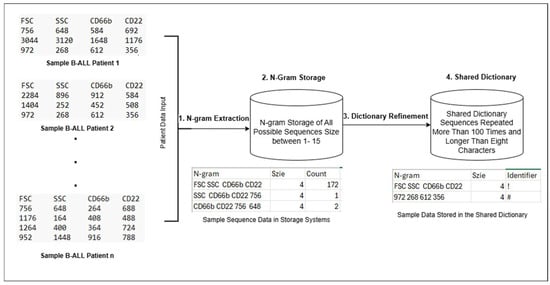
Figure 1
Open AccessArticle
Deepfake Image Classification Using Decision (Binary) Tree Deep Learning
by
Mariam Alrajeh and Aida Al-Samawi
J. Sens. Actuator Netw. 2025, 14(2), 40; https://doi.org/10.3390/jsan14020040 - 8 Apr 2025
Abstract
The unprecedented rise of deepfake technologies, leveraging sophisticated AI like Generative Adversarial Networks (GANs) and diffusion-based models, presents both opportunities and challenges in terms of digital media authenticity. In response, this study introduces a novel deep neural network ensemble that utilizes a tree-based
[...] Read more.
The unprecedented rise of deepfake technologies, leveraging sophisticated AI like Generative Adversarial Networks (GANs) and diffusion-based models, presents both opportunities and challenges in terms of digital media authenticity. In response, this study introduces a novel deep neural network ensemble that utilizes a tree-based hierarchical architecture integrating a vision transformer, ResNet, EfficientNet, and DenseNet to address the pressing need for effective deepfake detection. Our model exhibits a high degree of adaptability across varied datasets and demonstrates state-of-the-art performance, achieving up to 97.25% accuracy and a weighted F1 score of 97.28%. By combining the strengths of various convolutional networks and the vision transformer, our approach underscores a scalable solution for mitigating the risks associated with manipulated media.
Full article
(This article belongs to the Section Network Security and Privacy)
►▼
Show Figures
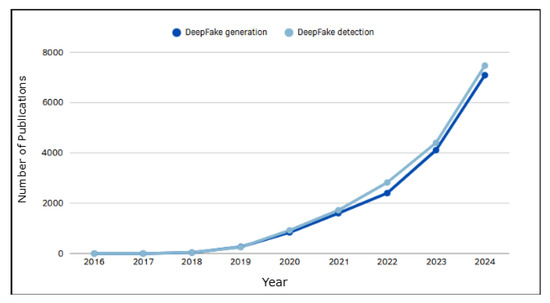
Figure 1
Open AccessReview
Urban Air Mobility, Personal Drones, and the Safety of Occupants—A Comprehensive Review
by
Dmytro Zhyriakov, Mariusz Ptak and Marek Sawicki
J. Sens. Actuator Netw. 2025, 14(2), 39; https://doi.org/10.3390/jsan14020039 - 6 Apr 2025
Abstract
Urban air mobility (UAM) is expected to provide environmental benefits while enhancing transportation for citizens and businesses, particularly in commercial and emergency medical applications. The rapid development of electric vertical take-off and landing (eVTOL) aircraft has demonstrated the potential to introduce new technological
[...] Read more.
Urban air mobility (UAM) is expected to provide environmental benefits while enhancing transportation for citizens and businesses, particularly in commercial and emergency medical applications. The rapid development of electric vertical take-off and landing (eVTOL) aircraft has demonstrated the potential to introduce new technological capabilities to the market, fostering visions of widespread and diverse UAM applications. This paper reviews state-of-the-art occupant safety for personal drones and examines existing occupant protection methods in the aircraft. The study serves as a guide for stakeholders, including regulators, manufacturers, researchers, policymakers, and industry professionals—by providing insights into the regulatory landscape and safety assurance frameworks for eVTOL aircraft in UAM applications. Furthermore, we present a functional hazard assessment (FHA) conducted on a reference concept, detailing the process, decision-making considerations, and key variations. The analysis illustrates the FHA methodology while discussing the trade-offs involved in safety evaluations. Additionally, we provide a summary and a featured description of current eVTOL aircraft, highlighting their key characteristics and technological advancements.
Full article
(This article belongs to the Special Issue Advances in Intelligent Transportation Systems (ITS))
►▼
Show Figures
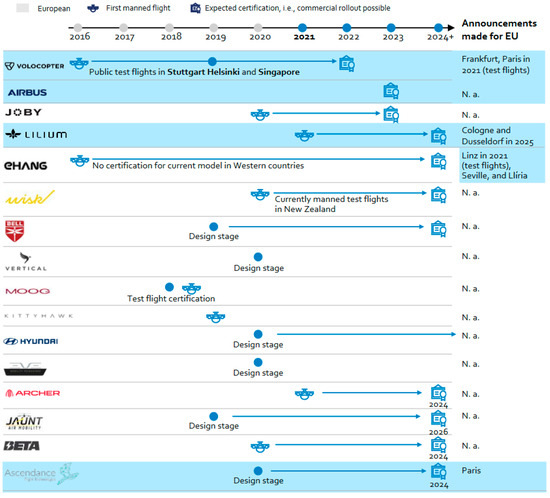
Figure 1
Open AccessArticle
An Efficient Framework for Peer Selection in Dynamic P2P Network Using Q Learning with Fuzzy Linear Programming
by
Mahalingam Anandaraj, Tahani Albalawi and Mohammad Alkhatib
J. Sens. Actuator Netw. 2025, 14(2), 38; https://doi.org/10.3390/jsan14020038 - 2 Apr 2025
Abstract
►▼
Show Figures
This paper proposes a new approach to integrating Q learning into the fuzzy linear programming (FLP) paradigm to improve peer selection in P2P networks. Using Q learning, the proposed method employs real-time feedback to adjust and update peer selection policies. The FLP framework
[...] Read more.
This paper proposes a new approach to integrating Q learning into the fuzzy linear programming (FLP) paradigm to improve peer selection in P2P networks. Using Q learning, the proposed method employs real-time feedback to adjust and update peer selection policies. The FLP framework enriches this process by dealing with imprecise information through fuzzy logic. It is used to achieve multiple objectives, such as enhancing the throughput rate, reducing the delay, and guaranteeing a reliable connection. This integration effectively solves the problem of network uncertainty, making the network configuration more stable and flexible. It is also important to note that throughout the use of the Q-learning agent in the network, various state metric indicators, including available bandwidth, latency, packet drop rates, and connectivity of nodes, are observed and recorded. It then selects actions by choosing optimal peers for each node and updating a Q table that defines states and actions based on these performance indices. This reward system guides the agent’s learning, refining its peer selection policy over time. The FLP framework supports the Q-learning agent by providing optimized solutions that balance conflicting objectives under uncertain conditions. Fuzzy parameters capture variability in network metrics, and the FLP model solves a fuzzy linear programming problem, offering guidelines for the Q-learning agent’s decisions. The proposed method is evaluated under different experimental settings to reveal its effectiveness. The Erdos–Renyi model simulation is used, and it shows that throughput increased by 21% and latency decreased by 40%. The computational efficiency was also notably improved, with computation times diminishing by up to five orders of magnitude compared to traditional methods.
Full article
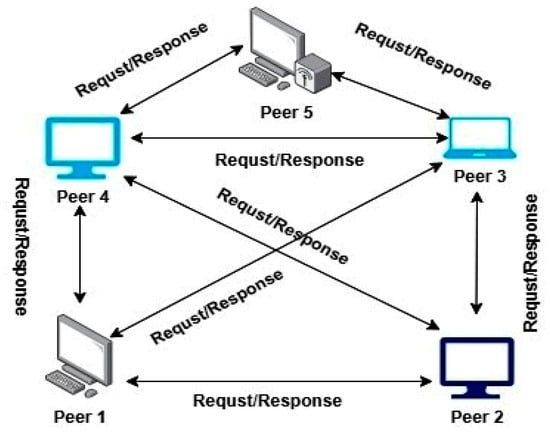
Figure 1
Open AccessArticle
Heterogeneity Challenges of Federated Learning for Future Wireless Communication Networks
by
Lorena Isabel Barona López and Thomás Borja Saltos
J. Sens. Actuator Netw. 2025, 14(2), 37; https://doi.org/10.3390/jsan14020037 - 1 Apr 2025
Abstract
Two technologies of great interest in recent years—Artificial Intelligence (AI) and massive wireless communication networks—have found a significant point of convergence through Federated Learning (FL). Federated Learning is a Machine Learning (ML) technique that enables multiple participants to collaboratively train a model while
[...] Read more.
Two technologies of great interest in recent years—Artificial Intelligence (AI) and massive wireless communication networks—have found a significant point of convergence through Federated Learning (FL). Federated Learning is a Machine Learning (ML) technique that enables multiple participants to collaboratively train a model while keeping their data local. Several studies indicate that while improving performance metrics—such as accuracy, loss reduction, or computation time—is a primary goal, achieving this in real-world scenarios remains challenging. This difficulty arises due to various heterogeneity characteristics inherent to the wireless devices participating in the Federation. Heterogeneity in Federated Learning arises when participants contribute differently, leading to challenges in the model training process. Heterogeneity in Federated Learning may appear in architecture, statistics, and behavior. System heterogeneity arises from differences in device capabilities, including processing power, transmission speeds, availability, energy constraints, and network limitations, among others. Statistical heterogeneity occurs when participants contribute non-independent and non-identically distributed (non-IID) data. This situation can harm the global model instead of improving it, especially when the data are of poor quality or too scarce. The third type, behavioral heterogeneity, refers to cases where participants are unwilling to engage or expect rewards despite minimal effort. Given the growing research in this area, we present a summary of heterogeneity characteristics in Federated Learning to provide a broader perspective on this emerging technology. We also outline key challenges, opportunities, and future directions for Federated Learning. Finally, we conduct a simulation using the LEAF framework to illustrate the impact of heterogeneity in Federated Learning.
Full article
(This article belongs to the Special Issue Federated Learning: Applications and Future Directions)
►▼
Show Figures
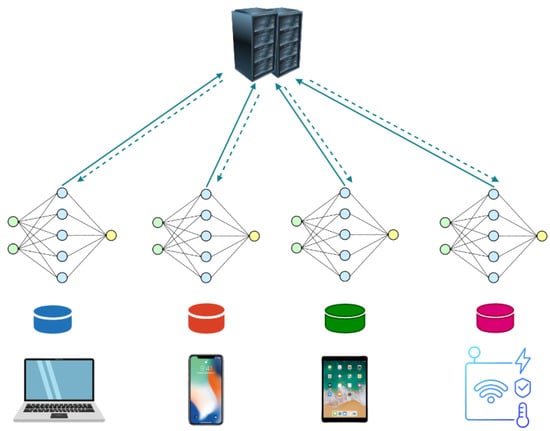
Figure 1
Open AccessArticle
Lower-Complexity Multi-Layered Security Partitioning Algorithm Based on Chaos Mapping-DWT Transform for WA/SNs
by
Tarek Srour, Mohsen A. M. El-Bendary, Mostafa Eltokhy, Atef E. Abouelazm, Ahmed A. F. Youssef and Ali M. El-Rifaie
J. Sens. Actuator Netw. 2025, 14(2), 36; https://doi.org/10.3390/jsan14020036 - 31 Mar 2025
Abstract
The resource limitations of Low-Power Wireless Networks (LP-WNs), such as Wireless Sensor Networks (WSNs), Wireless Actuator/Sensor Networks (WA/SNs), and Internet of Things (IoT) outdoor applications, restrict the utilization of the error-performance-enhancing techniques and the use of the powerful and robust security tools. Therefore,
[...] Read more.
The resource limitations of Low-Power Wireless Networks (LP-WNs), such as Wireless Sensor Networks (WSNs), Wireless Actuator/Sensor Networks (WA/SNs), and Internet of Things (IoT) outdoor applications, restrict the utilization of the error-performance-enhancing techniques and the use of the powerful and robust security tools. Therefore, these LP-WN applications require special techniques to satisfy the requirements of a low data loss rate and satisfy the security requirements while considering the accepted level of complexity and power efficiency of these techniques. This paper focuses on proposing a power-efficient, robust cryptographic algorithm for the WA/SNs. The lower-complexity cryptographic algorithm is proposed, based on merging the data composition tools utilizing data transforms and chaos mapping techniques. The decomposing tool is performed by the various data transforms: Discrete Cosine Transform (DCT), Discrete Cosine Wavelet (DWT), Fast Fourier Transform (FFT), and Walsh Hadamard Transform (WHT); the DWT performs better with efficient complexity. It is utilized to separate the plaintext into the main portion and side information portions to reduce more than 50% of complexity. The main plaintext portion is ciphered in the series of cryptography to reduce the complexity and increase the security capabilities of the proposed algorithm by two chaos mappings. The process of reduction saves complexity and is employed to feed the series of chaos cryptography without increasing the complexity. The two chaos mappings are used, and two-dimensional (2D) chaos logistic maps are used due to their high sensitivity to noise and attacks. The chaos 2D baker map is utilized due to its high secret key managing flexibility and high sensitivity to initial conditions and plaintext dimensions. Several computer experiments are demonstrated to evaluate the robustness, reliability, and applicability of the proposed complexity-efficient crypto-system algorithm in the presence of various attacks. The results prove the high suitability of the proposed lower-complexity crypto-system for WASN and LP-WN applications due to its robustness in the presence of attacks and its power efficiency.
Full article
(This article belongs to the Special Issue Applications of Wireless Sensor Networks: Innovations and Future Trends)
►▼
Show Figures
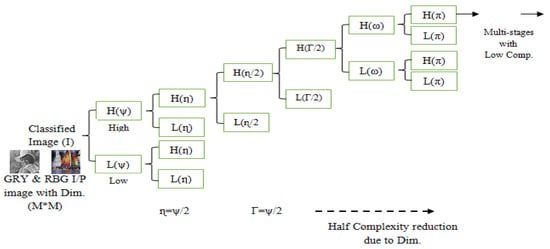
Figure 1
Open AccessArticle
A Zero-Trust Multi-Processor Reporter-Verifier Design of Edge Devices for Firmware Authenticity in Internet of Things and Blockchain Applications
by
Ananda Maiti and Alexander A. Kist
J. Sens. Actuator Netw. 2025, 14(2), 35; https://doi.org/10.3390/jsan14020035 - 31 Mar 2025
Abstract
Firmware authenticity and integrity during upgrades are critical security factors in Internet of Things (IoT) applications in the age of edge artificial intelligence (AI). Data from IoT applications are vital for business decisions. Any unintended or malicious change in data can adversely impact
[...] Read more.
Firmware authenticity and integrity during upgrades are critical security factors in Internet of Things (IoT) applications in the age of edge artificial intelligence (AI). Data from IoT applications are vital for business decisions. Any unintended or malicious change in data can adversely impact the goals of an IoT application. Several studies have focused on using blockchain to ensure the authentication of IoT devices and the integrity of data once the data are in the blockchain. Firmware upgrades on IoT edge devices have also been investigated with blockchain applications, with a focus on eliminating external threats during firmware upgrades on IoT devices. In this paper, we propose a new IoT device design that works against internal threats by preventing malicious codes from device manufacturers. In IoT applications that monitor critical data, it is important to ensure that the correct firmware reporting honest data is running on the devices. As devices are owned and operated by a small group of application stakeholders, this multiprocessor design extracts the firmware periodically and checks whether it matches the signatures of the expected firmware designed for the business goals of the IoT applications. The test results show that there is no significant increase in code, disruption, or power consumption when implementing such a device. This scheme provides a hardware-oriented solution utilizing processor-to-processor communication protocols and is an alternative to running lightweight blockchain on IoT edge devices.
Full article
(This article belongs to the Special Issue Security and Smart Applications in IoT and Wireless Sensor and Actuator Networks)
►▼
Show Figures
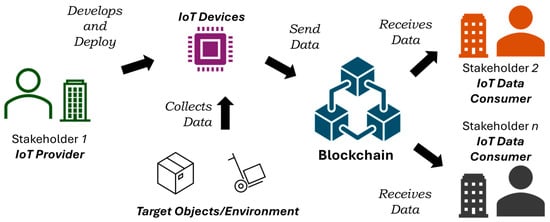
Figure 1
Open AccessArticle
Sensor Fusion Enhances Anomaly Detection in a Flood Forecasting System
by
Andrew Ma, Abhir Karande, Natalie Dahlquist, Fabien Ferrero and N. Rich Nguyen
J. Sens. Actuator Netw. 2025, 14(2), 34; https://doi.org/10.3390/jsan14020034 - 25 Mar 2025
Abstract
To build an Internet of Things (IoT) infrastructure that provides flood susceptibility forecasts for granular geographic levels, an extensive network of IoT weather sensors in local regions is crucial. However, these IoT devices may exhibit anomalistic behavior due to factors such as diminished
[...] Read more.
To build an Internet of Things (IoT) infrastructure that provides flood susceptibility forecasts for granular geographic levels, an extensive network of IoT weather sensors in local regions is crucial. However, these IoT devices may exhibit anomalistic behavior due to factors such as diminished signal strength, physical disturbance, low battery life, and more. To ensure that incorrect readings are identified and addressed appropriately, we devise a novel method for multi-stream sensor data verification and anomaly detection. Our method uses time-series anomaly detection to identify incorrect readings. We expand on the state-of-the-art by incorporating sensor fusion mechanisms between nearby devices to improve anomaly detection ability. Our system pairs nearby devices and fuses them by creating a new time series with the difference between the corresponding readings. This new time series is then input into a time-series anomaly detection model which identifies if any readings are anomalistic. By testing our system with nine different machine learning anomaly detection methods on synthetic data based on one year of real weather data, we find that our system outperforms the previous anomaly detection methods by improving F1-Score by 10.8%.
Full article
(This article belongs to the Special Issue Fault Diagnosis in the Internet of Things Applications)
►▼
Show Figures
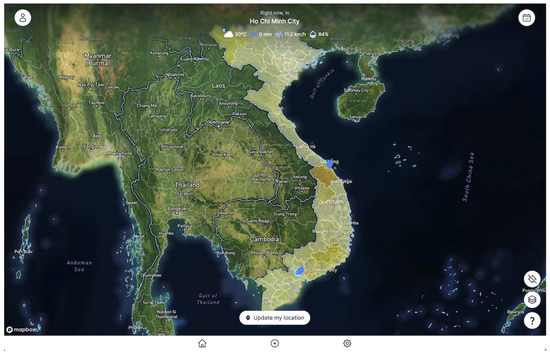
Figure 1
Open AccessArticle
Environmental Factors in Structural Health Monitoring—Analysis and Removal of Effects from Resonance Frequencies
by
Rims Janeliukstis, Lasma Ratnika, Liga Gaile and Sandris Rucevskis
J. Sens. Actuator Netw. 2025, 14(2), 33; https://doi.org/10.3390/jsan14020033 - 20 Mar 2025
Abstract
Strategically important objects, such as dams, tunnels, bridges, and others, require long-term structural health monitoring programs in order to preserve their structural integrity with minimal downtime, financial expenses, and increased safety for civilians. The current study focuses on developing a damage detection methodology
[...] Read more.
Strategically important objects, such as dams, tunnels, bridges, and others, require long-term structural health monitoring programs in order to preserve their structural integrity with minimal downtime, financial expenses, and increased safety for civilians. The current study focuses on developing a damage detection methodology that is applicable to the long-term monitoring of such structures. It is based on the identification of resonant frequencies from operational modal analysis, removing the effect of environmental factors on the resonant frequencies through support vector regression with optimized hyperparameters and, finally, classifying the global structural state as either healthy or damaged, utilizing the Mahalanobis distance. The novelty lies in two additional steps that supplement this procedure, namely, the nonlinear estimation of the relative effects of various environmental factors, such as temperature, humidity, and ambient loads on the resonant frequencies, and the selection of the most informative resonant frequency features using a non-parametric neighborhood component analysis algorithm. This methodology is validated on a wooden two-story truss structure with different artificial structural modifications that simulate damage in a non-destructive manner. It is found that, firstly, out of all environmental factors, temperature has a dominating decreasing effect on resonance frequencies, followed by humidity, wind speed, and precipitation. Secondly, the selection of only a handful of the most informative resonance frequency features not only reduces the feature space, but also increases the classification performance, albeit with a trade-off between false alarms and missed damage detection. The proposed approach effectively minimizes false alarms and ensures consistent damage detection under varying environmental conditions, offering tangible benefits for long-term SHM applications.
Full article
(This article belongs to the Special Issue Fault Diagnosis in the Internet of Things Applications)
►▼
Show Figures
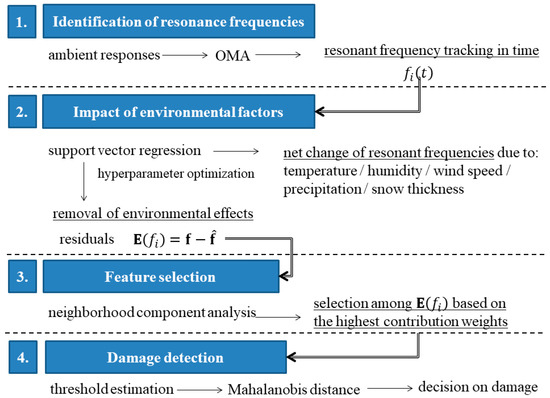
Figure 1
Open AccessArticle
Robust Distributed Collaborative Beamforming for WSANs in Dual-Hop Scattered Environments with Nominally Rectangular Layouts
by
Oussama Ben Smida, Sofiène Affes, Dushantha Jayakody and Yoosuf Nizam
J. Sens. Actuator Netw. 2025, 14(2), 32; https://doi.org/10.3390/jsan14020032 - 19 Mar 2025
Abstract
We introduce a robust distributed collaborative beamforming (RDCB) approach for addressing channel estimation challenges in dual-hop transmissions within wireless sensor and actuator networks (WSANs) of K nodes. WSANs enhance wireless communication by reducing data transmission, latency, and energy consumption while optimizing network load
[...] Read more.
We introduce a robust distributed collaborative beamforming (RDCB) approach for addressing channel estimation challenges in dual-hop transmissions within wireless sensor and actuator networks (WSANs) of K nodes. WSANs enhance wireless communication by reducing data transmission, latency, and energy consumption while optimizing network load through integrated sensing and actuation. The source S transmits signals to the WSAN, where nodes relay them to the destination D using beamforming weights to minimize noise and preserve signal integrity. These weights depend on channel state information (CSI), where estimation errors degrade performance. We develop RDCB solutions for three first-hop propagation scenarios—monochromatic [line-of-sight (LoS)] or “M”, bichromatic (moderately scattered) or “B”, and polychromatic (highly scattered) or “P”—while assuming a monochromatic LoS or “M” link for the second hop between the nodes and the far-field destination. Termed MM-RDCB, BM-RDCB, and PM-RDCB, respectively (“X” and “Y” in XY-RDCB—for X and Y —refer to the chromatic natures of the first- and second-hop channels, respectively, to which a specific RDCB solution is tailored), these solutions leverage asymptotic approximations for large K values and the nodes’ geometric symmetries. Our distributed solutions allow local weight computation, enhancing spectral and power efficiency. Simulation results show significant improvements in the signal-to-noise ratio (SNR) and robustness versus WSAN node placement errors, making the solutions well suited for emerging 5G and future 5G+/6G and Internet of Things (IoT) applications for different challenging environments.
Full article
(This article belongs to the Special Issue Applications of Wireless Sensor Networks: Innovations and Future Trends)
►▼
Show Figures
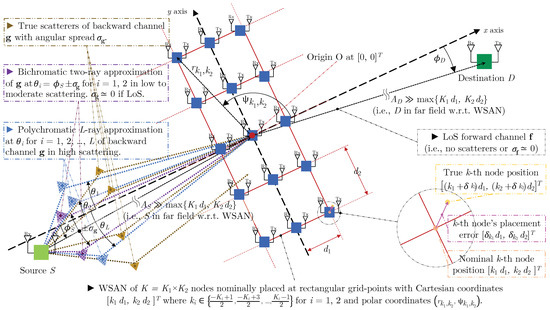
Figure 1
Open AccessArticle
Optimizing Sensor Locations for Electrodermal Activity Monitoring Using a Wearable Belt System
by
Riley Q. McNaboe, Youngsun Kong, Wendy A. Henderson, Xiaomei Cong, Aolan Li, Min-Hee Seo, Ming-Hui Chen, Bin Feng and Hugo F. Posada-Quintero
J. Sens. Actuator Netw. 2025, 14(2), 31; https://doi.org/10.3390/jsan14020031 - 18 Mar 2025
Abstract
Wearable devices for continuous health monitoring in humans are constantly evolving, yet the signal quality may be improved by optimizing electrode placement. While the commonly used locations to measure electrodermal activity (EDA) are at the fingers or the wrist, alternative locations, such as
[...] Read more.
Wearable devices for continuous health monitoring in humans are constantly evolving, yet the signal quality may be improved by optimizing electrode placement. While the commonly used locations to measure electrodermal activity (EDA) are at the fingers or the wrist, alternative locations, such as the torso, need to be considered when applying an integrated multimodal approach of concurrently recording multiple bio-signals, such as the monitoring of visceral pain symptoms like those related to irritable bowel syndrome (IBS). This study aims to quantitatively determine the EDA signal quality at four torso locations (mid-chest, upper abdomen, lower back, and mid-back) in comparison to EDA signals recorded from the fingers. Concurrent EDA signals from five body locations were collected from twenty healthy participants as they completed a Stroop Task and a Cold Pressor task that elicited salient autonomic responses. Mean skin conductance (meanSCL), non-specific skin conductance responses (NS.SCRs), and sympathetic response (TVSymp) were derived from the torso EDA signals and compared with signals from the fingers. Notably, TVSymp recorded from the mid-chest location showed significant changes between baseline and Stroop phase, consistent with the TVSymp recorded from the fingers. A high correlation (0.77–0.83) was also identified between TVSymp recorded from the fingers and three torso locations: mid-chest, upper abdomen, and lower back locations. While the fingertips remain the optimal site for EDA measurement, the mid-chest exhibited the strongest potential as an alternative recording site, with the upper abdomen and lower back also demonstrating promising results. These findings suggest that torso-based EDA measurements have the potential to provide reliable measurement of sympathetic neural activities and may be incorporated into a wearable belt system for multimodal monitoring.
Full article
(This article belongs to the Special Issue Security and Smart Applications in IoT and Wireless Sensor and Actuator Networks)
►▼
Show Figures
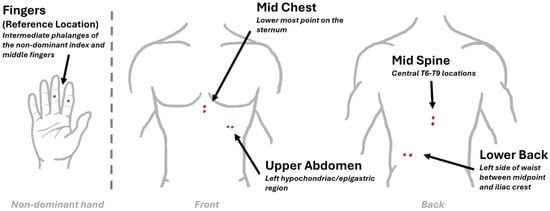
Figure 1
Open AccessReview
Key Enabling Technologies for 6G: The Role of UAVs, Terahertz Communication, and Intelligent Reconfigurable Surfaces in Shaping the Future of Wireless Networks
by
Wagdy M. Othman, Abdelhamied A. Ateya, Mohamed E. Nasr, Ammar Muthanna, Mohammed ElAffendi, Andrey Koucheryavy and Azhar A. Hamdi
J. Sens. Actuator Netw. 2025, 14(2), 30; https://doi.org/10.3390/jsan14020030 - 17 Mar 2025
Abstract
Sixth-generation (6G) wireless networks have the potential to transform global connectivity by supporting ultra-high data rates, ultra-reliable low latency communication (uRLLC), and intelligent, adaptive networking. To realize this vision, 6G must incorporate groundbreaking technologies that enhance network efficiency, spectral utilization, and dynamic adaptability.
[...] Read more.
Sixth-generation (6G) wireless networks have the potential to transform global connectivity by supporting ultra-high data rates, ultra-reliable low latency communication (uRLLC), and intelligent, adaptive networking. To realize this vision, 6G must incorporate groundbreaking technologies that enhance network efficiency, spectral utilization, and dynamic adaptability. Among them, unmanned aerial vehicles (UAVs), terahertz (THz) communication, and intelligent reconfigurable surfaces (IRSs) are three major enablers in redefining the architecture and performance of next-generation wireless systems. This survey provides a comprehensive review of these transformative technologies, exploring their potential, design challenges, and integration into future 6G ecosystems. UAV-based communication provides flexible, on-demand communication in remote, harsh areas and is a vital solution for disasters, self-driving, and industrial automation. THz communication taking place in the 0.1–10 THz band reveals ultra-high bandwidth capable of a data rate of multi-gigabits per second and can avoid spectrum bottlenecks in conventional bands. IRS technology based on programmable metasurface allows real-time wavefront control, maximizing signal propagation and spectral/energy efficiency in complex settings. The work provides architectural evolution, active current research trends, and practical issues in applying these technologies, including their potential contribution to the creation of intelligent, ultra-connected 6G networks. In addition, it presents open research questions, possible answers, and future directions and provides information for academia, industry, and policymakers.
Full article
(This article belongs to the Special Issue Applications of Wireless Sensor Networks: Innovations and Future Trends)
►▼
Show Figures
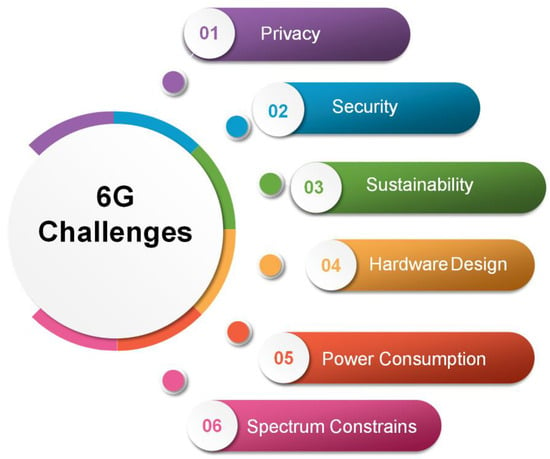
Figure 1
Open AccessArticle
Comprehensive Exploration of Limitations of Simplified Machine Learning Algorithm for Fault Diagnosis Under Fault and Ground Resistances of Multiterminal High-Voltage Direct Current System
by
Raheel Muzzammel
J. Sens. Actuator Netw. 2025, 14(2), 29; https://doi.org/10.3390/jsan14020029 - 17 Mar 2025
Abstract
►▼
Show Figures
High power density and better efficiency make the multiterminal high-voltage direct current (MT-HVDC) system the best candidate for long-distance bulk power transfer in the cases of onshore and offshore power systems. Many machine learning-based algorithms have been developed for the protection of MT-HVDC
[...] Read more.
High power density and better efficiency make the multiterminal high-voltage direct current (MT-HVDC) system the best candidate for long-distance bulk power transfer in the cases of onshore and offshore power systems. Many machine learning-based algorithms have been developed for the protection of MT-HVDC systems. However, the exploration of the effects of change in the fault and ground resistances of MT-HVDC systems has not been studied comprehensively. In this study, a four-terminal HVDC test system is employed for the analysis of the effects on fault diagnosis under change in the fault and ground resistances. A simplified medium tree-based machine learning algorithm that works on Gini’s index of diversity is developed for fault diagnosis in the MT-HVDC system. It is found from the simulation analysis that the preprocessing based on mean and differences in featured data extracted for fault current is required to reduce the impacts of the accuracy of machine learning algorithms. The preprocessing not only retains the accuracy of the machine learning algorithm in different cases of faults, but also minimizes the reduction in accuracy in some fault cases. In the test cases, the accuracy is 88.7%, 60%, and 57.1% without preprocessing of featured data for the machine learning algorithm under different values of fault and ground resistances, but the accuracy is improved to 99.5%, 84.1%, and 77.8%, respectively. Hence, the machine learning algorithm can be made applicable under different values of fault and ground resistances for the protection of the MT-HVDC system. This helps to develop a protected MT-HVDC system for long distances without the fear of different soil conditions.
Full article
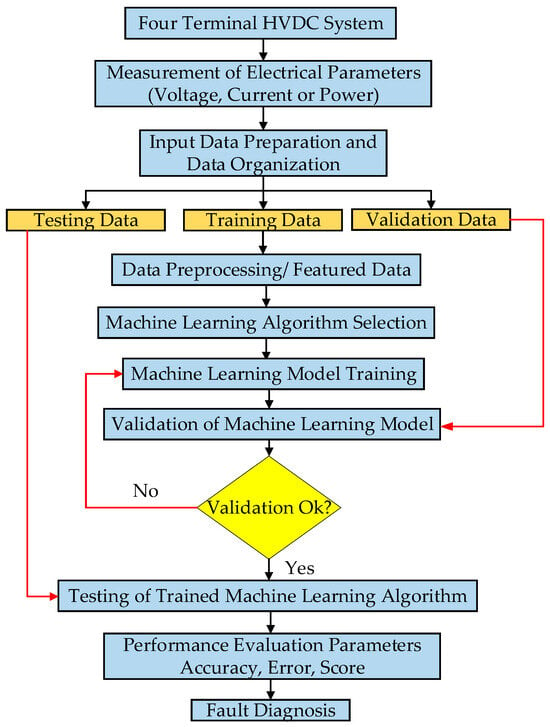
Figure 1
Open AccessReview
Compressive Sensing in Power Engineering: A Comprehensive Survey of Theory and Applications, and a Case Study
by
Lekshmi R. Chandran, Ilango Karuppasamy, Manjula G. Nair, Hongjian Sun and Parvathy Krishnan Krishnakumari
J. Sens. Actuator Netw. 2025, 14(2), 28; https://doi.org/10.3390/jsan14020028 - 7 Mar 2025
Abstract
Compressive Sensing (CS) is a transformative signal processing framework that enables sparse signal acquisition at rates below the Nyquist limit, offering substantial advantages in data efficiency and reconstruction accuracy. This survey explores the theoretical foundations of CS, including sensing matrices, sparse bases, and
[...] Read more.
Compressive Sensing (CS) is a transformative signal processing framework that enables sparse signal acquisition at rates below the Nyquist limit, offering substantial advantages in data efficiency and reconstruction accuracy. This survey explores the theoretical foundations of CS, including sensing matrices, sparse bases, and recovery algorithms, with a focus on its applications in power engineering. CS has demonstrated significant potential in enhancing key areas such as state estimation (SE), fault detection, fault localization, outage identification, harmonic source identification (HSI), Power Quality Detection condition monitoring, and so on. Furthermore, CS addresses challenges in data compression, real-time grid monitoring, and efficient resource utilization. A case study on smart meter data recovery demonstrates the practical application of CS in real-world power systems. By bridging CS theory and its application, this survey underscores its potential to drive innovation, efficiency, and sustainability in power engineering and beyond.
Full article
(This article belongs to the Section Wireless Control Networks)
►▼
Show Figures
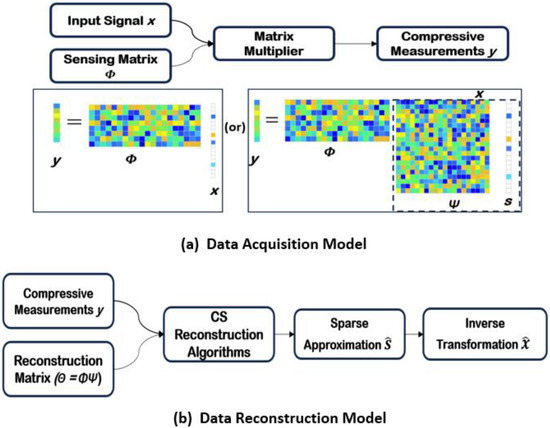
Figure 1
Open AccessReview
Network Diffusion Algorithms and Simulators in IoT and Space IoT: A Systematic Review
by
Charbel Mattar, Jacques Bou Abdo, Jacques Demerjian and Abdallah Makhoul
J. Sens. Actuator Netw. 2025, 14(2), 27; https://doi.org/10.3390/jsan14020027 - 4 Mar 2025
Cited by 1
Abstract
Network diffusion algorithms and simulators play a critical role in understanding how information, data, and malware propagate across various network topologies in Internet of Things and Space IoT configurations. This paper conducts a systematic literature review (SLR) of the key diffusion algorithms and
[...] Read more.
Network diffusion algorithms and simulators play a critical role in understanding how information, data, and malware propagate across various network topologies in Internet of Things and Space IoT configurations. This paper conducts a systematic literature review (SLR) of the key diffusion algorithms and network simulators utilized in studies over the past decade. The review focuses on identifying the algorithms and simulators employed, their strengths and limitations, and how their performance is evaluated under different IoT network topologies. Common network simulators, such as NS-3, Cooja, and OMNeT++ are explored, highlighting their features, scalability, and suitability for different IoT network scenarios. Additionally, network diffusion algorithms, including epidemic, cascading, and threshold models, are analyzed in terms of their effectiveness, complexity, and applicability in IoT environments with diverse network topologies. This SLR aims to provide a comprehensive reference for researchers and practitioners when selecting appropriate tools and methods for simulating and analyzing network diffusion across IoT and Space IoT configurations.
Full article
(This article belongs to the Special Issue Applications of Wireless Sensor Networks: Innovations and Future Trends)
►▼
Show Figures
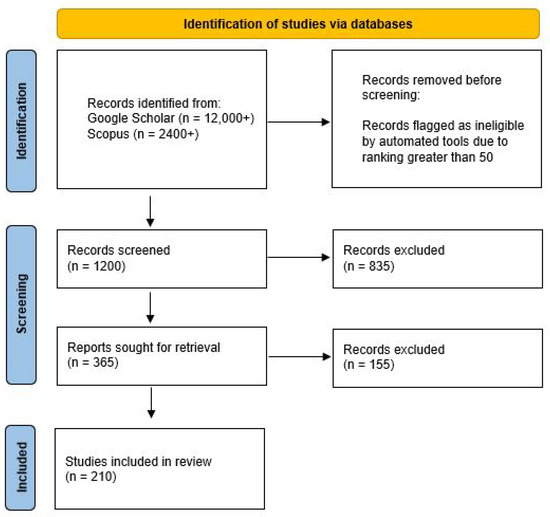
Figure 1
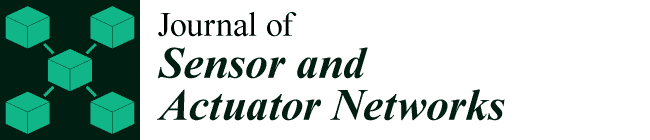
Journal Menu
► ▼ Journal Menu-
- JSAN Home
- Aims & Scope
- Editorial Board
- Reviewer Board
- Topical Advisory Panel
- Instructions for Authors
- Special Issues
- Topics
- Sections
- Article Processing Charge
- Indexing & Archiving
- Editor’s Choice Articles
- Most Cited & Viewed
- Journal Statistics
- Journal History
- Journal Awards
- Conferences
- Editorial Office
Journal Browser
► ▼ Journal BrowserHighly Accessed Articles
Latest Books
E-Mail Alert
News
7 April 2025
Meet Us at the 4th National Conference on Electronic Information Materials and Devices, 18–20 April 2025, Nanjing, China
Meet Us at the 4th National Conference on Electronic Information Materials and Devices, 18–20 April 2025, Nanjing, China
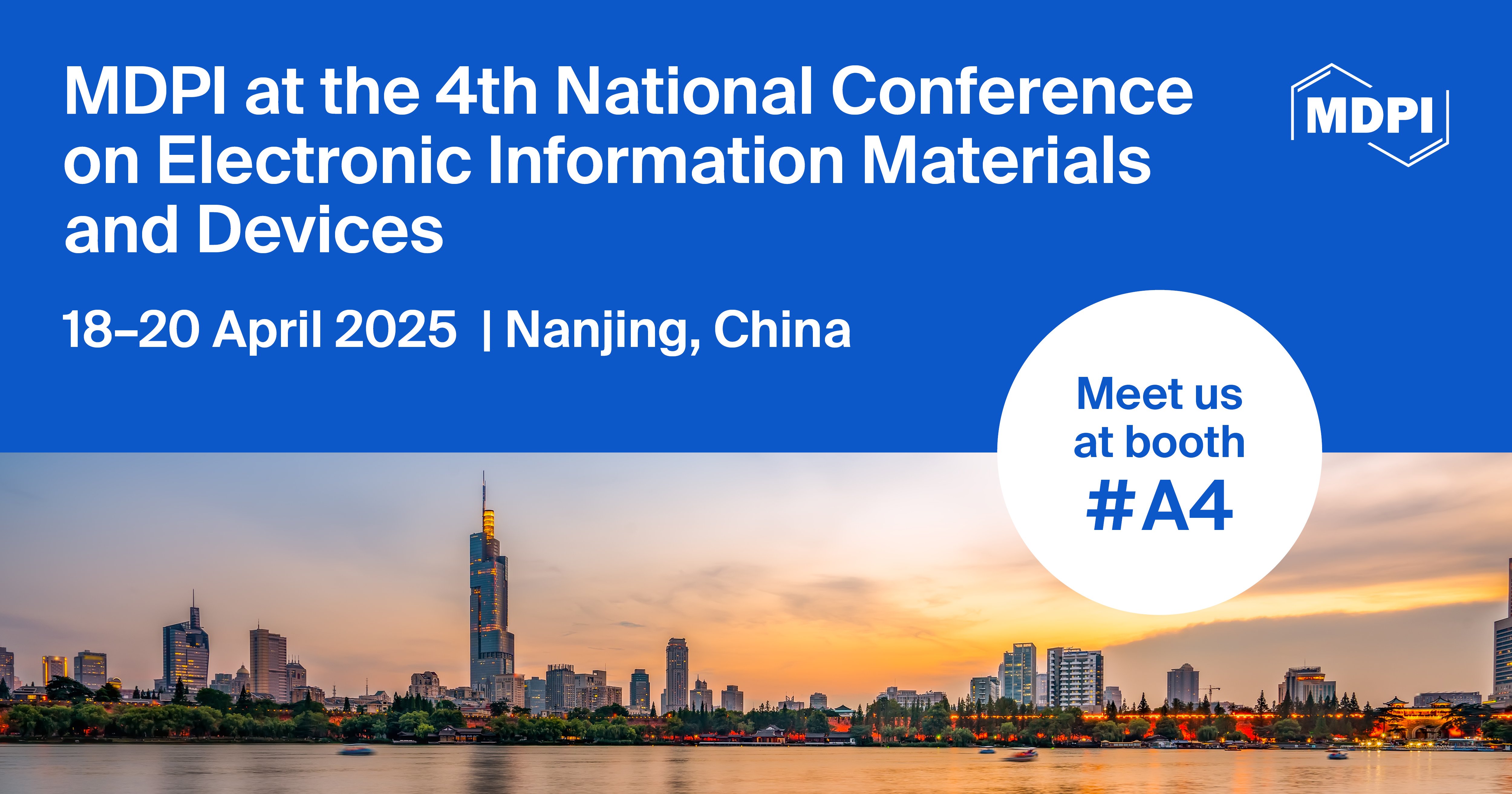
2 April 2025
MDPI INSIGHTS: The CEO's Letter #21 - Annual Report, Swiss Consortium, IWD, ICARS, Serbia
MDPI INSIGHTS: The CEO's Letter #21 - Annual Report, Swiss Consortium, IWD, ICARS, Serbia
Topics
Topic in
Applied Sciences, Electronics, JSAN, Photonics, Sensors, Telecom
Machine Learning in Communication Systems and Networks, 2nd Edition
Topic Editors: Yichuang Sun, Haeyoung Lee, Oluyomi SimpsonDeadline: 20 July 2025
Topic in
Applied Sciences, Electronics, IoT, JSAN, Network, Sensors, Telecom, Technologies
Wireless Energy Harvesting and Power Transfer for Communications and Networks
Topic Editors: Yichuang Sun, Arooj Mubashara Siddiqui, Xiaojing Chen, Oluyomi SimpsonDeadline: 31 October 2025
Topic in
Applied Sciences, Computers, Electronics, JSAN, Technologies
Emerging AI+X Technologies and Applications
Topic Editors: Byung-Seo Kim, Hyunsik Ahn, Kyu-Tae LeeDeadline: 31 December 2025
Topic in
Applied Sciences, Computers, JSAN, Technologies, BDCC, Sensors, Telecom, Electronics
Electronic Communications, IOT and Big Data, 2nd Volume
Topic Editors: Teen-Hang Meen, Charles Tijus, Cheng-Chien Kuo, Kuei-Shu Hsu, Jih-Fu TuDeadline: 31 March 2026

Conferences
Special Issues
Special Issue in
JSAN
AI-Assisted Machine-Environment Interaction
Guest Editors: Manolo Dulva Hina, Amar Ramdane-CherifDeadline: 30 April 2025
Special Issue in
JSAN
Recent Trends and Advancements in Location Fingerprinting
Guest Editors: Cristiano Pendão, Ivo Silva, Jianwei NiuDeadline: 31 May 2025
Special Issue in
JSAN
Feature Papers in the Section of Network Security and Privacy
Guest Editors: Jordi Mongay Batalla, Pascal Lorenz, Ioannis ChatzigiannakisDeadline: 31 May 2025
Special Issue in
JSAN
Applications of Wireless Sensor Networks: Innovations and Future Trends
Guest Editors: Dionisis Kandris, Eleftherios Anastasiadis, Purav ShahDeadline: 30 June 2025