Topic Menu
► Topic MenuTopic Editors
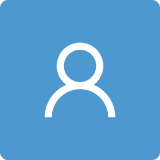
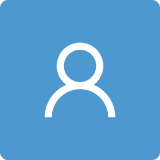
Spatial Epidemiology and GeoInformatics
Topic Information
Dear Colleagues,
Under the broad area of Spatial Epidemiology that includes the description and analysis of geographic variation in health outcomes, this Topic collection will address the association of multiple contextual factors (including environmental, demographic, socio- economic, psychological, lifestyle and behavioral factors, e.g., dietary habits, smoking, physical activity, etc.), with a variety of health outcomes, including both NCD and communicable factors (e.g., COVID-19). Spatial epidemiology and geoinformatics are two interdisciplinary research approaches that have been increasingly used in recent years to address public health challenges. Spatial epidemiology studies the distribution of disease across space, while geoinformatics focuses on the use of geospatial data to identify geographic patterns in health outcomes. These approaches offer researchers unique opportunities to gain insights into how environmental factors influence the spread of disease and how specific interventions can be targeted to specific regions or populations. Despite their potential, there are a number of challenges associated with these research methods. These include, among others, the availability and quality of data, as well as the techniques and tools. This Topic collection aims to provide a wide overview of how to approach spatial problems in epidemiology, with a specific focus on novel methodologies, e.g., those deriving from spatial analysis, spatiotemporal modelling, statistical and probability theory and practice, artificial intelligence/machine learning techniques and software presentation, as well as public health applications. Papers that focus on spatial health and covariate data, visualization and spatial exploration, quantification of spatial patterns, detection of spatial heterogeneities and statistical, analytical methods for spatial prediction are welcome.
Prof. Dr. Christos Chalkias
Dr. Demosthenes Panagiotakos
Topic Editors
Keywords
- spatial epidemiology
- geoinformatics
- health geography
- GIS modeling
- public health
- global health
- chronic disease
- infectious disease
- COVID-19
- social medicine
Participating Journals
Journal Name | Impact Factor | CiteScore | Launched Year | First Decision (median) | APC | |
---|---|---|---|---|---|---|
![]()
Healthcare
|
2.8 | 2.7 | 2013 | 19.5 Days | CHF 2700 | Submit |
![]()
International Journal of Environmental Research and Public Health
|
- | 5.4 | 2004 | 29.6 Days | CHF 2500 | Submit |
![]()
ISPRS International Journal of Geo-Information
|
3.4 | 6.2 | 2012 | 35.5 Days | CHF 1700 | Submit |
MDPI Topics is cooperating with Preprints.org and has built a direct connection between MDPI journals and Preprints.org. Authors are encouraged to enjoy the benefits by posting a preprint at Preprints.org prior to publication:
- Immediately share your ideas ahead of publication and establish your research priority;
- Protect your idea from being stolen with this time-stamped preprint article;
- Enhance the exposure and impact of your research;
- Receive feedback from your peers in advance;
- Have it indexed in Web of Science (Preprint Citation Index), Google Scholar, Crossref, SHARE, PrePubMed, Scilit and Europe PMC.