Residual Neural Networks for Origin–Destination Trip Matrix Estimation from Traffic Sensor Information
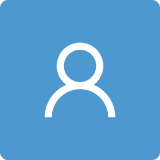
Round 1
Reviewer 1 Report
Reviewer’s comments
Thanks for the privilege given to me to review this manuscript; I have read it with great interest. The authors present a novel addition to the ODEP by using residual neural network to solve the problem for origin-destination trip matrix estimation from traffic sensors information. I feel some points must be considered and certainly need some amendments. Some obvious and major corrections are highlighted below.
What is your contribution or innovation of your research? Please identify it.
There is not an explanation of your dataset. Please provide and explain it carefully.
Where are your experiment designs and results?
No statistical analysis in your result.
The authors lack a discussion section.
There is no comprehensive discussion of the comparison analysis. The discussion section must focus on your summary, interpretations, implications, limitations, and recommendations of the results.
Conclusions are not complete due to the fact that there is no comprehensive discussion of the results.
I feel that minor editing of English language required.
Author Response
Main changes with respect to the latest version of the manuscript
- The Literature Review section has been improved with new references.
- The research design methods have been improved with new paragraphs.
- The interesting points of discussion raised by the reviewers have been added in their relevant sections.
- New illustrations have been added to avoid any potential confusion for the readers.
- Some parts have been rewritten to give coherent insight into the manuscript.
- More discussions have been given to highlight the superiority of the proposed methodology results.
- The results section has been modified with a new analysis representation.
- The conclusion has been improved to be more specific.
- The references section has been checked and updated.
- The language has been refined carefully using suggestions from professional editing colleagues.
More detailed changes are outlined in the responses to the reviewers' comments.
Detailed responses to the reviewers' comments:
Our responses are presented in italic, bold font.
Reviewer 1:
Thanks for the privilege given to me to review this manuscript; I have read it with great interest. The authors present a novel addition to the ODEP by using residual neural network to solve the problem for origin-destination trip matrix estimation from traffic sensors information. I feel some points must be considered and certainly need some amendments. Some obvious and major corrections are highlighted below.
We really appreciate your opinion on our work.
What is your contribution or innovation of your research? Please identify it.
Thank you for your valuable suggestion. In the revised manuscript, we stated clearly that we introduced a new contribution to the traffic sensors network problem using machine learning based on an exhaustive literature assessment. To the best of the authors' knowledge, it is the first time to use Residual neural networks for the origin-destination trip matrix prediction problem from traffic sensors information. The framework is unique and general.
There is not an explanation of your dataset. Please provide and explain it carefully.
Thank you for your hint, In the revised manuscript, we stated that the data set is generated randomly using equations (14 and 15) and the assignment method in Algorithm 1.
Where are your experiment designs and results?
Thank you for your recommendation. We added a new network to the current network. So that two networks are used to experiment with the methodology.
No statistical analysis in your result.
Using machine learning limit the use of statistical analysis like p-value and other metrics, unlike classical statistical tool like regression analysis.
The authors lack a discussion section. There is no comprehensive discussion of the comparison analysis. The discussion section must focus on your summary, interpretations, implications, limitations, and recommendations of the results. Conclusions are not complete due to the fact that there is no comprehensive discussion of the results.
Thank you for the suggestions. In the revised manuscript, a new discussion is added with the enhanced experimental section to rectify the mentioned drawbacks.
Comments on the Quality of English Language: I feel that minor editing of English language required
Thank you for your comment. The revised manuscript's language has been refined carefully using suggestions from professional editing colleagues. We again appreciate your opinion on our work
At last, the authors would like to thank the Associate Editor and the reviewers for their constructive comments that have led to significant improvements in the quality of the paper."
Reviewer 2 Report
1. Compare with state of art approaches and report and analyze results.
2. Present efficiency analysis for proposed and compared approaches.
3. Improve figures and structure and organization of the paper.
No comments
Author Response
Main changes with respect to the latest version of the manuscript
- The Literature Review section has been improved with new references.
- The research design methods have been improved with new paragraphs.
- The interesting points of discussion raised by the reviewers have been added in their relevant sections.
- New illustrations have been added to avoid any potential confusion for the readers.
- Some parts have been rewritten to give coherent insight into the manuscript.
- More discussions have been given to highlight the superiority of the proposed methodology results.
- The results section has been modified with a new analysis representation.
- The conclusion has been improved to be more specific.
- The references section has been checked and updated.
- The language has been refined carefully using suggestions from professional editing colleagues.
More detailed changes are outlined in the responses to the reviewers' comments.
Detailed responses to the reviewers' comments:
Our responses are presented in italic, bold font.
Reviewer 2:
- Compare with state of art approaches and report and analyze results.
Thank you for your recommendation. In the revised manuscript, we added a new popular benchmark network to the current network to Compare with state-of-the-art approaches and report and analyze results.
- Present efficiency analysis for proposed and compared approaches.
Thank you for your recommendation. In the revised manuscript, we presented Table 2 to Present efficiency analysis for proposed and compared approaches from the literature.
- Improve figures and structure and organization of the paper.
Thank you for your recommendation. The revised manuscript is improved according to be better in its structure and organization.
At last, the authors would like to thank the Associate Editor and the reviewers for their constructive comments that have led to significant improvements in the quality of the paper."
Reviewer 3 Report
The authors present to solve sensor location problem and OD estimation problem by using machine learning algorithms. The idea is interesting. However, there are some issues need to be further addressed in order to improve the quality of this paper.
1. The title needs to be adjusted. In this paper, the authors only applied the residual neural networks to estimate OD flows. The neural network itself is not the contribution of the authors. In addition, the sensor location problem is also involved, and hence needs to be mentioned.
2. The abstract needs to be improved. In the abstract, the motivation of the paper is required. That is, it is necessary to illustrate why the residual neural networks are used in modeling ODEP and TSLP. In addition, the key steps of the proposed approach or framework need to be included. Some important findings are also required.
3. The literature review needs to be improved. The authors are suggested to divide the section into two subsections, one is ODEP, and another is TSLP, since two problems are jointly solved by the proposed approach. In addition, there are some recent works on ODEP based on machine learning, which are not mentioned in the literature review section. Please search and cited them in this section. Some of them are as follows:
(1) Learn, assign, and search: Real-time estimation of dynamic origin-destination flows using machine learning algorithms.
(2) Origin-destination pattern estimation based on trajectory reconstruction using automatic license plate recognition data.
(3) Dynamic origin‐destination flow estimation using automatic vehicle identification data: A 3D convolutional neural network approach
(4) Signalized arterial origin-destination flow estimation using flawed vehicle trajectories: A self-supervised learning approach without ground truth.
4. The motivation is not very clear. In lines 197-205, the authors tried to indicate the drawbacks of the existing studies. However, as mentioned above, there are indeed some works on ODEP based on machine learning. Please adjust the motivation. It seems that the application of machine learning to ODEP-TSLP is novel. However, this point is simplified in the paper.
5. Figure 3 depicts the architecture of the DRNN. However, the architecture was not proposed or designed by the authors. Please try to combine the specific problem and the DRNN architecture to produce the developed ODEP model. In other words, the input and output are required to bind to the specific problem. The developed model is yours but not the DRNN architecture.
6. In section 5, the proposed model is not fully evaluated and more experiments are required. The typical evaluation measures need to be given. The OD estimation results and assigned link flow comparisons are also required.
7. Minor comments:
(1) The symbols of the equations needs to be consistent and adjusted to make them more formal.
(2) The pseudocode of the algorithm 1 needs to be described in a table or a figure.
-
The paper needs an appropriate language revision.
Author Response
Main changes with respect to the latest version of the manuscript
- The Literature Review section has been improved with new references.
- The research design methods have been improved with new paragraphs.
- The interesting points of discussion raised by the reviewers have been added in their relevant sections.
- New illustrations have been added to avoid any potential confusion for the readers.
- Some parts have been rewritten to give coherent insight into the manuscript.
- More discussions have been given to highlight the superiority of the proposed methodology results.
- The results section has been modified with a new analysis representation.
- The conclusion has been improved to be more specific.
- The references section has been checked and updated.
- The language has been refined carefully using suggestions from professional editing colleagues.
More detailed changes are outlined in the responses to the reviewers' comments.
Detailed responses to the reviewers' comments:
Our responses are presented in italic, bold font.
Reviewer 3:
The authors present to solve sensor location problem and OD estimation problem by using machine learning algorithms. The idea is interesting. However, there are some issues need to be further addressed in order to improve the quality of this paper.
Thank you for your positive opinion of our work.
- The title needs to be adjusted. In this paper, the authors only applied the residual neural networks to estimate OD flows. The neural network itself is not the contribution of the authors. In addition, the sensor location problem is also involved, and hence needs to be mentioned.
Thank you for your valuable comment that may help to outline our contribution. In the revised manuscript, we could argue the two referred points. First, applying the DRNNS constitutes a real contribution to the study, where its application is impossible without the methodology presented in this study. In the literature review, deep learning is concerned with local traffic flow prediction, where there is a correlation between the input with time in the network. On the other hand, there is no contribution presented regarding the TSLP since we used a previously developed algorithm by the corresponding author.
- The abstract needs to be improved. In the abstract, the motivation of the paper is required. That is, it is necessary to illustrate why the residual neural networks are used in modeling ODEP and TSLP. In addition, the key steps of the proposed approach or framework need to be included. Some important findings are also required.
Thank you for your suggestion. The abstract is modified accordingly.
- The literature review needs to be improved. The authors are suggested to divide the section into two subsections, one is ODEP, and another is TSLP, since two problems are jointly solved by the proposed approach. In addition, there are some recent works on ODEP based on machine learning, which are not mentioned in the literature review section. Please search and cited them in this section. Some of them are as follows: (1) Learn, assign, and search: Real-time estimation of dynamic origin-destination flows using machine learning algorithms.b (2) Origin-destination pattern estimation based on trajectory reconstruction using automatic license plate recognition data. (3) Dynamic origin‐destination flow estimation using automatic vehicle identification data: A 3D convolutional neural network approach (4) Signalized arterial origin-destination flow estimation using flawed vehicle trajectories: A self-supervised learning approach without ground truth.
Thank you for your suggested references. All the mentioned references are added in their relevant places in the revised manuscript.
- The motivation is not very clear. In lines 197-205, the authors tried to indicate the drawbacks of the existing studies. However, as mentioned above, there are indeed some works on ODEP based on machine learning. Please adjust the motivation. It seems that the application of machine learning to ODEP-TSLP is novel. However, this point is simplified in the paper.
Thank you for your recommendation. In the revised manuscript, the lines are modified accordingly by adding the references and needed discussion.
- Figure 3 depicts the architecture of the DRNN. However, the architecture was not proposed or designed by the authors. Please try to combine the specific problem and the DRNN architecture to produce the developed ODEP model. In other words, the input and output are required to bind to the specific problem. The developed model is yours but not the DRNN architecture.
Thank you for your recommendation. In the revised manuscript, the DRRNS symbols in Figure 3 description are correlated with the input and output description in the problem formulation section. Therefore the reader can easily extract the specificity of the proposed DRNNS architecture.
- In section 5, the proposed model is not fully evaluated and more experiments are required. The typical evaluation measures need to be given. The OD estimation results and assigned link flow comparisons are also required.
Thank you for your recommendation. In the revised manuscript, we added a new popular benchmark network to the current network to Compare with state-of-the-art approaches and report and analyze results.
- Minor comments:
(1) The symbols of the equations needs to be consistent and adjusted to make them more formal. (2) The pseudocode of the algorithm 1 needs to be described in a table or a figure.
Thank you for the minor corrections. In the revised manuscript, the symbols are adjusted to ensure consistency, and algorithm one is placed in Table 1.
Comments on the Quality of English Language
- The paper needs an appropriate language revision.
The language has been refined carefully using suggestions from professional editing colleagues.
At last, the authors would like to thank the Associate Editor and the reviewers for their constructive comments that have led to significant improvements in the quality of the paper."
Reviewer 4 Report
Reviewer #
This work was constructed to overcome the traffic flow problems in locating origin-destination and dependency between network topology and traffic flow. The authors engaged the Residual Neural Networks to predict all routes and traffic of the existing destination to provide the optimized solution for locating traffic sensors. The idea can be fulfilled in the recent area of IoT and vehicle electronic devices. Meanwhile, I have the following concerns about the study:
In abstract:
(1) The authors have to point out the recent studies' problems in the research area.
(2) Please, clarify the feature of a Residual Neural Network (as a very deep neural network) than other approaches to overcome the mentioned challenges.
(3) The authors have to present the outcome of the study on improving the performance of the traffic sensors.
(4) Please, utilize a different combination of "traffic flow data" such as "traffic flow", "traffic dataflow", or other standard terms in the research area.
In introduction:
(5) Interrogative sentences are the most popular for presenting literature review papers. I think they seem not proper for a research paper.
(6) The authors have to give a picture of the idea innovation and problem definition. The researchers will confuse finding the idea novelty until analyze sections 2-3.
In state of the art:
(7) I think the title of the section was not reflected its purpose.
(8) I faced many numbers of the old studies. In the literature review, the authors have to aim at the recent studies in the research area. Please, remove the inessential and old works to attend to the novel methods.
(9) Many deep learning and machine learning-based approaches have been conducted to predict traffic sensor networks. Please, refer to one or two recent studies and compare them with the case study idea.
(10) Please prove the supremacy of the idea over the recent Fuzzy-logic and deep Q-learning methods in the research area.
In problem formulation:
(11) I faced some typo problems such as "r. has", and "as follows where;".
(12) Please, revise Table 1. The authors repeated the "Congestion effect" assumption twice.
(13) Please, describe all abbreviations before employing them such as "DUE", etc.
(14) Please, utilize an integrated format to address Equations. The authors employed different formats for the issue such as "Equation" and "Eq. ".
In methodology:
(15) The authors can simplify some sentences such as " The genuine unknown demand, the reference/historical demand, and the anticipated demand" to "The genuine unknown, the reference/historical, and the anticipated demands" or other simplified forms.
(16) Please, consider that the approach and algorithm follow two different concepts. Hence, please, revise "the iterative approach below".
(17) Please, define and specify the input and output of the algorithm.
(18)I think seems not proper that refers to a reference in the algorithm structure. 'The authors can refer to the reference on the main text or label of the algorithm.
(19) In Figure 3, the authors can mitigate four blocks of "Residual building units" to one block of "Residual building units 1-4".
(20) I think the related Figures and layers of the Residual neural network could be useful when presented as an architecture of a neural network.
(21) The huge number of case studies clarified the steps of the DRNN. The authors employed the learning method to solve the problem of traffic sensor flows and locations. Hence, please, target the related explanation to DRNN on the steps of balancing traffic and location assignment instead of its basic information.
In numerical study:
(22) Figure 6 reflected the efficiency of model learning in two phases of training and testing. It seems not proper since was employed the performance to describe the Figure.
(23) I think the presented results were not sufficient and convincing. Please, add and provide the comparison results to justify the role of the idea over other recent approaches to solving the traffic flow problem.
(24) The authors have to discuss the reported results.
(25) Figure 8 consists of four Figures that the authors have to label (such as (a)-(d)) and clearly explain them.
In conclusion:
(26) It is not routine that refers to the references in the conclusion. All concepts are clarified in other sections and this section only concludes them.
Comments for author File: Comments.pdf
The technical English writing of the manuscript seems convincing.
Author Response
Main changes with respect to the latest version of the manuscript
- The Literature Review section has been improved with new references.
- The research design methods have been improved with new paragraphs.
- The interesting points of discussion raised by the reviewers have been added in their relevant sections.
- New illustrations have been added to avoid any potential confusion for the readers.
- Some parts have been rewritten to give coherent insight into the manuscript.
- More discussions have been given to highlight the superiority of the proposed methodology results.
- The results section has been modified with a new analysis representation.
- The conclusion has been improved to be more specific.
- The references section has been checked and updated.
- The language has been refined carefully using suggestions from professional editing colleagues.
More detailed changes are outlined in the responses to the reviewers' comments.
Detailed responses to the reviewers' comments:
Our responses are presented in italic, bold font.
Reviewer 4:
This work was constructed to overcome the traffic flow problems in locating origin-destination and dependency between network topology and traffic flow. The authors engaged the Residual Neural Networks to predict all routes and traffic of the existing destination to provide the optimized solution for locating traffic sensors. The idea can be fulfilled in the recent area of IoT and vehicle electronic devices. Meanwhile, I have the following concerns about the study:
Thank you for your informative summary of our work.
In abstract:
(1) The authors have to point out the recent studies' problems in the research area.
Thank you for your recommendation. The abstract is a tight area, so the manuscript body has been updated accordingly.
(2) Please, clarify the feature of a Residual Neural Network (as a very deep neural network) than other approaches to overcome the mentioned challenges.
Thank you for your recommendation. In the revised manuscript, the abstract is modified accordingly.
(3) The authors have to present the outcome of the study on improving the performance of the traffic sensors.
The abstract indicated that a considerable degree of accuracy is obtained using the DRNNS, where its application in the presented methodology is innovative to the problem.
(4) Please, utilize a different combination of "traffic flow data" such as "traffic flow", "traffic dataflow", or other standard terms in the research area.
Thank you for the suggestion. We tried to follow the referred flow terms.
In introduction:
(5) Interrogative sentences are the most popular for presenting literature review papers. I think they seem not proper for a research paper.
Thank you for the recommendation. In the revised manuscript, some parts of the introduction are modified in an attempt to mitigate the referred drawback.
(6) The authors have to give a picture of the idea innovation and problem definition. The researchers will confuse finding the idea novelty until analyze sections 2-3.
Thank you for the suggestion. In the revised manuscript, an abstract picture of the idea innovation and problem definition is given in the introduction.
In state of the art:
(7) I think the title of the section was not reflected its purpose.
Thank you for the suggestion. In the revised manuscript, it was changed to the Background section.
(8) I faced many numbers of the old studies. In the literature review, the authors have to aim at the recent studies in the research area. Please, remove the inessential and old works to attend to the novel methods.
Thank you for the suggestion. In the revised manuscript, the bacgroung section is updated with many updated references like:
- J. Ou, J. Lu, J. Xia, C. An, and Z. Lu, "Learn, assign, and search: real-time estimation of dynamic origin-destination flows using machine learning algorithms," IEEE Access, vol. 7, pp. 26967-26983, 2019.
- W. Rao, Y.-J. Wu, J. Xia, J. Ou, and R. Kluger, "Origin-destination pattern estimation based on trajectory reconstruction using automatic license plate recognition data," Transportation Research Part C: Emerging Technologies, vol. 95, pp. 29-46, 2018.
- K. Tang, Y. Cao, C. Chen, J. Yao, C. Tan, and J. Sun, "Dynamic origin‐destination flow estimation using automatic vehicle identification data: A 3D convolutional neural network approach," Computer‐Aided Civil and Infrastructure Engineering, vol. 36, no. 1, pp. 30-46, 2021.
- Q. Wang, Y. Yuan, Q. Zhang, and X. T. Yang, "Signalized arterial origin-destination flow estimation using flawed vehicle trajectories: A self-supervised learning approach without ground truth," Transportation Research Part C: Emerging Technologies, vol. 145, p. 103917, 2022.
(9) Many deep learning and machine learning-based approaches have been conducted to predict traffic sensor networks. Please, refer to one or two recent studies and compare them with the case study idea.
Thank you for the suggestion. In the revised manuscript, we stated clearly after a wide review by the authors and based on the extensive review article in
- Owais, "Traffic Sensor Location Problem: Three Decades of Research," Expert Systems with Applications, p. 118134, 2022.
It is the first application of machine learning for a formulated problem.
(10) Please prove the supremacy of the idea over the recent Fuzzy-logic and deep Q-learning methods in the research area.
Thank you for the comment. In the revised manuscript, we indicated that Fuzzy-logic and deep Q-learning are unsuitable for the formulated problem, which is closer to nonlinear regression than decision problems. Therefore deep learning based on neural network structures is more useful to our problem.
In problem formulation:
(11) I faced some typo problems such as "r. has", and "as follows where;".
(12) Please, revise Table 1. The authors repeated the "Congestion effect" assumption twice.
Thank you for the valuable observation and careful revising. In the revised manuscript, we tracked all the typos and corrected them.
(13) Please, describe all abbreviations before employing them such as "DUE", etc.
Thank you for the suggestion. In the revised manuscript, all abbreviations are defined before their first mention.
(14) Please, utilize an integrated format to address Equations. The authors employed different formats for the issue such as "Equation" and "Eq. ".
Thank you for the valuable observation. We employed the full term "Equation" in the revised manuscript in all the text.
In methodology:
(15) The authors can simplify some sentences such as " The genuine unknown demand, the reference/historical demand, and the anticipated demand" to "The genuine unknown, the reference/historical, and the anticipated demands" or other simplified forms.
Thank you for the suggestion. In the revised manuscript, we modified the text to remove the terms to more simplified forms.
(16) Please, consider that the approach and algorithm follow two different concepts. Hence, please, revise "the iterative approach below".
Thank you for the suggestion. In the revised manuscript, the algorithm presentation is reformulated in Table 2.
(17) Please, define and specify the input and output of the algorithm.
Thank you for the suggestion. In the revised manuscript, we specified the input as one historical O/D matrix, network structure, and traffic assignment assumption, whereas the output is a learning scheme that enables a subset of traffic sensor information to predict the corresponding O/D matrix.
(18)I think seems not proper that refers to a reference in the algorithm structure. 'The authors can refer to the reference on the main text or label of the algorithm.
Thank you for the recommendation. In the revised manuscript, we moved the reference to the footnote of the algorithm table.
(19) In Figure 3, the authors can mitigate four blocks of "Residual building units" to one block of "Residual building units 1-4". (20) I think the related Figures and layers of the Residual neural network could be useful when presented as an architecture of a neural network. (21) The huge number of case studies clarified the steps of the DRNN. The authors employed the learning method to solve the problem of traffic sensor flows and locations. Hence, please, target the related explanation to DRNN on the steps of balancing traffic and location assignment instead of its basic information.
Thank you for the valuable suggestions, which we tried our best to follow in the revised manuscript while trying to keep the DRNNS presentation as informative as possible.
In numerical study:
(22) Figure 6 reflected the efficiency of model learning in two phases of training and testing. It seems not proper since was employed the performance to describe the Figure.
Thank you for the hint. In the revised manuscript, we used efficiency terms to describe the Figure.
(23) I think the presented results were not sufficient and convincing. Please, add and provide the comparison results to justify the role of the idea over other recent approaches to solving the traffic flow problem. (24) The authors have to discuss the reported results.
Thank you for your recommendation. In the revised manuscript, we added a new popular benchmark network to the current network to Compare with state-of-the-art approaches and report and analyze results.
(25) Figure 8 consists of four Figures that the authors have to label (such as (a)-(d)) and clearly explain them.
Thank you for your recommendation. In the revised manuscript, Figure 8 is updated accordingly.
In conclusion:
(26) It is not routine that refers to the references in the conclusion. All concepts are clarified in other sections and this section only concludes them.
Thank you for your suggestion. In the revised manuscript, the references are removed from the conclusions.
At last, the authors would like to thank the Associate Editor and the reviewers for their constructive comments that have led to significant improvements in the quality of the paper."
Reviewer 5 Report
Motivation and Problem Statement: The introduction section should more clearly describe the motivation for addressing the traffic flow prediction problem using Residual Neural Networks. Additionally, provide a more comprehensive review of the existing literature on traffic management and control, highlighting the gaps in the current methods and how the proposed method can address those gaps.
Methodology: The methodology section should provide a more detailed explanation of the Residual Neural Network architecture, including the choice of activation functions, the number of layers, and the rationale behind these choices. Also, discuss any relevant hyperparameter tuning and the model's training process.
Literature Review: To broaden the scope of this paper, the authors should refer to some work such as: Age Efficient Optimization in UAV-Aided VEC Network: A Game Theory Viewpoint, Data freshness optimization under CAA in the UAV-aided MECN: a potential game perspective and Ultra super fast authentication protocol for electric vehicle charging using extended chaotic maps.
Data Generation: Clarify the process of generating random synthetic flow data from historical demand data. Describe the statistical properties of the synthetic data, such as the distribution and correlation structure, and explain how this synthetic data is representative of real-world traffic flow patterns.
Performance Metrics: In addition to the root mean square error (RMSE), consider including other performance metrics such as Mean Absolute Error (MAE) and R-squared to provide a more comprehensive evaluation of the model's performance. Discuss the trade-offs between these metrics and justify the choice of RMSE as the primary performance metric.
Comparison with Baseline Methods: The paper should include a comparison of the proposed method with existing techniques for traffic flow prediction and sensor deployment. This will help to highlight the advantages of using Residual Neural Networks over traditional methods.
Robustness Analysis: Discuss the robustness of the proposed method in more detail, including how it performs under different traffic conditions, varying sensor locations, and different levels of noise in the data.
Case Study: Provide more information on the large-scale network used for testing the model. Describe its characteristics, such as the number of nodes, links, and traffic demand patterns. Additionally, include a discussion of how the proposed method can be applied to real-world traffic management scenarios.
Conclusion and Future Work: Summarize the key findings and contributions of the paper, and discuss potential avenues for future research in this area, such as exploring other deep learning architectures, incorporating additional data sources, or addressing other traffic management challenges.
Figures and Tables: Ensure that all figures and tables are clear, appropriately labeled, and referenced in the text. Include detailed captions to aid in the understanding of the presented results.
none
Author Response
Main changes with respect to the latest version of the manuscript
- The Literature Review section has been improved with new references.
- The research design methods have been improved with new paragraphs.
- The interesting points of discussion raised by the reviewers have been added in their relevant sections.
- New illustrations have been added to avoid any potential confusion for the readers.
- Some parts have been rewritten to give coherent insight into the manuscript.
- More discussions have been given to highlight the superiority of the proposed methodology results.
- The results section has been modified with a new analysis representation.
- The conclusion has been improved to be more specific.
- The references section has been checked and updated.
- The language has been refined carefully using suggestions from professional editing colleagues.
More detailed changes are outlined in the responses to the reviewers' comments.
Detailed responses to the reviewers' comments:
Our responses are presented in italic, bold font.
Reviewer 5:
Motivation and Problem Statement: The introduction section should more clearly describe the motivation for addressing the traffic flow prediction problem using Residual Neural Networks. Additionally, provide a more comprehensive review of the existing literature on traffic management and control, highlighting the gaps in the current methods and how the proposed method can address those gaps.
Thank you for your recommendation. The revised manuscript's introduction section is modified to Explain the rationale for utilizing Residual Neural Networks to solve the traffic flow prediction issue. In addition, offer a more thorough assessment of the existing literature on traffic management and control, stressing the shortcomings in present approaches and how the suggested method might solve those deficiencies.
Methodology: The methodology section should provide a more detailed explanation of the Residual Neural Network architecture, including the choice of activation functions, the number of layers, and the rationale behind these choices. Also, discuss any relevant hyperparameter tuning and the model's training process.
Thank you for your recommendation. In the revised manuscript, the methods section is modified to include a more thorough explanation of the Residual Neural Network design, including the selection of activation functions, the number of layers, and the justification behind these decisions. Additionally, we detailed any pertinent hyperparameter adjustments and the model training procedure.
Literature Review: To broaden the scope of this paper, the authors should refer to some work such as: Age Efficient Optimization in UAV-Aided VEC Network: A Game Theory Viewpoint, Data freshness optimization under CAA in the UAV-aided MECN: a potential game perspective and Ultra super fast authentication protocol for electric vehicle charging using extended chaotic maps.
Thank you for your suggested references. All the mentioned references are added in their relevant places in the revised manuscript.
Data Generation: Clarify the process of generating random synthetic flow data from historical demand data. Describe the statistical properties of the synthetic data, such as the distribution and correlation structure, and explain how this synthetic data is representative of real-world traffic flow patterns.
Thank you for your recommendations. In the revised manuscript, we clarified the method of creating random synthetic flow data from historical demand data. Also, we described the statistical aspects of the synthetic data, such as the distribution and correlation structure, and explained how this synthetic data is typical of real-world traffic flow patterns.
Performance Metrics: In addition to the root mean square error (RMSE), consider including other performance metrics such as Mean Absolute Error (MAE) and R-squared to provide a more comprehensive evaluation of the model's performance. Discuss the trade-offs between these metrics and justify the choice of RMSE as the primary performance metric.
Thank you for your valuable point of discussion. In the revised manuscript, we used both the root mean square error (RMSE) and squared to provide a more comprehensive evaluation of the model's performance, while we found that the Mean Absolute Error (MAE) does not provide new information over the robust RMSE metric.
Comparison with Baseline Methods: The paper should include a comparison of the proposed method with existing techniques for traffic flow prediction and sensor deployment. This will help to highlight the advantages of using Residual Neural Networks over traditional methods.
Thank you for your recommendation. In the revised manuscript, we added a new popular benchmark network to the current network to Compare with state-of-the-art approaches and report and analyze results.
Robustness Analysis: Discuss the robustness of the proposed method in more detail, including how it performs under different traffic conditions, varying sensor locations, and different levels of noise in the data.
Thank you for your recommendation. In the revised manuscript, different traffic conditions are taken inherently in the synthetic data with multiple assessment steps. Where vary sensor locations effect is given in the newly added Figure 10. Tackling noise levels in the data is discussed in the conclusion.
Case Study: Provide more information on the large-scale network used for testing the model. Describe its characteristics, such as the number of nodes, links, and traffic demand patterns. Additionally, include a discussion of how the proposed method can be applied to real-world traffic management scenarios.
Thank you for your suggestions. In the revised manuscript, more details about the large-scale network are given.
Conclusion and Future Work: Summarize the key findings and contributions of the paper, and discuss potential avenues for future research in this area, such as exploring other deep learning architectures, incorporating additional data sources, or addressing other traffic management challenges.
Thank you for your suggestions. In the revised manuscript, the conclusion is modified accordingly.
Figures and Tables: Ensure that all figures and tables are clear, appropriately labeled, and referenced in the text. Include detailed captions to aid in the understanding of the presented results.
Thank you for your valuable recommendations. In the revised manuscript, all these issues are ensured while revising the second version of the manuscript.
At last, the authors would like to thank the Associate Editor and the reviewers for their constructive comments that have led to significant improvements in the quality of the paper."
Round 2
Reviewer 1 Report
The responses aren't clear and didn't answer my question.
The methodology and result cannot convince the performance of your proposed method.
Author Response
Detailed responses to the reviewers' comments:
Our responses are presented in italic, bold font.
Reviewer 1:
The responses aren't clear and didn't answer my question.
We are sorry that you have changed your positive comments from the first round of review hoping you approve the final manuscript version after the revision according to your valuable comments and other reviewers' comments.
The methodology and result cannot convince the performance of your proposed method
Thank you for your comment. In the revised manuscript you will find that the results of both the benchmark network and large-scale network support the superiority of the methodology in the prediction of O/D demand values. It is enough to refer you to Figure 8 and Table 3.
Reviewer 2 Report
No more comments
Author Response
Thank you for your help to make the manuscript reach its best quality.
Reviewer 3 Report
My comments have been properly addressed.
Author Response
Thank you for your help to make the manuscript reach its best quality.
Reviewer 4 Report
The authors well-overcooked the mentioned problems by applying the revisions. However, some details can be still re-checked, which you can find them here:
(8) I faced many numbers of the old studies. In the literature review, the authors have to aim at the recent studies in the research area. Please, remove the inessential and old works to attend to the novel methods.
Authors: Thank you for the suggestion. In the revised manuscript, the bacgroung section is updated with many updated references like:
- J. Ou, J. Lu, J. Xia, C. An, and Z. Lu, "Learn, assign, and search: real-time estimation of dynamic origin-destination flows using machine learning algorithms," IEEE Access, vol. 7, pp. 26967-26983, 2019.
- W. Rao, Y.-J. Wu, J. Xia, J. Ou, and R. Kluger, "Origin-destination pattern estimation based on trajectory reconstruction using automatic license plate recognition data," Transportation Research Part C: Emerging Technologies, vol. 95, pp. 29-46, 2018.
- K. Tang, Y. Cao, C. Chen, J. Yao, C. Tan, and J. Sun, "Dynamic origin‐destination flow estimation using automatic vehicle identification data: A 3D convolutional neural network approach," Computer‐Aided Civil and Infrastructure Engineering, vol. 36, no. 1, pp. 30-46, 2021.
- Q. Wang, Y. Yuan, Q. Zhang, and X. T. Yang, "Signalized arterial origin-destination flow estimation using flawed vehicle trajectories: A self-supervised learning approach without ground truth," Transportation Research Part C: Emerging Technologies, vol. 145, p. 103917, 2022.
Re-comment: I found the additional and recent studies. Nevertheless, some inessential and old works remain in the section. The authors can cure the level of the research study by removing them.
(10) Please prove the supremacy of the idea over the recent Fuzzy-logic and deep Q-learning methods in the research area.
Authors: Thank you for the comment. In the revised manuscript, we indicated that Fuzzy-logic and deep Q-learning are unsuitable for the formulated problem, which is closer to nonlinear regression than decision problems. Therefore deep learning based on neural network structures is more useful to our problem.
Re-comment: I think it will be challenging for the researchers. Please, highlight their weaknesses and advantages in a Table besides pointing out the role of the presented DRNN.
In methodology:
(17) Please, define and specify the input and output of the algorithm.
Authors: Thank you for the suggestion. In the revised manuscript, we specified the input as one historical O/D matrix, network structure, and traffic assignment assumption, whereas the output is a learning scheme that enables a subset of traffic sensor information to predict the corresponding O/D matrix.
Re-comment: The study discussed the algorithm where the authors have to clearly provide it. In a standard format of the algorithm, the inputs and output have to define on its main body, such as:
Inputs: x whereas 0 ≤ x ≤ 1
Output: Z whereas …
Pre-condition…
The authors can find the standard and technical format of the algorithm in robustness and valid related studies.
New comment: I think ResNet is a trained machine learning model and not described as an approach.
In numerical study:
New comment: Please, point Figure 9 out of the parenthesis.
Comments for author File: Comments.pdf
Author Response
Detailed responses to the reviewers' comments:
Our responses are presented in italic, bold font.
Reviewer 4:
I found the additional and recent studies. Nevertheless, some inessential and old works remain in the section. The authors can cure the level of the research study by removing them.
First, the authors would like to thank you for your deep and careful revision of our manuscript. We have tried to refine the reference by removing the outdated articles however, there are pioneer studies that should be referred to.
I think it will be challenging for the researchers. Please, highlight their weaknesses and advantages in a Table besides pointing out the role of the presented DRNN.
We agree with the review for his/her opinion. In the revised manuscript, we referred to an article for the required comparison.
The study discussed the algorithm where the authors have to clearly provide it. In a standard format of the algorithm, the inputs and output have to define on its main body, such as:
Inputs: x whereas 0 ≤ x ≤ 1
Output: Z whereas …
Pre-condition…
The authors can find the standard and technical format of the algorithm in robustness and valid related studies.
The input variables of link flow and the O/D demand as output are well-defined elements for both academic and fractionates in the field of transportation.
Please, point Figure 9 out of the parenthesis.
Thank you for your note in the revised manuscript. We have adjusted it accordingly.
At last, the authors would like to thank the Associate Editor and the reviewers for their constructive comments that have led to significant improvements in the quality of the paper."
Reviewer 5 Report
The authors have well addressed all my comments.
The authors have well addressed all my comments.
Author Response
Thanks for your valuable comments that helped the manuscript to reach its best quality.
Round 3
Reviewer 1 Report
Thank you for your revision.