The Prediction of Pectin Viscosity Using Machine Learning Based on Physical Characteristics—Case Study: Aglupectin HS-MR
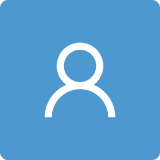
Round 1
Reviewer 1 Report
Comments and Suggestions for AuthorsDear Dr. Editor,
This paper showed a prediction of pectin viscosity using machine learning algorithms. Although this manuscript has a certain amount of work and some practical innovation, except presentation, some mistakes were found. Please find my comments in the following.
1) The main problem is that this work should focus on the diversity of the pectin samples selected for predictive models for pectin viscosity by using machine learning algorithms.
ü Please cancel the comparative analysis of the pectin samples with different concentration and PH.
The aim of different concentration and PH combined was to obtain the different viscosity of pectin samples and further to establish the predictive models for viscosity.
So, to present the pectin viscosity characteristics for learning models and add the viscosity values in table.
2) How much samples selected for learning and test dataset, respectively? Please give a defined number.
3) Line 143-145, “According to the issue, 6 predictive models were selected:1 Linear regression(LR),2 Decision Tree(DT), 3Random Forest(RF), 4Support Vector Machine(SVR), 5Bayesian, 6ElsaticNet,7 Huber regression (HR).
6 is ok? 7
4) Line 198-200,”These changes can be described by an exponential function of the type 𝜂 = 𝐴 ∗ 𝑒B*C, where c represents the concentration, while A and B are constants. This type of relationship is characteristic among others of hydrocolloids[38-40]
In table 2, A and B values, please add the calculated methods for A and B.
5)Conclusions
Line 342-345, “In the result of physical and rheological analyses, it was demonstrated that pectin solutions in aqueous buffers without any additive did not lead to a gel structure, even for extremely low pH. It was also demonstrated that pectin has a high shelf life over a long period of storage among others in domestic conditions”
Line 350-352, “estimation of pectin viscosity as a function of solution concentration and pH value significantly affected the learning performance of the proposed machine learning algorithms
These conclusion obtained were not strongly supported by the results presented in this work. Please rewrite the conclusion.
Author Response
Dear Reviewer 1,
thank you very much for your valuable comments. I have made the changes as you recommended. This certainly improved and strengthened the substantive aspects of my study.
Response to Reviewer 1 Comments:
Comments and Suggestions for Authors
Dear Dr. Editor,
This paper showed a prediction of pectin viscosity using machine learning algorithms. Although this manuscript has a certain amount of work and some practical innovation, except presentation, some mistakes were found. Please find my comments in the following.
1) The main problem is that this work should focus on the diversity of the pectin samples selected for predictive models for pectin viscosity by using machine learning algorithms.
Ü Please cancel the comparative analysis of the pectin samples with different concentration and PH.
The aim of different concentration and PH combined was to obtain the different viscosity of pectin samples and further to establish the predictive models for viscosity.
So, to present the pectin viscosity characteristics for learning models and add the viscosity values in table.
Figure 2 was prepared based on values obtained from actual viscosity tests performed according to the procedure outlined in Section 2.3 viscosity measurement. In order to enable analysis of viscosity values, both as a function of pH and concentration, we have inserted Table 3 into the text.
Table 3. Results of viscosity measurements with SD. The capital letters indicate statistical differences within the columns, lower case letters indicate statistical differences across rows (p<0.05)
C |
pH |
|||||
[%] |
3 |
4 |
5 |
6 |
7 |
8 |
1 |
0.596±0.159Ga |
0.699±0.147Fa |
0.831±0.090Ea |
0.887±0.194Ea |
0.812±0.132Ea |
0.665±0.104Ea |
2 |
0.763±0.840Ga |
0.858±0.183Fa |
0.915±0.049Ea |
1.025±0.498Ea |
0.626±0.188Ea |
0.585±0.109Ea |
3 |
1.512±0.436Fa |
1.220±0.197Fab |
1.153±0.080Eab |
1.004±0.191Eab |
0.635±0.129Eb |
0.641±0.037Eb |
4 |
2.694±0.399Ea |
2.095±0.284Ea |
2.682±0.296Da |
1.442±0.201Db |
0.733±0.208Ec |
0.746±0.138Ec |
5 |
4.044±0.127Da |
3.401±0.189Db |
2.292±0.218Dc |
1.961±0.106EDcd |
1.862±0.075Dcd |
1.498±0.071Dd |
6 |
7.676±0.149Ca |
5.301±0.530Cb |
3.656±0.125Cc |
3.337±0.079Ccd |
3.250±0.092Ccd |
2.934±0.207Cd |
7 |
13.928±0.159Ba |
9.866±0.766Bb |
6.047±0.252Bc |
6.164±0.063Bc |
5.537±0.161Bcd |
5.351±0.104Bd |
8 |
27.758±0.132Aa |
19.894±0.105Ab |
11.291±0.191Ac |
8.681±0.127Ae |
9.945±0.177Ad |
8.409±0.317Ae |
In addition, the authors, as recommended by the other reviewers, also clarified the necessary information in the aspect of attributes (input/output data) when designing predictive models. We included this content in the methodology.
2) How much samples selected for learning and test dataset, respectively? Please give a defined number.
In machine learning from experience when learning algorithms, we have method train-and-test (TandT). At the very first stage of action at the criterion during machine learning, in addition to the selection of models based on the amount of data, the method of learning is determined. For this research problem and the amount of collected data in the sets, learning with the train-and-test method is sufficient to prevent the model from over-fitting.
The choice of learning method, e.g. training and testing, translates into the fact that the data is divided into two sets of training and testing in a ratio of 70% to 30% (70:30). In Python, according to the specifics of the issue, it is performed using the train_test_test method, the so-called split sets. In the training and testing method, the process of selecting data randomly is carried out, i.e. the indexes of the learning cases are shuffled in a random order in order not to affect specific learning cases (i.e. with a given index) when learning models. It is important that the data partitioning is done by normalization or standardization so as not to get bad model quality metrics (model performance). In this work using Python, features were normalized using the StandardScaler tool.
The set contained 482 cases with the 70:30 ratio included in the article meaning that 337 cases belong to the teaching set and the rest to the test set (145 cases).
We explain it in lines: 127-142, but we also corrected into the text.
3) Line 143-145, “According to the issue, 6 predictive models were selected:1 Linear regression(LR),2 Decision Tree(DT), 3Random Forest(RF), 4Support Vector Machine(SVR), 5Bayesian, 6ElsaticNet,7 Huber regression (HR). 6 is ok? 7
We corrected into the text.
4) Line 198-200,”These changes can be described by an exponential function of the type ? = ? ∗ ?B*C, where c represents the concentration, while A and B are constants. This type of relationship is characteristic among others of hydrocolloids[38-40]
In table 2, A and B values, please add the calculated methods for A and B.
The authors thank you for your feedback and explain that the results in the methodology were described in table 2 using the least squares method.
5)Conclusions
Line 342-345, “In the result of physical and rheological analyses, it was demonstrated that pectin solutions in aqueous buffers without any additive did not lead to a gel structure, even for extremely low pH. It was also demonstrated that pectin has a high shelf life over a long period of storage among others in domestic conditions”
Physical and rheological analyses showed that pectin solutions in aqueous buffers without any additives did not lead to the formation of a gel structure, even at extremely low pH (pH=3). It was also shown that pectin has a high shelf life over a long period of storage, among other things, at home.
We corrected into the text.
Line 350-352, “estimation of pectin viscosity as a function of solution concentration and pH value significantly affected the learning performance of the proposed machine learning algorithms
The proposed machine learning algorithms successfully estimated pectin viscosity based on concentration, pH and L*a*b*.
We corrected into the text.
These conclusion obtained were not strongly supported by the results presented in this work. Please rewrite the conclusion.
We added information about numerical data and rewrite conclusion too. We corrected into the text.
Kind regards,
Krzysztof Przybył
Author Response File: Author Response.pdf
Reviewer 2 Report
Comments and Suggestions for AuthorsThe article presents an informative and insightful overview of the role of pectins in the food industry, emphasizing their natural origin, health benefits, and functional properties. It successfully highlights the increasing consumer preference for natural and minimally processed ingredients, making pectins a valuable addition to food products.
From the perspective of research content and methods, this paper adopts a scientifically rigorous research method, combining physical analysis and machine learning techniques, demonstrating the effective application of these two methods in the field of food science. The research results not only have high accuracy, but also provide a new perspective for predicting pectin viscosity, which has important guiding significance for food production and quality control.
However, there are also some potential areas for improvement in this paper. For example, In the part of Introduction, the research progress about prediction of pectin viscosity should be detailed described. Although decision trees and random forest models have achieved good results in predicting pectin viscosity, the paper did not delve into other possible machine learning models, such as neural networks, nor did it compare the advantages and disadvantages of different models. In addition, when explaining the prediction results and model performance, the article can delve deeper into the physical and chemical mechanisms behind them, in order to better understand and apply these prediction models.
Comments on the Quality of English LanguageThe article presents an informative and insightful overview of the role of pectins in the food industry, emphasizing their natural origin, health benefits, and functional properties. It successfully highlights the increasing consumer preference for natural and minimally processed ingredients, making pectins a valuable addition to food products.
From the perspective of research content and methods, this paper adopts a scientifically rigorous research method, combining physical analysis and machine learning techniques, demonstrating the effective application of these two methods in the field of food science. The research results not only have high accuracy, but also provide a new perspective for predicting pectin viscosity, which has important guiding significance for food production and quality control.
However, there are also some potential areas for improvement in this paper. For example, In the part of Introduction, the research progress about prediction of pectin viscosity should be detailed described. Although decision trees and random forest models have achieved good results in predicting pectin viscosity, the paper did not delve into other possible machine learning models, such as neural networks, nor did it compare the advantages and disadvantages of different models. In addition, when explaining the prediction results and model performance, the article can delve deeper into the physical and chemical mechanisms behind them, in order to better understand and apply these prediction models.
Author Response
Dear Reviewer 2,
thank you very much for your valuable comments. I have made the changes as you recommended. This certainly improved and strengthened the substantive aspects of my study.
Response to Reviewer 2 Comments:
Comments and Suggestions for Authors
The article presents an informative and insightful overview of the role of pectins in the food industry, emphasizing their natural origin, health benefits, and functional properties. It successfully highlights the increasing consumer preference for natural and minimally processed ingredients, making pectins a valuable addition to food products.
From the perspective of research content and methods, this paper adopts a scientifically rigorous research method, combining physical analysis and machine learning techniques, demonstrating the effective application of these two methods in the field of food science. The research results not only have high accuracy, but also provide a new perspective for predicting pectin viscosity, which has important guiding significance for food production and quality control.
Thank you for opinion.
However, there are also some potential areas for improvement in this paper. For example, In the part of Introduction, the research progress about prediction of pectin viscosity should be detailed described. Although decision trees and random forest models have achieved good results in predicting pectin viscosity, the paper did not delve into other possible machine learning models, such as neural networks, nor did it compare the advantages and disadvantages of different models. In addition, when explaining the prediction results and model performance, the article can delve deeper into the physical and chemical mechanisms behind them, in order to better understand and apply these prediction models.
We add information about the research progress about prediction of pectin viscosity. This was confirmed by studies on the prediction of pectin viscosity by atomic force microscopy, among others, and measurements of the viscosity of pectin solutions showing the high stability of pectin solutions (Ref1). Moreover, it demonstrated that rheological changes of potato pectin depend on factors such as: concentration, pH value, temperature and metal ions (Ref2).
Ref1 - Wang, H.; Fei, S.; Wang, Y.; Zan, L.; Zhu, J. Comparative Study on the Self-Assembly of Pectin and Alginate Molecules Regulated by Calcium Ions Investigated by Atomic Force Microscopy. Carbohydr Polym 2020, 231, 115673, doi:10.1016/j.carbpol.2019.115673.
Ref2 - Wang, W.; Yang, Y.; Zhang, H.; Liu, B.; Xu, T. Study on Rheological Properties of Potato Pulp Pectin. Food and Fermentation Industries 2021, 47, doi:10.13995/j.cnki.11-1802/ts.024285.
Considering the popularity of neural networks in machine learning, among other things, this time, however, the authors focused on those machine learning algorithms that are easier to implement in industry by, among other things, their simple structure and interpretability.
From experience, these types of models are chosen depending on the complexity of the dataset. Neural networks are worth using for more applied data in the input with images (CNN) or text (NLP), among others. Moreover, algorithms such as: Random Forest or Decision Tree offers resistance to data overfitting, especially when dealing with a smaller amount of data in the set.
We corrected into the text.
Kind regards,
Krzysztof Przybył
Author Response File: Author Response.pdf
Reviewer 3 Report
Comments and Suggestions for AuthorsGENERAL COMMENT
The manuscript presents an interesting topic focused on the food area. The authors approached the problem from an industrial point of view, where its simulation could be used as a method to know the viscosity from the physicochemical characteristics. However, is this really feasible for the industry? That is, if you need to know at least two parameters to estimate viscosity, wouldn't the industry be better off calculating viscosity directly?
SPECIFIC COMMENTS
The methodology of the work leaves many scientific gaps, for example, what mathematical model did they use in their predictions? Or simply the different analyzes were carried out based on the results of the equalization shown in L199.
Considering the title and scope shown in the introduction, it seems to me that making the prediction with a single pectin does not allow it to be a standardized prediction, it is simply a prediction for the Agglupectin HS-MR pectin.
For an original work, it is inappropriate to use 32 references to organize a one-page introduction and only 15 for a discussion that aims to validate or standardize its proposal as a technological development.
Author Response
Dear Reviewer 3,
thank you very much for your valuable comments. I have made the changes as you recommended. This certainly improved and strengthened the substantive aspects of my study.
Response to Reviewer 3 Comments:
Comments and Suggestions for Authors
GENERAL COMMENT
The manuscript presents an interesting topic focused on the food area. The authors approached the problem from an industrial point of view, where its simulation could be used as a method to know the viscosity from the physicochemical characteristics. However, is this really feasible for the industry? That is, if you need to know at least two parameters to estimate viscosity, wouldn't the industry be better off calculating viscosity directly?
In the study, the authors clearly defined the number of input variables in the methodology and the dataset contained 6 attributes informing about the physical and rheological properties of pectin, i.e. concentration, pH, color parameters (L*, a*, b*) and value of average viscosity pectin. The authors specified that the decision variable in this research aspect was the value of average pectin viscosity.
Moreover, the authors as suggested by reviewer 4 discussed in the conclusion that the application of machine learning leads to optimization of the current pectin quality control process, which will translate into waste control, among other things. Machine learning can lead to the development of new food product formulations (i.e., food gelation) by controlling optimal conditions for selected physical parameters.
We corrected into the text.
SPECIFIC COMMENTS
The methodology of the work leaves many scientific gaps, for example, what mathematical model did they use in their predictions? Or simply the different analyzes were carried out based on the results of the equalization shown in L199.
On the basis of the results of viscosity measurements for different pH (3-8 with the step of 1) and concentrations (1-8% with 1% step), we have performed nonlinear regression for the viscosity value changes as a function of concentration for each pH separately, using three different model equations according to Marcotte at al. (Marcotte, M.; Taherian Hoshahili, A.R.; Ramaswamy, H.S. Rheological Properties of Selected Hydrocolloids as a Function of Concentration and Temperature. Food Research International 2001, 34, 695–703, doi:10.1016/S0963-9969(01)00091-6). The models used were: power, exponential and polynomial. The best fit was with the exponential model (Figure 1).
We have introduced additional information to the text (Materials and methods section).
Considering the title and scope shown in the introduction, it seems to me that making the prediction with a single pectin does not allow it to be a standardized prediction, it is simply a prediction for the Agglupectin HS-MR pectin.
The authors agree with the reviewer's opinion. The authors added information in the title and the material and methods section explaining that the pectin is Aglupectin HS-MR.
We corrected into the text.
For an original work, it is inappropriate to use 32 references to organize a one-page introduction and only 15 for a discussion that aims to validate or standardize its proposal as a technological development.
The authors agree with the reviewer's opinion. We added references in result and discussion. We corrected into the text.
Kind regards,
Krzysztof Przybył
Author Response File: Author Response.pdf
Reviewer 4 Report
Comments and Suggestions for AuthorsThe manuscript "The prediction of pectin viscosity using machine learning based on physical characteristics" presents an innovative proposal by proposing the use of artificial intelligence as a tool in the quality control of food production in order to minimize waste.
Below are my considerations:
- Figure 2 is illegible due to its small size.
- In tables 3 to 5, set as a footer explaining that the same letters in the columns do not represent statistically significant differences.
- Lines 334-335: "assessed visually no degradation of the solutions or mold formation was observed during 1 week of storage under home refrigeration”. It would be interesting to carry out microbiological stability tests to consolidate this information.
- Linhas 356-358: “Modeling the physical parameters of pectin using machine learning could potentially lead to the development of new recipes for food products...”. Explain what these new recipes are. Exemplify.
- When discussing the results, I suggest comparing them with other studies in the scientific literature.
- In the conclusion, I suggest you add information on how artificial intelligence can contribute to waste control through this study.
Author Response
Dear Reviewer 4,
thank you very much for your valuable comments. I have made the changes as you recommended. This certainly improved and strengthened the substantive aspects of my study.
Response to Reviewer 4 Comments:
Comments and Suggestions for Authors
The manuscript "The prediction of pectin viscosity using machine learning based on physical characteristics" presents an innovative proposal by proposing the use of artificial intelligence as a tool in the quality control of food production in order to minimize waste.
Below are my considerations:
- Figure 2 is illegible due to its small size.
Figure 2 is readable due to its 300dpi size. However, to improve the quality of the image it was created in 600dpi resolution.
We corrected into the text.
- In tables 3 to 5, set as a footer explaining that the same letters in the columns do not represent statistically significant differences.
We corrected into the text.
- Lines 334-335: "assessed visually no degradation of the solutions or mold formation was observed during 1 week of storage under home refrigeration”. It would be interesting to carry out microbiological stability tests to consolidate this information.
Thank you for your opinion. In the future, the authors will also take this aspect into account. Nevertheless, looking at the various applications and behaviors of pectin post-mediately, they can contribute to the understanding of their stability in various aspects, including high methoxyl pectin (PPOP) showed greater dilution at higher shear rates [Ref1], pectin-rich acid extract from onion flesh showed stable emulsions with good stability before creaming [Ref2], or pectin is used as gelling, thickening and stabilizing agents in the food industry [Ref 3].
Ref1 - Eliana Noemí Fissore; Basanta, F.; Calvache, J.E.N.; Ana María Rojas; Gerschenson, L.N. Chemical Composition and Rheological Behaviour of Pectins from Unconventional Sources. In Pectin: Chemical Properties, Uses and Health Benefits; 2014.
Ref2 - Neckebroeck, B.; Verkempinck, S.H.E.; Bernaerts, T.; Verheyen, D.; Hendrickx, M.E.; Van Loey, A.M. Investigating the Role of the Different Molar Mass Fractions of a Pectin Rich Extract from Onion towards Its Emulsifying and Emulsion Stabilizing Potential. Food Hydrocoll 2021, 117, 106735, doi:10.1016/j.foodhyd.2021.106735.
Ref 3- Colodel, C.; Vriesmann, L.C.; Lucia de Oliveira Petkowicz, C. Rheological Characterization of a Pectin Extracted from Ponkan (Citrus Reticulata Blanco Cv. Ponkan) Peel. Food Hydrocoll 2019, 94, 326–332, doi:10.1016/j.foodhyd.2019.03.025.
- Linhas 356-358: “Modeling the physical parameters of pectin using machine learning could potentially lead to the development of new recipes for food products...”. Explain what these new recipes are. Exemplify.
Applying modeling of physical parameters through concentration, pH and L*,a*b*, it becomes possible to influence pectin viscosity. The application of machine learning, one is led to optimize the current pectin quality control process, which will translate into among other things, for waste control. Machine learning can lead to the development of new food product formulations (i.e., food gelation) by controlling optimal conditions for selected physical parameters [Ref4].
Ref4 - Kamal, Md.M.; Akhtaruzzaman, Md.; Sharmin, T.; Rahman, M.; Mondal, S.C. Optimization of Extraction Parameters for Pectin from Guava Pomace Using Response Surface Methodology. J Agric Food Res 2023, 11, 100530, doi:10.1016/j.jafr.2023.100530
We corrected into the text.
- When discussing the results, I suggest comparing them with other studies in the scientific literature.
We added references as reviewer 3 suggested too. We corrected into the text.
- In the conclusion, I suggest you add information on how artificial intelligence can contribute to waste control through this study.
The application of machine learning, one is led to optimize the current pectin quality control process, which will translate into among other things, for waste control [Ref5].
Ref5 - Cha, G.-W.; Moon, H.J.; Kim, Y.-C. A Hybrid Machine-Learning Model for Predicting the Waste Generation Rate of Building Demolition Projects. J Clean Prod 2022, 375, 134096, doi:10.1016/j.jclepro.2022.134096.
We corrected into the text.
Kind regards,
Krzysztof Przybył
Author Response File: Author Response.pdf
Reviewer 5 Report
Comments and Suggestions for AuthorsIn the study “the prediction of pectin viscosity using machine learning based on physical characteristics” the authors developed a new approach using machine learning algorithms for investigating the viscosity of pectin. The researchers used the pectin concentration, pH conditions, and the color of the pectin solutions as parameters to train a number of predictive models. The authors reported that out of the 6 predictive models they used to predict the viscosity of pectin, Decision Tree and Random Forest models were the best as they had the highest values of R2 and minimal MSE error.
There is just one thing in the methods that should be explained. The viscosity measurements were done at a 2.6 Hz frequency, why 2.6 Hz was chosen, and were the measurements done within the linear viscoelastic range? If so, what was the strain (%)?
Author Response
Dear Reviewer 5,
thank you very much for your valuable comments. I have made the changes as you recommended. This certainly improved and strengthened the substantive aspects of my study.
Response to Reviewer 5 Comments:
Comments and Suggestions for Authors
In the study “the prediction of pectin viscosity using machine learning based on physical characteristics” the authors developed a new approach using machine learning algorithms for investigating the viscosity of pectin. The researchers used the pectin concentration, pH conditions, and the color of the pectin solutions as parameters to train a number of predictive models. The authors reported that out of the 6 predictive models they used to predict the viscosity of pectin, Decision Tree and Random Forest models were the best as they had the highest values of R2 and minimal MSE error.
There is just one thing in the methods that should be explained. The viscosity measurements were done at a 2.6 Hz frequency, why 2.6 Hz was chosen, and were the measurements done within the linear viscoelastic range? If so, what was the strain (%)?
The Dynamic Mechanical Thermal Analyzer DMWT (COBRABiD - Poznań, Poland), which operates on the basis of the free oscillation fading technique, was used to perform the measurements. Therefore, it is not possible to choose any frequency (as is the case when using the forced oscillation technique). The oscillation frequency of 2.6 Hz used makes it possible to determine the rheological properties of the tested systems (weakly cross-linked) over the entire range of concentrations used. The deformation (strain) was 0.8%.
We corrected into the text.
Kind regards,
Krzysztof Przybył
Author Response File: Author Response.pdf
Round 2
Reviewer 1 Report
Comments and Suggestions for AuthorsDear Dr. Editor
Overall the quality of this work is still not good, and hasn’t improved significantly.
1) Please present the pectin viscosity characteristics for learning models and validation set.
2) Please delete Fig3
3) 482 learning case is ok?
4) In Fig.4, please separate 7 validation scatter plot , it is difficult to figure out
Comments on the Quality of English Language
English language need be improved
Author Response
Dear Reviewer 1,
thank you very much for your valuable comments. I have made the changes as you recommended. This certainly improved and strengthened the substantive aspects of my study.
Response to Reviewer 1 Comments:
Overall the quality of this work is still not good, and hasn’t improved significantly.
- Please present the pectin viscosity characteristics for learning models and validation set.
The set contained a total of 482 teaching cases, with a ratio of 70:30 included in the article. This means that 337 cases belong to the training set and the rest to the test set (145 cases). We have improved the style of the language. We corrected into the text. Moreover, the validation set is used only when the need to tune the hyperparameters of the models is required. Currently and having experience in the context of the behavior of selected machine learning algorithms from previous studies, this procedure was not required. Thank You for your opinion.
2) Please delete Fig3
We remove figure 3. We have corrected in the text.
3) 482 learning case is ok?
Yes. It’s ok. We explained that this is the entire dataset consists of a learning set and a test set.
4) In Fig.4, please separate 7 validation scatter plot , it is difficult to figure out
The other reviewers didn't have comments on the drawing before and don't have comments now either. The figure is vectorized and very clear. The authors also believe figure is clear. However, they tried to separate the elements in the drawing, the effect was much worse, we leave unchanged. Thank You for your opinion.
- English language corrected.
Kind regards,
Krzysztof Przybył
Author Response File: Author Response.pdf
Reviewer 2 Report
Comments and Suggestions for AuthorsIt has been well modified, and the current one can be accepted for publication.
Author Response
Dear Reviewer 2,
thank you very much for your valuable comments. I have made the changes as you recommended. This certainly improved and strengthened the substantive aspects of my study.
Response to Reviewer 2 Comments:
It has been well modified, and the current one can be accepted for publication.
Thank You for your opinion.
Kind regards,
Krzysztof Przybył
Author Response File: Author Response.pdf
Reviewer 3 Report
Comments and Suggestions for AuthorsSPECIFIC COMMENTS
L191. It is not necessary to justify the use of scheduling software with 3 appointments.
L196. It is not necessary to justify the use of your significance level with a citation.
L198-201. The exponential function is not part of a result, but of the methodology. Therefore, it should not go in that section. The authors must find a way to describe its behavior without placing it.
L213-214. Why, if this function is typical of colloids, did the authors use others in their methodology? Why not just use it directly?
Table 1. What test for the analysis of means did you use for your experimental results?
L242. The authors used 4 quotes to express those other studies also found statistical changes, surely there are many others where they saw these variations or even studies where there were no variations... however, the analysis has to be deeper... that is, why those 4 studies had statistical changes? What did they do differently or what did they do the same?
Table 4. What test for the analysis of means did you use for your experimental results?
Table 5. What test for the analysis of means did you use for your experimental results?
Table 6. What test for the analysis of means did you use for your experimental results?
Tables 4-6. They can easily be grouped into one.
L301-305. What do neural networks have to do with the study of viscosity?
L305-306Review the information because 6 citations for information that can be given in the introduction (does not justify results) is too much.
Conclusions. Conclusions NEVER carry citations.
References. The authors did not reduce the number of citations in the introduction and used an excessive number of references (up to 5) to "endorse" very basic and unnecessary information.
Author Response
Dear Reviewer 3,
thank you very much for your valuable comments. I have made the changes as you recommended. This certainly improved and strengthened the substantive aspects of my study.
Response to Reviewer 3 Comments:
SPECIFIC COMMENTS
L191. It is not necessary to justify the use of scheduling software with 3 appointments.
The authors removed unnecessary sentence and packages. They added information about the version of each package. We have corrected the text.
L196. It is not necessary to justify the use of your significance level with a citation.
The authors removed citation. We have corrected the text.
L198-201. The exponential function is not part of a result, but of the methodology. Therefore, it should not go in that section. The authors must find a way to describe its behavior without placing it.
The presented viscosity values in results section are experimental results and served as basic information for learning models implemented. In the methodology we have presented three most common equations describing typical behavior of hydrocolloids in model systems. In the results section we have mentioned the best fit of experimental results to one of those simple type of equations. Therefore, we believe that those results are essential for the manuscript and fitting provides additional information.
L213-214. Why, if this function is typical of colloids, did the authors use others in their methodology? Why not just use it directly?
From the literature follows that there are several model equations to describe the changes of viscosity as a function of concentration, which fit differently for different types of colloids. In Materials and Methods section we have mentioned three are the most popular and the simplest of them (power, exponential and polynomial), and fitted them as a starting point of the learning procedure. The essential conclusion from the initial fitting is that exponential model fit was the best for our samples.
Table 1. What test for the analysis of means did you use for your experimental results?
The authors averaged the output variable in the data analysis to help reduce noise in the data from the set while giving precise relationships between the variables. Averaging the output variable also stabilizes the results to produce a consistent predictive model. Moreover, averaging the output variable minimizes the impact of receiving outliers through the data on the model. Although few authors in literature use average. Nevertheless, we will take this suggestion into account in the future to obtain only the most recent solutions.
L242. The authors used 4 quotes to express those other studies also found statistical changes, surely there are many others where they saw these variations or even studies where there were no variations... however, the analysis has to be deeper... that is, why those 4 studies had statistical changes? What did they do differently or what did they do the same?
1. In the literature, the team of Gamm et al. 2020 also focused on studying the difference between probability distributions of L*a*b* color measurements, but proposed a metric called Threshold for Color Difference Discrimination (TCDD) in units of ΔE. They applied Hotelling's T2 test to the color measurement of color coatings focused, among other things, on evaluating the precision of outlier detection within color reproduction. In this research, the Tukey Test was chosen to analyze the differences in L*a*b color for pectin between their concentration ranges and pH values. - Gamm, B.M.; Gerard, P. Testing the Differences between Two Color Measurement Probability Distributions Using Hotelling’s T 2 Test and the Permutation Test. Color Res Appl 2020, 45, 196–207, doi:10.1002/col.22468.
2. Badaró's team et al. 2020 focused their research on the hyperspectral imaging technique, which is classified based on orange peels according to their pectin content. They focused on Principal components analysis (PCA) and Linear discriminant analysis (LDA) techniques to separate the group of suppliers of different pectins into three classes of primary, intermediate and external pectin content. The team's research results from the hypothesis that NIR-HSI may have a quantitative impact on the pectin content in orange peels. The literature has concluded that this technique has potential as an alternative to colorimetric methods and for the quantification of pectin in orange peels and for categorizing orange peel into a group with a pectin concentration driver.
r Badaró, A.T.; Garcia-Martin, J.F.; López-Barrera, M. del C.; Barbin, D.F.; Alvarez-Mateos, P. Determination of Pectin Content in Orange Peels by near Infrared Hyperspectral Imaging. Food Chem 2020, 323, 126861, doi:10.1016/j.foodchem.2020.126861.
3. The team of Zhu et al. 2016, as part of their analysis of peach fruit, conducted research on determining color differences with pectin distribution as well as fruit maturity. They used hyperspectral imaging providing a promising alternative, enabling visualization of pectin distribution at the pixel level. - Zhu, N.; Huang, W.; Wu, D.; Chen, K.; He, Y. Quantitative Visualization of Pectin Distribution Maps of Peach Fruits. Sci Rep 2017, 7, 9275, doi:10.1038/s41598-017-09817-7.
We corrected into the text.
Table 4. What test for the analysis of means did you use for your experimental results?
An analysis of variance (ANOVA) was conducted to compare means between pairs of L*a*b* color space model groups. Tukey’s test was applied with level of importance of p = 0.05. We have corrected the text.
Table 5. What test for the analysis of means did you use for your experimental results?
An analysis of variance (ANOVA) was conducted to compare means between pairs of L*a*b* color space model groups. Tukey’s test was applied with level of importance of p = 0.05. We have corrected the text.
Table 6. What test for the analysis of means did you use for your experimental results?
An analysis of variance (ANOVA) was conducted to compare means between pairs of L*a*b* color space model groups. Tukey’s test was applied with level of importance of p = 0.05. We have corrected the text.
Tables 4-6. They can easily be grouped into one.
We have corrected the text.
L301-305. What do neural networks have to do with the study of viscosity?
The authors use the literature as an example to provide a key explanatory argument that artificial neural networks (SNNs) have shown greater accuracy in predicting viscosity compared to traditional correlations and empirical models.
• Bilodeau, C., Kazakov, A., Mukhopadhyay, S., Emerson, J., Kalantar, T., Muzny, C., & Jensen, K. (2023). Machine learning for predicting the viscosity of binary liquid mixtures. Chemical Engineering Journal, 464, 142454. https://doi.org/10.1016/j.cej.2023.142454
• Chen, Z., Wang, M., Meng, Z., Wang, H., Liu, L., & Wang, X. (2021). Development of structure-informed artificial neural network for accurately modeling viscosity of multicomponent molten slags. Ceramics International, 47(21), 30691–30701. https://doi.org/10.1016/j.ceramint.2021.07.248
• Chen, Z., & Yang, D. (2021). Predicting Viscosities of Heavy Oils and Solvent–Heavy Oil Mixtures Using Artificial Neural Networks. Journal of Energy Resources Technology, 143(11). https://doi.org/10.1115/1.4049603
• Maleki, E., Sadrhosseini, H., & Ghiami, A. (2015). Investigation of Artificial Neural Networks Capability to Predict Viscosity of Lubricants Used in Journal Bearings. Proceedings of the The International Conference on Engineering & MIS 2015, 24-26-September-2015, 1–7. https://doi.org/10.1145/2832987.2833019
L305-306 Review the information because 6 citations for information that can be given in the introduction (does not justify results) is too much.
We have corrected the text.
Conclusions. Conclusions NEVER carry citations.
We have corrected the text.
References. The authors did not reduce the number of citations in the introduction and used an excessive number of references (up to 5) to "endorse" very basic and unnecessary information.
The authors removed citations. We have corrected the text.
Kind regards,
Krzysztof Przybył
Author Response File: Author Response.pdf
Round 3
Reviewer 3 Report
Comments and Suggestions for AuthorsThe manuscript improved compared to the first version, however, in my opinion it still does not meet the quality that Sustainability works on. For example, there is repeated information in tables and figures, the analysis is based on the general phenomena that occur in the samples and not on the differences in their treatments. On the other hand, the authors did not prepare a correct revision response, since they kept revisions from the previous version in the manuscript and this generated a loss of time to identify which were from the last revision and which from the last one. It seems to me that this version 3 is the one with which the submission should have started (since we corrected many basic errors that should not have been made) and from there the improvements should have begun.
Author Response
Dear Reviewer 3,
thank you very much for your valuable comments. We have made the changes as you recommended. This certainly improved and strengthened the substantive aspects of my study.
Response to Reviewer 3 Comments:
The manuscript improved compared to the first version, however, in my opinion it still does not meet the quality that Sustainability works on. For example, there is repeated information in tables and figures, the analysis is based on the general phenomena that occur in the samples and not on the differences in their treatments. On the other hand, the authors did not prepare a correct revision response, since they kept revisions from the previous version in the manuscript and this generated a loss of time to identify which were from the last revision and which from the last one. It seems to me that this version 3 is the one with which the submission should have started (since we corrected many basic errors that should not have been made) and from there the improvements should have begun.
In the result of the 1st round of review, the authors revised the manuscript according to the comments of 5 reviewers. In the second round, the authors, also made appropriate corrections as expected by 3 reviewers, including changes in tables and figures. However, the authors also added information in the manuscript as to exactly which tables were performed with the aforementioned Tukey test, in response to an inquiry about the Round 2 tables from the third reviewer. We corrected into the text. Regarding the viscosity figures, the authors explicitly suggested to the comments of the other reviewers as well, which translated into the creation of similar additional information related to the with the table and figure.
The authors believe that they have made corrections in accordance with the comments of all reviewers. The corrections from the previous version were retained because they were accepted by the earlier reviewers, which translates into the changes made from the first round as well as the second round of reviews. Normally, the editors approve all versions in the last phase, which has been done so far. We point out that the file has a change tracking option and changes from both the first and second rounds of reviews are made, without conflicting within the transparency of content changes.
Nevertheless, the authors have made every effort to respond to the reviewer's comments.
Kind regards,
Krzysztof Przybył
Author Response File: Author Response.pdf