Investigating Factors Influencing Crash Severity on Mountainous Two-Lane Roads: Machine Learning Versus Statistical Models
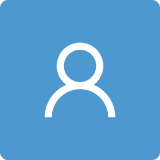
Round 1
Reviewer 1 Report
Comments and Suggestions for AuthorsThis study employs a range of statistical and machine learning models to examine the severity of traffic accidents on mountainous roads. It evaluates the performance of various methods in predicting accident severity and ranks the contributing factors using multiple models. An in-depth analysis of the impact on accident severity is carried out using XGBoost and SHAP methods. The research workload is substantial, the technical approach is well-constructed.
Recommendations for Revision:
- Supporting References for Table 4: Table 4 lacks supporting references; please provide them.
- Addressing Data Imbalance with SMOTE: While SMOTE is effective for resolving the issue of imbalanced distribution across different accident severity levels, this issue is also present among the factors influencing the study. For example, Table 6 indicates that the accident pattern includes up to ten categories with highly imbalanced distributions. Although it is challenging to apply SMOTE to each factor, it is advisable to merge certain levels of significant factors to improve data distribution. For instance, 'Collision with fixed object' and 'Collision with stationary vehicle' could be combined.
- Clarification on Data Source: There is a point of confusion regarding the data used in this study, as the paper states that the accidents occurred on two-way, two-lane mountainous roads. It is unclear whether these roads are basic segments or if they include both basic segments and intersections, particularly since side collisions, which mainly occur at intersections, are present in the dataset. Please provide a clear explanation.
- Comparison with Existing Studies: In Section 6, it is recommended to include comparisons with and support from existing studies regarding the influencing effects to enhance the reliability of the research findings.
- Update References: Most of the references are over three years old, with few recent studies from the past three years. Please supplement with more up-to-date research.
Some other tips:
- Enlarge and Enhance Figure 1: Figure 1 is relatively small; consider enlarging it and improving its resolution.
- Improve Resolution of Figures 5, 7-10: Figures 5, and 7-10 currently have low resolution, which does not meet publication standards. Please enlarge these figures and improve their resolution accordingly.
No suggestions
Author Response
Thank you for your thorough review. Specific responses to the comments are included in the attached document.
Author Response File: Author Response.pdf
Reviewer 2 Report
Comments and Suggestions for AuthorsThis paper presents a comparative analysis of six machine learning models and three statistical models' performance in modeling traffic crashes on mountainous highways. It looks well-presented but I have some comments.
1. the paper title is not academic. How to understand crash severity on various mountainous road types? This manuscript investigates which mountainous road types? These are not seen in the abstract and manuscript. The authors lack a rigorous academic attitude.
2. the conciseness of the abstract is poor and redundant. It is hard to understand which research gap this study filled. Some long sentences are very boring, such as “To select a more superior model and more accurately reveal the impact of key factors such as accident type and road conditions on accident severity, this paper focuses on two-way, two-lane mountain roads in Yunnan Province, China. It compares three statistical models (Ordered Logit, Partial Proportional Odds Model, and Multinomial Logit) and six machine learning models (Decision Tree, Random Forest, Gradient Boosting, Extra Trees, AdaBoost, and XGBoost) in terms of their performance in predicting accident severity and analyzing accident causes.” The fact that the accuracy of machine learning is more than statistics is common sense. In the abstract, some words are also puzzling, such as “two-way, two-lane mountain roads” and “mountainous areas”, “accident type” and “accident pattern”. Overall, the writing of the abstract is discursive.
3. In the introduction, the authors claim that "in the analysis of the causes of mountain traffic accidents, studies that comprehensively compare the accuracy and causative analysis results of statistical models and machine learning models are relatively rare. Therefore, it is currently unclear which model is more suitable for analyzing traffic accidents on mountain roads". This statement is currently unsupported by enough references, making it appear baseless and weak. Some helpful references are listed below. Many studies have already explored the accuracy and interpretability of statistical methods and machine learning algorithms on other roads. Therefore, this study just conducted similar research using different crash datasets and offered little new insight.
4. In the methodology section, details of SMOTE technology are lacking. For example, why SMOTE technology is necessary? How it works in your study. Meanwhile, the authors list the use of SMOTE for data resampling as one of the contributions to this study. This contribution is weak. First, SMOTE is one of the most popular technologies for handling imbalanced datasets, the authors do not make themselves improvements. Second, there are a few common methods to address imbalanced dataset issues, why did you choose SMOTE? Maybe another one is more suitable? Third, in the data profile section (due to the wrong page number in this manuscript, I do not what page number it is), lines 277-278, the authors state that “In the dataset, there is a noticeable imbalance in the distribution of accident severity levels.” I do not know if this is a hypothesis or a fact. If this is a fact, it is necessary to present obvious pieces of evidence to support that your dataset is imbalanced? Additionally, I have a question about whether machine learning technology is not effective for imbalanced datasets. In fact, many real datasets are imbalanced. For statistics, if there are many zero samples in datasets, you can apply this Zero expansion Poisson model which is a usual method in accident data modelling.
5. In the methodology section, this study utilizes and compares various statistical and machine learning models. However, the selected models are rather basic and commonly used, lacking significant innovation. In Table 2, the formulation for the Ordered Logit Model is the same as the Partial Proportional Odds Model. Are there any distinctions between them? In addition, the Ordered Models and Multicategory Models are usually suitable for different dependent variables. In the Ordered Models, the dependent variable is degree ranking and categorical. But in the Multicategory Model, it just requests that the dependent variable be categorical. Here, I have a question. For the same dataset and the same dependent variable, why can two different models be compared?
6. For machine learning methods, this manuscript indicated the XGBoost model is the best. It should be noted that the accuracy of models is related to the dataset and hyperparameter combination. For example, the Random Forest with the optimal hyperparameter combination may perform better than the Xgboost model. In other words, the reliability of the conclusion that XGBoost outperformed other machine learning models needs to be further discussed in another accident dataset of Mountainous two-lane roads.
7. In Table 6, the accident pattern (AP) is beyond comprehension. As a category variable, it has 10 types, which is beyond other variables. Another question, in your model, is why all independent variables are categorical variables. The continuous variable is lacking, which could influence the model's accuracy.
8. This paper considered eight independent variables, including weather (WR), accident pattern (AP), road surface condition (RSC), collision vehicle type (CVT), time (TE), road alignment (RA), vertical curve type (VCT), and holiday (HY). Some very important variables related to traffic flow are missing, such as vehicle speed when the crash happened and flow volume on the mountain road. This limits the contributions of your study.
9. The discussion in Section 6.3 lacks rationality and it is enough clear. Therefore, it is difficult to follow them.
10. Why the title of the final section is named “Conclusion and Outlook”. It really makes readers feel strange.
Author Response
Thank you for your thorough review. Specific responses to the comments are included in the attached document.
Author Response File: Author Response.pdf
Reviewer 3 Report
Comments and Suggestions for AuthorsThank you for your work. Please see my comments in the attached file.
Comments for author File: Comments.pdf
Author Response
Thank you for your thorough review. Specific responses to the comments are included in the attached document.
Author Response File: Author Response.pdf
Round 2
Reviewer 3 Report
Comments and Suggestions for AuthorsThe authors have addressed all the comments and suggestions I provided. The article, in my opinion, is ready for publication.