Classification of Southern Corn Rust Severity Based on Leaf-Level Hyperspectral Data Collected under Solar Illumination
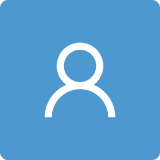
Round 1
Reviewer 1 Report
The topic and methodologies of the paper is extremely interesting. Here you can find my comments:
OVERALL: Please produce HD figures and enlarge them and all font sizes. The reader must be properly guided in the understanding of all sections
INTRODUCTION: Please, consider in the scientific background of your study also the most advanced statistical mtehods for geosciences applications (i.e.,
Albarracín, J.F.H.; Oliveira, R.S.; Hirota, M.; dos Santos, J.A.; Torres, R.d.S. A Soft Computing Approach for Selecting and Combining Spectral Bands. Remote Sens. 2020, 12, 2267. https://doi.org/10.3390/rs12142267
Fu, Y., Yang, G., Wang, J., & Feng, H. (2013). A comparative analysis of spectral vegetation indices to estimate crop leaf area index. Intelligent Automation & Soft Computing, 19(3), 315-326. https://doi.org/10.1080/10798587.2013.824176.
Lama, G.F.C.; Sadeghifar, T.; Azad, M.T.; Sihag, P.; Kisi, O. On the Indirect Estimation of Wind Wave Heights over the Southern Coasts of Caspian Sea: A Comparative Analysis. Water 2022 4, 843. https://doi.org/10.3390/w1406084.
Sarhadi, A., Burn, D. H., Johnson, F., Mehrotra, R., & Sharma, A. (2016). Water resources climate change projections using supervised nonlinear and multivariate soft computing techniques. Journal of Hydrology, 536, 119-132. https://doi.org/10.1016/j.jhydrol.2016.02.040.
Pham, Q. B., Mohammadpour, R., Linh, N. T. T., Mohajane, M., Pourjasem, A., Sammen, S. S., ... & Nam, V. T. (2021). Application of soft computing to predict water quality in wetland. Environmental Science and Pollution Research, 28(1), 185-200. https://doi.org/10.1007/s11356-020-10344-8.
Arabameri, A.; Blaschke, T.; Pradhan, B.; Pourghasemi, H.R.; Tiefenbacher, J.P.; Bui, D.T. Evaluation of Recent Advanced Soft Computing Techniques for Gully Erosion Susceptibility Mapping: A Comparative Study. Sensors 2020, 20, 335. https://doi.org/10.3390/s20020335.
Esposito, M., Crimaldi, M., Cirillo, V., Sarghini, Maggio, F., A. Drone and sensor technology for sustainable weed management: a review. Chem. Biol. Technol. Agric. 8, 18. https://doi.org/10.1186/s40538-021-00217-8)
I will reconsider the article after major revision.
Author Response
Please see the attachment.Author Response File: Author Response.pdf
Reviewer 2 Report
General comments:
This paper mainly explored using hyperspectral data to classify southern corn rust severity. Specifically, this paper collected leaf-level hyperspectral data within the experimental field, conducted ANOVA, and built RF models to classify the severity of SCR. In general, this paper is well organized and the results presented are clear. However, some details are missing or wrong and need to be addressed. This research needs to respond to concerns prior to any publication. The major concerns are as follows.
1. Section 2.3, how the percentage of the uredinium coverage is determined? Is it determined by manual estimation or calculated by some computer vision methods?
2. Table 2, when selecting samples from different groups, how the number of samples is determined? Why there are different numbers of samples from different groups, and will this bias the results?
3. Section 2.6.2, is the training-testing data split randomly?
4. Figure 6, since sub-figure c is to compare different leaf morphology, the suggestion is to separate c into several sub-figures and each sub-figure only contains one severity. The current one is too busy.
5. Section 3.3.1, "No grey box exited..." => "No grey box existed..."
6. Section 3.3.2, "purple boxes", the color appears to me is "magenta". Suggest changing colormap to sequential colormap.
7. Figure 10, suggest deleting the left two sub-figures in the last row.
8. Section 3.4, "Based on ANOVA test results, SI, RENDVI, PRI, LRDSI, MTCI, and SRI were selected for random forest classification.", what criteria are adopted here?
9. Section 4.2, "i.e., NDVI", there is no NDVI throughout the paper except the "RENDVI".
10. Section 4.4, why was the loss function not converging and the relative lower performance caused by a small sample size? Why there are 30 hidden layers? What are the inputs for the DNN model? Suggest removing the discussion about the DNN exploration if no details are provided.
11. Give the full name of "GF-6".
12. For the classification, besides the overall accuracy, it is better to include the confusion matrix to help understand the classification model performance.
Author Response
Please see the attachment
Author Response File: Author Response.pdf
Reviewer 3 Report
Please find my comments in attachment
Comments for author File: Comments.pdf
Author Response
Please see the attachment
Author Response File: Author Response.pdf
Round 2
Reviewer 1 Report
The authors were able to improve the scientific quality of the study. Just few issues must be fixed.
INTRODUCTION: Please, consider the importance of image processing in the monitoring of natural reosurces broadly speaking (i.e.,
Sadeghifar, T., Lama, G.F.C., Sihag, P., Bayram, A., Kisi, O. 2022. Wave height predictions in complex sea flows through soft computing models: Case study of Persian gulf. Ocean Eng. 245, 110467. https://doi.org/10.1016/j.oceaneng.2021.110467.
Dal Sasso, S.F., Pizarro, A., Samela, C., Mita, L., Manfreda, S. 2018. Exploring the optimal experimental setup for surface flow velocity measurements using PTV. Environ. Monit. Assess. 190, 460. https://doi.org/10.1007/s10661-018-6848-3.
Esposito, M., Crimaldi, M., Cirillo, V., Sarghini, F., Maggio, A. 2021. Drone and sensor technology for sustainable weed management: a review. Chem. Biol. Technol. Agric. 8, 18. https://doi.org/10.1186/s40538-021-00217-8.)
OVERALL: Please, re-arrange all equations and table in order to fit the template of the Journal.
FIGURE 5: Please, re-arrange Fgiure 5 by separating the text from the images. The reader must be clearily guided and supported in the understanding of all sections.
Author Response
Please see the attachment.
Author Response File: Author Response.pdf
Reviewer 3 Report
The author have a great improvement for thier study, I agree to publish this paper on Remote Sening
Author Response
Please see the attachment.
Author Response File: Author Response.pdf