Improvement of Typhoon Intensity Forecasting by Using a Novel Spatio-Temporal Deep Learning Model
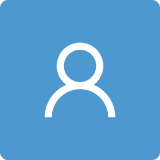
Round 1
Reviewer 1 Report
Overview:
The manuscript structure was well written and easy to read. This study not only compared the results of other deep learning models, but also compared the results of numerical models such as HWRF and forecasting agencies such as CMA, JMA, and JTWC showed superiority in predicting typhoon intensity.
L42-43:
Why did you define the typhoon intensity as the 2-minute average wind speed? JTWC reports the maximum sustained surface winds in tropical disturbances and cyclones in terms of 1-minute mean wind speed. Other nations, however, report maximum sustained surface winds averaged over a different time interval, which in many cases is 10-minutes. RSMC-Tokyo uses 10-minutes mean wind speed.
L102-104:
Why did you use the ECMWF re-analysis data? You could not get re-analysis data from CMA?
L260-261, L285, L329:
Write the reference. ‘Error! Reference 260 source not found.’ is found.
L432:
NET.. --> NET.
Author Response
Please see the attachment.
Author Response File: Author Response.pdf
Reviewer 2 Report
In this paper, the authors propose a novel TC intensity forecasting model based upon deep learning. In particular, the deep learning model uses multi-dimensional environmental variables and attempts to capture the spatio-temporal dependencies of these variables. The preliminary experiments with this model show measurable improvement (as measured by mean absolute error) compared with existing dynamical models from official agencies (such as the National Hurricane Center).
Although the results are in preliminary form and that improvements should be made (especially with short-term forecasts), I believe that the results are significant and worthy of publication. I have attached my full commentary in the document below. I have two overall comments to add:
(1) Throughout the paper, there were errors associated with in-text citations (numerous times it is said that "Error! Reference source not found"). These should be corrected before the paper is submitted to publication.
(2) Much of the data that is used for the deep learning model are associated with the external factors that drive TC intensity variability. However, much modern research indicates that the variability in TC intensity is also governed by inner core processes (such as eyewall replacement cycles, mesovortices, etc.). It would be useful if the authors could address how internal TC dynamics could be addressed with the deep learning model. Perhaps that is one reason why the deep learning model under-performs for short-range forecasting.
Comments for author File: Comments.pdf
Author Response
Please see the attachment.
Author Response File: Author Response.pdf
Reviewer 3 Report
Interesting efforts to certainly be continued, possibly using other available data sets (e.g. NCEP, Japanese Reanalysis). Numerous previous studies have shown that the wind speeds obtained near the typhoon center from the reanalysis data cannot accurately reproduce the observed wind fields. Thus, modifications are generally needed (e.g. blending method with parametric models). In the introduction of the present paper, some comments are thus recommended. The fact that both the intensity and exact size of the maximal winds are not really well resolved by present-day reanalysis, i would invite the authors to provide some perspectives and future investigations to improve the present efforts.
Minor comment: I would recommend to use spatio-temporal rather than tempo-spatial
Author Response
Please see the attachment.
Author Response File: Author Response.pdf