YOLO-DCTI: Small Object Detection in Remote Sensing Base on Contextual Transformer Enhancement
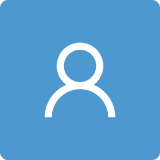
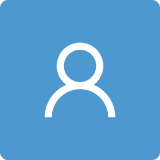
Round 1
Reviewer 1 Report
Issue 1:
The authors state that they integrate the CoT-I module into a decoupled detection head named DCTI, enabling the establishment of global interdependencies between the classification and regression tasks through self-attention mechanisms. However, in Figure 1, the overall framework, the structure of DCTI is not clearly illustrated. To facilitate a better understanding, the authors should provide a detailed explanation of the components comprising DCTI.
Issue 2:
In Figure 3, the authors refer to "Features," which I believe correspond to the three sets of features obtained after the PAN module. However, the manuscript does not explicitly mention whether these features have different dimensions in terms of width and height. To address this, the authors should provide a specific explanation and clarification regarding the dimensions of these features.
Author Response
Manuscript ID: remotesensing-2532161.R1
Paper Title: Yolo-DCTI: Small Object Detection in Remote Sensing Base on Contextual Transformer Enhancement
Authors: Lingtong Min, Ziman Fan, Qingyi Lv*, Mohamed Reda, Linghao Shen and Binglu Wang
Response to Reviewers’ Comments
The authors are grateful to the reviewers for their constructive comments, which have contributed in improving the quality of the paper. In the following, we provide detailed, item-by- item, point-by-point responses to all the very interesting issues raised by the Anonymous Reviewers.
[Reviewer 1]
Comment 1: The authors state that they integrate the CoT-I module into a decoupled detection head named DCTI, enabling the establishment of global interdependencies between the classification and regression tasks through self-attention mechanisms. However, in Figure 1, the overall framework, the structure of DCTI is not clearly illustrated. To facilitate a better understanding, the authors should provide a detailed explanation of the components comprising DCTI.
Response:
We sincerely value your review and the invaluable suggestions provided. Your recommendations are greatly appreciated, and we've taken steps to address and refine the unclear descriptions within the paper. Specifically addressing the challenge of explaining the DCTI structure in Problem 1, we've implemented the following enhancement: To offer a more lucid depiction of the DCTI structure, we've included its components in the caption of Figure 1. A comprehensive elaboration on the DCTI will be presented in Section 3.3, whereas at this juncture, we provide a concise overview of its overarching composition.
Comment 2: In Figure 3, the authors refer to "Features," which I believe correspond to the three sets of features obtained after the PAN module. However, the manuscript does not explicitly mention whether these features have different dimensions in terms of width and height. To address this, the authors should provide a specific explanation and clarification regarding the dimensions of these features.
Response:
To begin with, we've incorporated precise details about the origins of the features, acquired subsequent to the backbone, FPN, and PAN stages. Moreover, we've furnished clarifications concerning the three distinct dimensions of these features. In Figure 3, we've opted to exclusively exhibit variations within the channel dimension (C) to enhance visual clarity. Your valuable suggestion has significantly enriched our paper, and we extend our sincere appreciation.
Author Response File: Author Response.pdf
Reviewer 2 Report
This paper proposed a small object detection method using contextual transformer enhancement. The method has the merits and the experiments demonstrate the effectiveness. To improve the manuscript, the following two questions can be considered in the minor revision.
1. In Fig. 2, the architectures of CoI can be beautified to remove the cross lines.
2. In the experiment, more visualizations of intermediate features (such as Fig 9) can be given.
Author Response
Manuscript ID: remotesensing-2532161.R1
Paper Title: Yolo-DCTI: Small Object Detection in Remote Sensing Base on Contextual Transformer Enhancement
Authors: Lingtong Min, Ziman Fan, Qingyi Lv*, Mohamed Reda, Linghao Shen and Binglu Wang
Response to Reviewers’ Comments
The authors are grateful to the reviewers for their constructive comments, which have contributed in improving the quality of the paper. In the following, we provide detailed, item-by- item, point-by-point responses to all the very interesting issues raised by the Anonymous Reviewers.
[Reviewer 2]
Thank you very much for reviewing our paper and providing valuable feedback. We sincerely appreciate your suggestions and have supplemented and modified the issues related to visualization figures in the paper.
Comment 1: In Fig. 2, the architectures of CoI can be beautified to remove the cross lines.
Response: We have reworked the structural diagram of COT-I, eliminating any intersecting lines.
Comment 2: In the experiment, more visualizations of intermediate features (such as Fig 9) can be given.
Response: We've integrated Grad-CAM maps into the NWPU VHR-10 dataset. Your valuable input is greatly appreciated and has enhanced the depth of our study.
Author Response File: Author Response.pdf
Reviewer 3 Report
This paper presents an innovative approach to Small Object Detection in Remote Sensing images by leveraging a combination of YOLOv7 and a Visual Transformer. The paper demonstrates commendable writing and organization. However, certain concerns exist regarding the proposed method and concepts that warrant clarification:
1- The author denotes that mAP is computed at a Single IoU threshold of 0.5, suggesting that this metric should exceed 0.5 for [email protected] and also for [email protected]:0.95. To ensure a more comprehensive evaluation, mAP should be evaluated at continuous IoU thresholds, such as [email protected] for low precision allocation [0.5: 1] and [email protected] for high precision allocation [0.75: 1]. Consequently, all results should be recalculated based on these more inclusive metrics.
2- In Figure 7, the labels for sub-images A and B are not appropriately placed, creating ambiguity in the visual representation.
3- In Figure 8, the 5th sub-image indicates that most small vehicles, which qualify as small objects, are not recognized. This issue merits attention and requires further investigation.
3- The paper lacks an essential comparison between the proposed method and other existing methods in terms of Inference speed and parameters. Providing such a comparison is crucial to understanding the relative strengths and weaknesses of the proposed approach.
4- Visual examples for the VisDrone and NWPU VHR-10 Datasets are notably absent. Including these examples would enhance the clarity and applicability of the proposed method.
No Comments
Author Response
Manuscript ID: remotesensing-2532161.R1
Paper Title: Yolo-DCTI: Small Object Detection in Remote Sensing Base on Contextual Transformer Enhancement
Authors: Lingtong Min, Ziman Fan, Qingyi Lv*, Mohamed Reda, Linghao Shen and Binglu Wang
Response to Reviewers’ Comments
The authors are grateful to the reviewers for their constructive comments, which have contributed in improving the quality of the paper. In the following, we provide detailed, item-by- item, point-by-point responses to all the very interesting issues raised by the Anonymous Reviewers.
[Reviewer 3]
This paper presents an innovative approach to Small Object Detection in Remote Sensing images by leveraging a combination of YOLOv7 and a Visual Transformer. The paper demonstrates commendable writing and organization. However, certain concerns exist regarding the proposed method and concepts that warrant clarification:
Comment 1: The author denotes that mAP is computed at a Single IoU threshold of 0.5, suggesting that this metric should exceed 0.5 for [email protected] and also for [email protected]:0.95. To ensure a more comprehensive evaluation, mAP should be evaluated at continuous IoU thresholds, such as [email protected] for low precision allocation [0.5: 1] and [email protected] for high precision allocation [0.75: 1]. Consequently, all results should be recalculated based on these more inclusive metrics.
Response:
Thank you for your review and valuable feedback. We appreciate your suggestions and have clarified and modified the description of the evaluation metrics in the paper.
Specifically, in the paper, "[email protected]" denotes the mean Average Precision when the Intersection over Union (IoU) threshold exceeds 0.5, and "[email protected]:0.95" is calculated across IoU thresholds ranging from 0.5 to 0.95. We apologize for any inaccuracies in the initial description and have now rectified it accordingly..
In this paper, we adopt the AP at IoU=0.5 according to the PASCAL VOC standard and AP at IoU=.50:05:95 according to the COCO2014 standard as the evaluation metrics for object detection. We believe that these two metrics have broad applicability and effectively capture the performance of object detection algorithms. As shown in Figure 1, we use [email protected]:0.95 and [email protected] to represent the two metrics, respectively. Additionally, Wan et al. also employ the same evaluation metrics in object detection [1-4].
[1]Wan D, Lu R, Wang S, et al. YOLO-HR: Improved YOLOv5 for Object Detection in High-Resolution Optical Remote Sensing Images[J]. Remote Sensing, 2023, 15(3): 614.
[2]Liu Z, Gao Y, Du Q, et al. YOLO-extract: improved YOLOv5 for aircraft object detection in remote sensing images[J]. IEEE Access, 2023, 11: 1742-1751.
[3]Zakria Z, Deng J, Kumar R, et al. Multiscale and direction target detecting in remote sensing images via modified YOLO-v4[J]. IEEE Journal of Selected Topics in Applied Earth Observations and Remote Sensing, 2022, 15: 1039-1048.
[4]Cheng G, Yuan X, Yao X, et al. Towards large-scale small object detection: Survey and benchmarks[J]. IEEE Transactions on Pattern Analysis and Machine Intelligence, 2023.
We have refined the description of the evaluation metrics to ensure clarity and enhance readers' understanding of the metrics employed in our study. Your astute observation is greatly valued, as it has contributed to the overall enhancement of our research.
Figure 1. COCO Official Evaluation Metrics (https://cocodataset.org/#detection-eval)
Comment 2: In Figure 7, the labels for sub-images A and B are not appropriately placed, creating ambiguity in the visual representation.
Response:
We sincerely appreciate your vigilance in bringing this matter to our attention. We had likewise noted this inconsistency. To rectify the situation, we have included the model's name on the leftmost section of Figure 7, thereby enhancing the clarity of the comparative results.
Comment 3: In Figure 8, the 5th sub-image indicates that most small vehicles, which qualify as small objects, are not recognized. This issue merits attention and requires further investigation.
Response:
Thank you for pointing out this issue. We believe that the missed detections may be due to the complexity involved in recognizing similar attributes in highly congested environments. We have added supplementary explanations in the paper regarding the missed detections in Figure 8, and we have also provided an analysis in the conclusion.
Comment 4: The paper lacks an essential comparison between the proposed method and other existing methods in terms of Inference speed and parameters. Providing such a comparison is crucial to understanding the relative strengths and weaknesses of the proposed approach.
Response:
Firstly, we've directly compared the proposed method with other existing approaches in terms of inference speed and parameters. Secondly, we've introduced a new subsection to offer explanations and analyses related to this aspect.
Comment 5: Visual examples for the VisDrone and NWPU VHR-10 Datasets are notably absent. Including these examples would enhance the clarity and applicability of the proposed method.
Response:
We've incorporated visual examples from the VisDrone and NWPU VHR-10 datasets to bolster persuasiveness and credibility.
Author Response File: Author Response.pdf
Reviewer 4 Report
Dear authors,
thank you for your submission. The paper seems well structured and relevant. I don't have any remarks for improvement.
Author Response
Manuscript ID: remotesensing-2532161.R1
Paper Title: Yolo-DCTI: Small Object Detection in Remote Sensing Base on Contextual Transformer Enhancement
Authors: Lingtong Min, Ziman Fan, Qingyi Lv*, Mohamed Reda, Linghao Shen and Binglu Wang
Response to Reviewers’ Comments
The authors are grateful to the reviewers for their constructive comments, which have contributed in improving the quality of the paper. In the following, we provide detailed, item-by- item, point-by-point responses to all the very interesting issues raised by the Anonymous Reviewers.
[Reviewer 4]
Thank you for your submission. The paper seems well structured and relevant. I don't have any remarks for improvement.
Response:
We extend our gratitude for your review of our paper and the positive feedback provided!
Author Response File: Author Response.pdf
Round 2
Reviewer 3 Report
Thanks for your thoughtful and considerate responses to the comments.