Mitigating Masked Pixels in a Climate-Critical Ocean Dataset
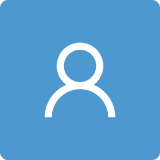
Round 1
Reviewer 1 Report
Comments and Suggestions for AuthorsThis paper presents an NLP-inspired algorithm trained with high-fidelity ocean simulations to accurately restore masked or missing data in SST fields—an essential climate variable identified by the GCOS. The study highlights that the Enki model consistently surpasses previously employed inpainting techniques, achieving significantly lower reconstruction errors by up to an order of magnitude, even in scenarios where a majority of pixels are masked.
In terms of enhancement, the paper could benefit from:
1. Providing a clearer explanation of the research motivation and challenges addressed in the abstract, rather than diving straight into the work description. The authors should articulate the significance of studying masked pixel mitigation.
2. Conducting a more thorough literature review, as the introduction currently includes only nine references.
3. Expanding the experimental design in Section 3 to include additional metrics beyond RMSE and exploring a broader range of comparisons, such as linear and cubic aspects, to better validate the efficacy of the proposed algorithm.
4. Improving the clarity of images in the paper and enhancing the font size, particularly in figures like Figure 1.
5. Including an overarching algorithm framework in Section 2 to showcase the machine learning algorithms employed in developing the model discussed in this paper.
Author Response
Please see the PDF which responds to all 4 Reviewers
Author Response File: Author Response.pdf
Reviewer 2 Report
Comments and Suggestions for AuthorsAfter reviewing the manuscript entitled "Mitigating masked pixels in a climate-critical ocean dataset" I would recommend it for minor revision. I have following very basic comments:
1. Page 1. L20-24: Please provide some references for the statement in line 20-24 (In 1978, .....surface height and SST)
2. Page 2, L39-40: Please provide reference for the statement "On average .......... estimate of SST".
3. Page 2, L42: Remove "several"
4. page 3, L82: "variables"
5. Page 3, L106: Does the statement "percentage of pixels masked during training" means e.g. for t = 75% only 25% data will be used for training the model.
6. Page 4, L133-135: "In this case ............ (m% = 30)"
How do you keep the trained dataset insulated from the test data, to keep the purity of the test data. e.g. in fig 2, the training was done on a 20% masked image and then implemented on a 30% masked image, however, it seems you used the same data with different masks for both training and testing.
7. Page 6, L208: Does "most complex cutout" means that LL is < 200? Can you elaborate on the threshold of LL values.
8. Page 6, L222: Remove "compared"
Author Response
Please see the attached PDF which responds to all 4 Reviewers.
Author Response File: Author Response.pdf
Reviewer 3 Report
Comments and Suggestions for AuthorsThis manuscript presents an innovative approach, ENKI model, that combines natural language processing techniques with high-fidelity ocean simulations to reconstruct masked or missing data in sea surface temperature fields. The authors show the performance of ENKI under different pixels masked and training percent, and then demonstrate the superiority of their proposed ENKl model over previously adopted inpainting techniques, achieving significantly lower reconstruction errors. The manuscript is not only well-written but also incredibly easy to follow, making it accessible for a broad audience. Overall, this is a high-quality study that makes a valuable contribution to the field of data reconstruction. I am impressed with this study and recommend it for publication subject to minor revisions.
Several minor issues exist in this article.
1. Figure 5 illustrates ENKI's reconstruction performance on the validation dataset. It is recommended to provide a certain error bar, especially for Figure 5b, so as to give readers a more comprehensive understanding of the reliability of the methodology's results.
2. In Figure 8, the results indicate that under low LLulmo conditions, ENKI's RMSE is higher than that of the biharmonic inpainting algorithm. Could you provide an explanation for this? Please consider the impact of this finding on the method's practical applications.
Author Response
Please see the attached PDF which responds to all 4 Reviewers.
Author Response File: Author Response.pdf
Reviewer 4 Report
Comments and Suggestions for AuthorsThis manuscript describes the ability of a Natural Language Processing algorithm dubbed ENKI, to fill missing pixels in remotely sensed sea surface temperature (pixels missing usually because of clouds oro ther atmospheric effects).
The algorithm is trained on numerical ocean model output and most of the test are done on such output, but a test involving real SST data from the VIIRS instrument is also included.
The manuscript thoroughly describes various tests of the algorthm's ability to reproduce withheld model data, and against the output of various other algorithms, concluding that ENKI yields the lwoest RMS discrepancies from the model data.
The test against VIIRS actual data yields higher values of discrepancy, but compatible with the known noise level of the instrument. The authors speculate that instrument noise and model resolution are the culprits and promise a future study to evaluate these hypotheses.
The only minor weakness is the lack of description of the algorithm itself (there is a description of the I/O to the algorithm), although a reference to Goh's similar work. Presumably the NLP algorithm uses a combination of neural networks and text structure algorithms.
Author Response
Please see the attached PDF which responds to all 4 Reviewers.
Author Response File: Author Response.pdf
Round 2
Reviewer 1 Report
Comments and Suggestions for AuthorsPrevious issues have been addressed.