Data Filling of Micrometeorological Variables in Complex Terrain for High-Resolution Nowcasting
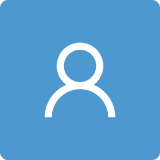
Round 1
Reviewer 1 Report
Dear Author(s),
Thanks for the research that you have done. Below you will see my comments to improve the manuscript.
The current manuscript presents an application of two Multivariable linear regression (MLR) and artificial neural networks (ANN) algorithms for filling the gaps or nowcasting of some meteorological variables including wind speed and direction, temperature, humidity, etc. from some weather stations in an almost small region with a complex terrain having thermally-driven and synoptically-forced flows. The data around one year (from January through March 2017 from LEMS station) was used. The focus was on the spatial scale rather than the time scale and they found that ANN and MLR provide almost the same result and considering the computation time they suggested MRL.
The paper is generally well written, terminology mixed with the literature were well explained, the methods were clearly described. While the nowcasting or gap-filling is of great interest to the wide community in different applications, I haven’t fully convinced about the results. The test of significance needs to be done when two methods are compared. Therefore, I recommend major revision or reject and resubmit to warrant enough time for additional work.
- Line 67 to 87: The principle of ANN was described in detail which is good but it has been described in detail in many papers. Thus, the author can summarize this part and refer to relevant papers.
- In line 157, the authors said that one of the novelties of this research is the comparisons between the two methods. I am a little disagree with that and it cannot be considered a novelty.
- How much the methods are sensitives to the time interval? In this manuscript, the author used 5-minute average of the data.
- In lines 235 and 236 author says that while many use stochastic gradient descent they used the Levenberg-Marquardt algorithm because it is the fastest method. Is it just because of the speed? What about the accuracy?
- Line 245: In the testing phase the split method (30 % and 70 % for testing and training respectively) have been used. Additionally, the duration of the test data is around 10 days in January and February 2017. Thus, the presented result cannot be more accurate. The author can think of using more accurate testing methods like cross-validation instead of splitting the data into 30 and 70 percent for test and train.
- Line 252: “This ensemble model typically has better performance than a single model, and is less likely to show outlier performance.” Reference?
- In line 277 to 283 authors says that they used LEMS I, J, and K for training because of some reasons (representative of different flows) as they mentioned in the manuscript. What will the results be like if they use different combinations of other stations?
- In Line 283: “LEMS C was not used in any of the tests because a complete dataset for it was not available.” I think no need to be presented even in the text, in figure 2, and in table 1. It would be better just to mention the station (s) that have been used.
- In different parts of the manuscript, the author (s) focused more on the computational time when they compared the two methods. How long does an ANN algorithm takes time? Is it the only advantage of MLR on ANN? Is it a serious problem in the gap filling?
- The test of significance needs to be done when two methods are compared. Look at some works like “Valavi, R., Guillera‐Arroita, G., Lahoz‐Monfort, J.J. and Elith, J., 2021. Predictive performance of presence‐only species distribution models: a benchmark study with reproducible code. Ecological Monographs, p.e1486.” and “Shafizadeh-Moghadam, H., Weng, Q., Liu, H. and Valavi, R., 2020. Modeling the spatial variation of urban land surface temperature in relation to environmental and anthropogenic factors: a case study of Tehran, Iran. GIScience & Remote Sensing, 57(4), pp.483-496. ”
- The study region is 6 km by 1 km which is not a large region regarding the change in temperature, humidity, temperature, etc in meteorology. Do thermally-driven flows and synoptically-forced flows various significantly in that small region?
- In figures 3 and 4 Y-axis in the different plots are not equal.
Author Response
Please see the attachment.
Author Response File: Author Response.pdf
Reviewer 2 Report
The paper titled “Data Filling of Micrometeorological Variables in Complex Terrain for High-Resolution Nowcasting” compares the results of the Linear Regression and Artificial Neural Network for the nowcasting of the horizontal wind components, potential temperature, and specific humidity. The paper is well-written, and the methods are described very carefully. Also, the paper fits the scope of the journal. However, there are a few concerns that should be addressed before the work is accepted for publication. Therefore, I recommend a major revision.
- ANN or Fully Connected Deep Neural Networks are known to have issues with tabular data. Therefore, it is not surprising to me that the LR is able to have a better performance. I recommend adding another benchmark for comparison which is the Random Forest algorithm. Random Forest is widely used in the field for different purposes. You can refer to this paper for a detailed explanation of the method: https://doi.org/10.1175/JHM-D-20-0240.1. Also, I believe that the algorithm is available in MATALB.
- The abstract is qualitative. Please add some quantitative statements to the abstract. For example, you can compare the percent improvement gained by using LR vs ANN.
- Line 84: This is not necessarily true. Loss functions can be MSE, MAE, or any other customized function.
- Line 86: This is not correct. Testing is evaluating the permanence of the model over instances/data that is not used during the training phase. Please revise the manuscript accordingly.
- Line 88: linearly model a single …
- Line 88: We can have multiple targets in linear regression. Please revise the text accordingly.
- Line 98-104: One of the very novel applications of nowcasting in atmospheric sciences is precipitation nowcasting. Please cite state-of-the-art literature accordingly. For example arXiv:2108.06868
- Line 125: Please cite more references here as it is a very common topic in the field. For example, https://doi.org/10.3390/w12113067 uses machine learning for the gap filling of several time series.
- Fig 1: Please add lat and lon ticks to the map
- Fig 2: Add latitude and longitude ticks to the map. Add legend for the land use map.
Author Response
Please see the attachment.
Author Response File: Author Response.pdf
Reviewer 3 Report
The authors used artificial neural networks (ANNs) and multiple linear regression (MLR) to nowcast meteorological variables, and found that MLR performed as well as ANNs. The paper is in publishable form.
Minor comments
- artificial-neural-network -> artificial neural network
- multiple-linear-regression -> multiple linear regression
- in line 669, kalman -> Kalman
- in line 678, gaussian -> Gaussian
Author Response
Please see the attachment.
Author Response File: Author Response.pdf
Round 2
Reviewer 1 Report
The authors have properly answered my questions. The updated manuscript has been clearly improved. I would recommend that this manuscript is ready to publish. Thanks.
Author Response
Please see the attachment.
Author Response File: Author Response.pdf
Reviewer 2 Report
As I explained clearly in the previous round of the review, fully connected deep neural networks have issues dealing with tabular data, specifically due to the non-stationarity of the climatic forcings/variables. Comparing ANN results with that of LR does not provide any insight regarding how the performance of the ANN is and we need another benchmark for comparison. Therefore, the experiment design of the study is flawed. I suggested adding random forest as another benchmark which the authors did not consider. From my perspective, the work still needs a major revision. However, I leave the decision on the manuscript to the dear editor.
Author Response
Please see the attachment.
Author Response File: Author Response.pdf