Fragility Curves for Material Characteristics and Damage Index for Brick Masonry Exposed to Freeze–Thaw Action
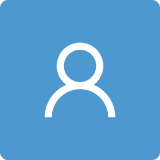
Round 1
Reviewer 1 Report
The content of the manuscript is not to the otherness of the journal. In the first part, freeze-thaw curves are investigated by regression. While in the second part it is applied on a hypothetical case study. The research setup is completely wrong
Author Response
Response to Reviewer 1
Author's Notes to Reviewer:
The authors wish to thank the reviewer for his/her valuable comments. Wherever it was possible, we considered and included his/her guidance regarding article’s shortcomings (research design, description of methods, presentation of results). Changes to the manuscript can be tracked in attached paper where “Track Changes” function was used.
Point by point response:
Point 1: The content of the manuscript is not to the otherness of the journal. In the first part, freeze-thaw curves are investigated by regression. While in the second part it is applied on a hypothetical case study. The research setup is completely wrong.
Response 1:
The reviewer has expressed his/her general opinion about the research presented and has not explicitly pointed out what bothers him/her. The statement "The research setup is completely wrong." does not help the authors to improve the article. It is worth noting that none of the other reviewers disputed the research setup. More so, they agreed with the structure of the article and presented results. Those who were not satisfied with parts of the article and/or those who found some shortcomings made explicit suggestions to the authors on how to improve the article.
It should be noted that the study of the freeze-thaw phenomenon was not limited to "freeze-thaw curves are investigated by regression". Subsection 2.2 describes a very complex approach that is quite difficult to understand for the reader who is not familiar with artificial intelligence methods and probabilistic analysis. Note that the aim of the article was to first introduce regression equations for two types of wallets and, in the last part, to construct advanced fragility curves which enable probabilistic risk assessments in case of deterioration of ductility and increase of damage on brick masonry due to freeze-thaw cycles. The latter part is one of the main novelties of the present study and, to the author's knowledge, has not been applied before to the phenomenon of freeze-thaw in brick masonry.
The purpose of the hypothetical case, which is only a part of the Discussion section, was to demonstrate one of the possible applications of fragility curves in everyday engineering practice. (It should be noted that fragility curves have been a very useful tool in the field of earthquake engineering for years.) Please note that fragility curves are the tool that allows a relatively easy transition from the classical deterministic approach (which is no longer sufficient for a complete understanding of natural/engineering phenomena) to the more accurate probabilistic approach.
Nevertheless, authors tried to do their best to improve the paper. The following changes (they are many!) have been applied:
- The presentation of the methods in paragraph 2.2. has been considerably amended and expanded. Consequently, some of the old text has been amended and deleted and new text added for clarification. It should be noted that this paragraph describes a very complex approach and is quite difficult to understand for the reader who is not familiar with artificial intelligence methods and probabilistic analysis. Note that the aim of the paper was to first introduce regression equations for two types of wallets and, in the last part, to construct advanced fragility curves which enable probabilistic risk assessments in case of ductility deterioration and increase of damage on brick masonry due to freeze-thaw cycles. The latter part is one of the main novelties of the presented study.
The whole of subsection 2.2. now reads as follows:
2.2. Analytical part
Fragility curves, which enable probabilistic risk assessments in case of ductility deterioration and increase of damage on brick masonry due to freeze-thawing cycles, are a novelty in this research. They are presented for the first time on the basis of a very complex mathematical model based on the combination of two research areas (artificial intelligence methods and probability analysis). The presentation of the whole demanding process of determining the fragility curves is not of primary importance in this article and cannot be presented in a short and at the same time simple (easy to understand) form. Therefore, the interested reader should find additional and supplementary information in the cited literature.
In this subsection, we briefly present a general mathematical framework of the proposed models in the form of formal (general) equations, which requires (partial) inference to artificial intelligence methods (neural networks, fuzzy logic) and probabilistic analysis, including sampling procedures. Note that the proposed framework is presented in such a way that it can be applied to regression analyses (which determine the general trends of the observed phenomena) and fragility curves (which enable probabilistic risk assessments of the observed phenomenon). Due to the complexity of the whole approach, only a very brief description is given here.
A statistical/probabilistic model, which is typically derived from the observed data and is presented in this paper, is a special class of mathematical models. Since it is non-deterministic, some or all of its variables do not have specific values, but instead have probability distributions (i.e. some or all of the variables are of random nature). It should be noted, that basically all of the phenomena in structural engineering are of random nature, but due to the complexity, they are often treated as deterministic systems. The behavior of masonry wallets during the F/T cycles (in the presented research expressed in terms of the modulus of elasticity, ductility and damage) can be determined by observing/measuring N wallets during the experimental testing. The mathematical description of the observation of one wallet during the testing, which simulates the F/T phenomenon, is called a model vector. As a result, the whole phenomenon can be described by a finite set of model vectors [21]:
(1)
It is assumed that the observation of one particular wallet can be described by a number of variables, which are treated as components of a model vector:
(2)
The vector can be further composed of two truncated vectors Qn and Rn:
and (3a)
is complementary to vector and therefore their concatenation yields the complete data model, vector . The prediction vector, too, is composed of two truncated vectors, i.e., the given truncated vector and the unknown complementary vector:
and (3b)
The problem now is how an unknown complementary vector can be estimated from a given truncated vector and the model vectors , i.e., how, e.g. the ductility of wallet, , can be estimated from known input parameters (type of mortar, number of F/T cycles, Young’s modulus etc.) and the available data collected in the database. In case of large amount of data (big databases) different methods can be applied. One of them is demonstrated in [22]. However, in case of small database, which is available for F/T phenomenon discussed in this paper, different approach should be applied. In order to obtained typical trends with F/T cycles, for optimal estimation of in terms of one or two mechanical properties, the standard statistical polynomial fit will be used in this (first) step. Two output parameters (M=2), Young’s modulus, E, and ductility, m, will be separately estimated as a function of two input parameters, F/T cycles (D=2) any type of mortar typeM. In this particular case equations 3 can be written as
and (4a)
and
and (4b)
In the presented research the estimates (i.e., functional relationship) of the variables and will be determined by using standard nonlinear regression (see Section 3).
However, to obtain as much information as possible from the observed phenomenon, a combination of Latin Hypercube Sampling (LHS) and fuzzy logic approach will be used next. While Latin Hypercube Sampling [23,24] is applied to obtain a reliable estimate of the statistical parameters for the probabilistic model, the fuzzy logic approach [25] is used to exploit the expert knowledge about the observed phenomenon (i.e. the influence of two mortars on the investigated mechanical properties). The latter approach is able to deal with the concept of partial truth, which enables incorporation of soft logic and/or engineering knowledge into the mathematical models. In summary, in terms of fragility curves for ductility and damage index will be estimated in this (second) step based on LHS data generated from assumed probability density functions and their statistical parameters (mean, standard deviation and coefficient of variation) of known input parameters of the phenomenon, including fuzzy logic. Note that in this case the formal description of equations 4 remains the same, only the finite set of model vectors (Nfc) increases significantly in this case due to the LHS (N Nfc).
Optimal estimation of will be carried out by applying the Conditional Average Estimation (CAE) method (Peruš et al., 2006). Note that the proposed approach (which includes application of LHS and fuzzy logic), takes into account the randomness and different uncertainties of the observed phenomenon of freezing and thawing. The fragility curve represents the probability curves of the F/T phenomenon. More specifically, the fragility curve of F/T phenomenon is defined as the conditional probability of exceedance for given values of the selected demand parameter (i.e., m) using the general definition [26,27]. The fragility curves can, therefore, be obtained by a series of reliability analyses (i.e., calculation of cumulative probability density functions for the mechanical property under consideration, taking into account all uncertainties) at varying demand thresholds. By ignoring the uncertainty in the demand [28,26], each fragility curve displays the probability that the actual mechanical property is less than or equal to a particular demand for this property.
- The following text was included in the description of Figure 1:
From Fig. 1 it can be seen that the black line representing EN requirement and the green line which represents the average achieved temperature are not aligned. The differences can be attributed to deficiencies of insulation of the climate chamber but also to the cooling system, unable to provide complete illustration of programmed cooling-heating scheme. The average temperature was obtained from eight thermocouples at different positions in the climate chamber. The proximity of some thermocouples to the door opening or to the cooling-heating source thus affected the average measured temperature resulting in peaks in green curve.
- R-squared value (R2) were calculated for models in Figure 2. Additional text was added at old line 254:
Note that a low R-squared value (R2) for wallets M1 generally indicates that the number of F/T cycles does not explaining much to the variation of Young's modulus and thus may indicate low predictive acceptance of the proposed model. However, it is well known that even with low R2 values, data with high variability can show a (significant) trend. As described above, the increase of Young's modulus at the beginning and the subsequent decrease has a sound physical basis. On the other hand, R2 value for wallets M3a indicates moderate predictive acceptance for Young’s modulus.
- The Figure 2 is improved. Additional labels were added in order to distinct between various models.
- The Figure 3 is improved. Additional labels were added in order to distinct between various models.
- R-squared value (R2) were calculated for models in Figure 3. Additional text was added at old line 280:
Note that a high R2 value for wallets M1 and a low R2 value for wallets M3a indicate high and low predictive acceptance for ductility, respectively. As with the Young's modulus, note that both derived relationships have a sound physical basis and the quality of the predictive equations cannot be judged from the R2 values alone.
- The following text (in red) was included in the caption of Figure 4:
Figure 4. Fragility curves (assumption of normal probability distribution) for ductility for M1 (left) and M3a wallets (right). Note that bold lines correspond to theoretical cdfs, while thin lines corresponds to the CAE estimation of cdfs.
- We made the thin lines in Fig. 4 visible.
- The following text (in red) was included in the caption of Figure 6:
Figure 6. Normal and log-normal fragility curves for damage index for M1 (left) and M3a (right) wallets. Bold and thin lines correspond to fitted theoretical cdfs based on normal (bold) and log-normal (thin) probability distributions, respectively.
- We made the thin lines in Fig. 6 visible.
- The following text (in red) was included in the caption of Figure 8:
Figure 8. Predicted values for Young’s modulus (top) and ductility (bottom) after 20 years for existing masonry brick building in hypothetical scenario. Graphs from Figures 2 and 3 were applied.
- Some changes related to the variables and general descriptions in Figs. 4, 5, 6, and 9 were made as follows:
Variables, presented in Figs. 4 (Fragility and Ductility), 5 (Damage index and Number of F/T cycles), 6 (Fragility and Damage index) and 9 (Fragility and Damage index) are all without units. We noticed inconsistencies at variable’s abbreviations and corresponding designations of units in some figures – they are now fixed in Figs. 2-6 and 8-9.
- The following text (in red) was included in the Conclusion
The presented research deals with F/T phenomenon of brick wallets that simulate structural elements (walls) of masonry buildings exposed to floods followed by cold weather. In an attempt to predict the progress of degradation and estimate the corresponding damage, the mathematical models which are able to predict important mechanical parameters and damage for brick wallets at given number of F/T cycles have been developed. Proposed statistical regressions enable estimation of deterioration of Young’s modulus and displacement ductility. Although the trends discovered have sound physical meanings, the low R2 values for Young's modulus in the case of M1 wallets and for ductility in the case of M3a wallets suggest that the proposed regressions should be used with caution. At the first stage the damage did not have significant effect on Young’s modulus. After 50 F/T cycles (M3a) and 80 F/T cycles (M1) the values begin to decrease. Although the wallets M3a built with improved mortar achieve significantly higher Young’s modulus, it’s decrease is more pronounced compared to wallets M1 built with ordinary one. Regarding the ductility properties somewhat better behavior can be observed by wallets built with improved mortar. In addition, proposed fragility curves for ductility and damage index (which is important novelty of the presented study) enable probabilistic risk assessments in case of deterioration of ductility and increase of damage on brick masonry due to F/T cycles. In terms of damage index wallets built with improved mortar showed higher durability compared to ordinary ones which is a good indicator for development and usage of other types of modified mortars. A simple use case has also been presented demonstrating usefulness of proposed graphs. It was shown how one is able to predict and obtain the values of Young’s modulus as well as the ductility at the selected age of a building exposed to F/T cycles.
Author Response File: Author Response.docx
Reviewer 2 Report
-
Author Response
Response to Reviewer 2
Author's Notes to Reviewer:
The authors wish to thank the reviewer for his/her valuable comments.
We have considered your suggestion for a better description of the methods. The presentation of the methods in paragraph 2.2. has been considerably altered and enlarged. This means that some of the old text has been changed and deleted and new text has been added for clarification.
The whole of subsection 2.2. now reads as follows:
2.2. Analytical part
Fragility curves, which enable probabilistic risk assessments in case of ductility deterioration and increase of damage on brick masonry due to freeze-thawing cycles, are a novelty in this research. They are presented for the first time on the basis of a very complex mathematical model based on the combination of two research areas (artificial intelligence methods and probability analysis). The presentation of the whole demanding process of determining the fragility curves is not of primary importance in this article and cannot be presented in a short and at the same time simple (easy to understand) form. Therefore, the interested reader should find additional and supplementary information in the cited literature.
In this subsection, we briefly present a general mathematical framework of the proposed models in the form of formal (general) equations, which requires (partial) inference to artificial intelligence methods (neural networks, fuzzy logic) and probabilistic analysis, including sampling procedures. Note that the proposed framework is presented in such a way that it can be applied to regression analyses (which determine the general trends of the observed phenomena) and fragility curves (which enable probabilistic risk assessments of the observed phenomenon). Due to the complexity of the whole approach, only a very brief description is given here.
A statistical/probabilistic model, which is typically derived from the observed data and is presented in this paper, is a special class of mathematical models. Since it is non-deterministic, some or all of its variables do not have specific values, but instead have probability distributions (i.e. some or all of the variables are of random nature). It should be noted, that basically all of the phenomena in structural engineering are of random nature, but due to the complexity, they are often treated as deterministic systems. The behavior of masonry wallets during the F/T cycles (in the presented research expressed in terms of the modulus of elasticity, ductility and damage) can be determined by observing/measuring N wallets during the experimental testing. The mathematical description of the observation of one wallet during the testing, which simulates the F/T phenomenon, is called a model vector. As a result, the whole phenomenon can be described by a finite set of model vectors [21]:
(1)
It is assumed that the observation of one particular wallet can be described by a number of variables, which are treated as components of a model vector:
(2)
The vector can be further composed of two truncated vectors Qn and Rn:
and (3a)
is complementary to vector and therefore their concatenation yields the complete data model, vector . The prediction vector, too, is composed of two truncated vectors, i.e., the given truncated vector and the unknown complementary vector:
and (3b)
The problem now is how an unknown complementary vector can be estimated from a given truncated vector and the model vectors , i.e., how, e.g. the ductility of wallet, , can be estimated from known input parameters (type of mortar, number of F/T cycles, Young’s modulus etc.) and the available data collected in the database. In case of large amount of data (big databases) different methods can be applied. One of them is demonstrated in [22]. However, in case of small database, which is available for F/T phenomenon discussed in this paper, different approach should be applied. In order to obtained typical trends with F/T cycles, for optimal estimation of in terms of one or two mechanical properties, the standard statistical polynomial fit will be used in this (first) step. Two output parameters (M=2), Young’s modulus, E, and ductility, m, will be separately estimated as a function of two input parameters, F/T cycles (D=2) any type of mortar typeM. In this particular case equations 3 can be written as
and (4a)
and
and (4b)
In the presented research the estimates (i.e., functional relationship) of the variables and will be determined by using standard nonlinear regression (see Section 3).
However, to obtain as much information as possible from the observed phenomenon, a combination of Latin Hypercube Sampling (LHS) and fuzzy logic approach will be used next. While Latin Hypercube Sampling [23,24] is applied to obtain a reliable estimate of the statistical parameters for the probabilistic model, the fuzzy logic approach [25] is used to exploit the expert knowledge about the observed phenomenon (i.e. the influence of two mortars on the investigated mechanical properties). The latter approach is able to deal with the concept of partial truth, which enables incorporation of soft logic and/or engineering knowledge into the mathematical models. In summary, in terms of fragility curves for ductility and damage index will be estimated in this (second) step based on LHS data generated from assumed probability density functions and their statistical parameters (mean, standard deviation and coefficient of variation) of known input parameters of the phenomenon, including fuzzy logic. Note that in this case the formal description of equations 4 remains the same, only the finite set of model vectors (Nfc) increases significantly in this case due to the LHS (N Nfc).
Optimal estimation of will be carried out by applying the Conditional Average Estimation (CAE) method (Peruš et al., 2006). Note that the proposed approach (which includes application of LHS and fuzzy logic), takes into account the randomness and different uncertainties of the observed phenomenon of freezing and thawing. The fragility curve represents the probability curves of the F/T phenomenon. More specifically, the fragility curve of F/T phenomenon is defined as the conditional probability of exceedance for given values of the selected demand parameter (i.e., m) using the general definition [26,27]. The fragility curves can, therefore, be obtained by a series of reliability analyses (i.e., calculation of cumulative probability density functions for the mechanical property under consideration, taking into account all uncertainties) at varying demand thresholds. By ignoring the uncertainty in the demand [28,26], each fragility curve displays the probability that the actual mechanical property is less than or equal to a particular demand for this property.
Author Response File: Author Response.docx
Reviewer 3 Report
Remarks:
1. In Fig. 2, it is advisable to show the regression equations accompanied by the coefficients of determination R2. This will allow to assessing the degree of adequacy of regression models.
2. The regression coefficients in empirical models are too accurate compared to the data in Table 1 and Table 2.
3. It is necessary to present the indices to the corresponding Ew values, because it is not clear which empirical equation models the process.
4. In Fig. 3, it is necessary to present the indices m, because it is not clear which process models the regression equation.
5. The absence of determination coefficients does not allow to form an opinion about the degree of adequacy of the regression equation.
6. Figure 4 shows two options for changing the fragility. You need to give an identification of each process, for example “a”, “b” and give an explanation of which process is represented in each diagram.
7. The thin lines in Fig. 4 are not visible.
8. In Fig. 6, the diagrams should be shown as “a” and “b” and each should be explained.
9. The thin lines in Fig. 6 are almost invisible.
10. The empirical equations in Fig. 8 must be supplemented with determination coefficients.
11. The “modulus of elasticity” indicators have an accuracy of 0.5 on the diagram, and the regression equation has a coefficient of 1.428303. There is no engineering compliance.
12. The “ductility” indicators in the diagram of Fig. 8 have an accuracy of 1.0, but the regression equation has a coefficient of 5.09966.
13. In conclusion, it is necessary to present the range of validity of regression models.
General opinion: the manuscript may be of interest to the scientific community and recommended for publication, taking into account comments.
Author Response
Response to Reviewer 3
Author's Notes to Reviewer:
The authors wish to thank the reviewer for his/her valuable and very specific comments. In the following, we provide point by point response to the received comments.
Point by point response:
Point 1: In Fig. 2, it is advisable to show the regression equations accompanied by the coefficients of determination R2. This will allow to assessing the degree of adequacy of regression models.
Response 1: Fixed!
Additional text was added at old line 254:
Note that a low R-squared value (R2) for wallets M1 generally indicates that the number of F/T cycles does not explaining much to the variation of Young's modulus and thus may indicate low predictive acceptance of the proposed model. However, it is well known that even with low R2 values, data with high variability can show a (significant) trend. As described above, the increase of Young's modulus at the beginning and the subsequent decrease has a sound physical basis. On the other hand, R2 value for wallets M3a indicates moderate predictive acceptance for Young’s modulus.
Point 2: The regression coefficients in empirical models are too accurate compared to the data in Table 1 and Table 2.
Response 2: We are fully aware that, from an engineering point of view, due to all the unreliabilities of the phenomenon under consideration, a large number of decimal places is meaningless. In general, values in figures were calculated with more decimals due to smoother curves (and thus nicer images). The same applies to the final number of each parameter considered - we calculated it with great accuracy and finally rounded to the final value; as such is compared to the values in presented tables.
Nevertheless, according to the reviewer's comment, we reduced the number of decimal places in the constant term in the proposed regression equations.
Point 3: It is necessary to present the indices to the corresponding Ew values, because it is not clear which empirical equation models the process.
Response 3: The Figure 2 is improved. Additional labels were added in order to distinct between various models.
Point 4: In Fig. 3, it is necessary to present the indices m, because it is not clear which process models the regression equation.
Response 4: The Figure 3 is improved. Additional labels were added in order to distinct between various models.
Point 5: The absence of determination coefficients does not allow to form an opinion about the degree of adequacy of the regression equation.
Response 5: Fixed!
Additional text was added at old line 280:
Note that a high R2 value for wallets M1 and a low R2 value for wallets M3a indicate high and low predictive acceptance for ductility, respectively. As with the Young's modulus, note that both derived relationships have a sound physical basis and the quality of the predictive equations cannot be judged from the R2 values alone.
Point 6: Figure 4 shows two options for changing the fragility. You need to give an identification of each process, for example “a”, “b” and give an explanation of which process is represented in each diagram.
Response 6: The following text (in red) was included in the caption of Figure 4:
Figure 4. Fragility curves (assumption of normal probability distribution) for ductility for M1 (left) and M3a wallets (right). Note that bold lines correspond to theoretical cdfs, while thin lines corresponds to the CAE estimation of cdfs.
Point 7: The thin lines in Fig. 4 are not visible.
Response 7: Fixed!
Point 8: In Fig. 6, the diagrams should be shown as “a” and “b” and each should be explained.
Response 8: The following text (in red) was included in the caption of Figure 6:
Figure 6. Normal and log-normal fragility curves for damage index for M1 (left) and M3a (right) wallets. Bold and thin lines correspond to fitted theoretical cdfs based on normal (bold) and log-normal (thin) probability distributions, respectively.
Point 9: The thin lines in Fig. 6 are almost invisible.
Response 9: Fixed!
Point 10: The empirical equations in Fig. 8 must be supplemented with determination coefficients.
Response 10: Fixed!
The following text (in red) was included in the caption of Figure 8:
Figure 8. Predicted values for Young’s modulus (top) and ductility (bottom) after 20 years for existing masonry brick building in hypothetical scenario. Graphs from Figures 2 and 3 were applied.
Point 11: The “modulus of elasticity” indicators have an accuracy of 0.5 on the diagram, and the regression equation has a coefficient of 1.428303. There is no engineering compliance.
Response 11: We agree with the comment (see also Response #2). The value of the constant term was written as 1.43!
Point 12: The “ductility” indicators in the diagram of Fig. 8 have an accuracy of 1.0, but the regression equation has a coefficient of 5.09966.
Response 12: We agree with the comment (see also Response #2). The value of the constant term was written as 5.1!
Point 13: In conclusion, it is necessary to present the range of validity of regression models.
Response 13: The following text (in red) was included in the Conclusion
The presented research deals with F/T phenomenon of brick wallets that simulate structural elements (walls) of masonry buildings exposed to floods followed by cold weather. In an attempt to predict the progress of degradation and estimate the corresponding damage, the mathematical models which are able to predict important mechanical parameters and damage for brick wallets at given number of F/T cycles have been developed. Proposed statistical regressions enable estimation of deterioration of Young’s modulus and displacement ductility. Although the trends discovered have sound physical meanings, the low R2 values for Young's modulus in the case of M1 wallets and for ductility in the case of M3a wallets suggest that the proposed regressions should be used with caution. In addition, proposed fragility curves for ductility and damage index enable probabilistic risk assessments in case of deterioration of ductility and increase of damage on brick masonry due to F/T cycles. A simple use case has also been presented demonstrating usefulness of proposed graphs.
General opinion: the manuscript may be of interest to the scientific community and recommended for publication, taking into account comments.
Response To General opinion: The authors wish again to thank the reviewer for his/her very specific comments, which helped us to considerably improve the paper. We hope that all comments were considered correctly.
Author Response File: Author Response.docx
Reviewer 4 Report
The paper is interesting, appropriate designed and the results are clearly explained.
The one thing should be corrected, in our opinion.
In the paragraph 2.2 the beraf description of the test methods have to be given. In other case it is hard to understand the material.
Author Response
Response to Reviewer 4
Author's Notes to Reviewer:
The authors wish to thank the reviewer for his/her valuable comments and guidance. In the following, we provide point by point response to the received comments.
Point by point response:
Point 1: The paper is interesting, appropriate designed and the results are clearly explained.
Response 1: The authors thank the reviewer for his/her positive comment.
Point 2: The one thing should be corrected, in our opinion.
In the paragraph 2.2 the brief description of the test methods have to be given. In other case it is hard to understand the material?
Response 2: The presentation of the test methods in paragraph 2.2. has been considerably amended and expanded. Consequently, some of the old text has been amended and deleted and new text added for clarification. It should be noted that this paragraph describes a very complex approach and is quite difficult to understand for the reader who is not familiar with artificial intelligence methods and probabilistic analysis. Note that the aim of the paper was to first introduce regression equations for two types of wallets and, in the last part, to construct advanced fragility curves which enable probabilistic risk assessments in case of ductility deterioration and increase of damage on brick masonry due to freeze-thaw cycles. The latter part is one of the main novelties of the presented study.
Consequently, the new paragraph 2.2 now reads as follows:
2.2. Analytical part
Fragility curves, which enable probabilistic risk assessments in case of ductility deterioration and increase of damage on brick masonry due to freeze-thawing cycles, are a novelty in this research. They are presented for the first time on the basis of a very complex mathematical model based on the combination of two research areas (artificial intelligence methods and probability analysis). The presentation of the whole demanding process of determining the fragility curves is not of primary importance in this article and cannot be presented in a short and at the same time simple (easy to understand) form. Therefore, the interested reader should find additional and supplementary information in the cited literature.
Therefore, in this subsection, we briefly present a general mathematical framework of the proposed models in the form of formal (general) equations, which requires (partial) inference to artificial intelligence methods (neural networks, fuzzy logic) and probabilistic analysis, including sampling procedures. Note that the proposed framework is presented in such a way that it can be applied to regression analyses (which determine the general trends of the observed phenomena) and fragility curves (which enable probabilistic risk assessments of the observed phenomenon). Due to the complexity of the whole approach, only a very brief description is given here.
A statistical/probabilistic model, which is typically derived from the observed data and is presented in this paper, is a special class of mathematical models. Since it is non-deterministic, some or all of its variables do not have specific values, but instead have probability distributions (i.e. some or all of the variables are of random nature). It should be noted, that basically all of the phenomena in structural engineering are of random nature, but due to the complexity, they are often treated as deterministic systems. The behavior of masonry wallets during the F/T cycles (in the presented research expressed in terms of the modulus of elasticity, ductility and damage) can be determined by observing/measuring N wallets during the experimental testing. The mathematical description of the observation of one wallet during the testing, which simulates the F/T phenomenon, is called a model vector. As a result, the whole phenomenon can be described by a finite set of model vectors [21]:
(1)
It is assumed that the observation of one particular wallet can be described by a number of variables, which are treated as components of a model vector:
(2)
The vector can be further composed of two truncated vectors Qn and Rn:
and (3a)
is complementary to vector and therefore their concatenation yields the complete data model, vector . The prediction vector, too, is composed of two truncated vectors, i.e., the given truncated vector and the unknown complementary vector:
and (3b)
The problem now is how an unknown complementary vector can be estimated from a given truncated vector and the model vectors , i.e., how, e.g. the ductility of wallet, , can be estimated from known input parameters (type of mortar, number of F/T cycles, Young’s modulus etc.) and the available data collected in the database. In case of large amount of data (big databases) different methods can be applied. One of them is demonstrated in [22]. However, in case of small database, which is available for F/T phenomenon discussed in this paper, different approach should be applied. In order to obtained typical trends with F/T cycles, for optimal estimation of in terms of one or two mechanical properties, the standard statistical polynomial fit will be used in this (first) step. Two output parameters (M=2), Young’s modulus, E, and ductility, m, will be separately estimated as a function of two input parameters, F/T cycles (D=2) any type of mortar typeM. In this particular case equations 3 can be written as
and (4a)
and
and (4b)
In the presented research the estimates (i.e., functional relationship) of the variables and will be determined by using standard nonlinear regression (see Section 3).
However, to obtain as much information as possible from the observed phenomenon, a combination of Latin Hypercube Sampling (LHS) and fuzzy logic approach will be used next. While Latin Hypercube Sampling [23,24] is applied to obtain a reliable estimate of the statistical parameters for the probabilistic model, the fuzzy logic approach [25] is used to exploit the expert knowledge about the observed phenomenon (i.e. the influence of two mortars on the investigated mechanical properties). The latter approach is able to deal with the concept of partial truth, which enables incorporation of soft logic and/or engineering knowledge into the mathematical models. In summary, in terms of fragility curves for ductility and damage index will be estimated in this (second) step based on LHS data generated from assumed probability density functions and their statistical parameters (mean, standard deviation and coefficient of variation) of known input parameters of the phenomenon, including fuzzy logic. Note that in this case the formal description of equations 4 remains the same, only the finite set of model vectors (Nfc) increases significantly in this case due to the LHS (N Nfc).
Optimal estimation of will be carried out by applying the Conditional Average Estimation (CAE) method (Peruš et al., 2006). Note that the proposed approach (which includes application of LHS and fuzzy logic), takes into account the randomness and different uncertainties of the observed phenomenon of freezing and thawing. The fragility curve represents the probability curves of the F/T phenomenon. More specifically, the fragility curve of F/T phenomenon is defined as the conditional probability of exceedance for given values of the selected demand parameter (i.e., m) using the general definition [26,27]. The fragility curves can, therefore, be obtained by a series of reliability analyses (i.e., calculation of cumulative probability density functions for the mechanical property under consideration, taking into account all uncertainties) at varying demand thresholds. By ignoring the uncertainty in the demand [28,26], each fragility curve displays the probability that the actual mechanical property is less than or equal to a particular demand for this property.
Author Response File: Author Response.docx
Reviewer 5 Report
The conclusion must be improved. I have not found your main findings.
Fig. 1 : What does it mean the peaks in green curves around -15 C?
It is better to use Young's modulus instead of modulus of elasticity.
The units in Figs. 4, 5, 6, and 9 is missing.
Fig. 5: Use English style of numbers.
The resolution of Fig. 8 is poor.
Author Response
Response to Reviewer 5
Author's Notes to Reviewer:
The authors wish to thank the reviewer for his/her valuable comments and guidance. In the following, we provide point by point response to the received comments.
Point by point response:
Point 1: The conclusion must be improved. I have not found your main findings.
Response 1: The following text (in red) was included in the conclusions:
The presented research deals with F/T phenomenon of brick wallets that simulate structural elements (walls) of masonry buildings exposed to floods followed by cold weather. In an attempt to predict the progress of degradation and estimate the corresponding damage, the mathematical models which are able to predict important mechanical parameters and damage for brick wallets at given number of F/T cycles have been developed. Proposed statistical regressions enable estimation of deterioration of modulus of elasticity and displacement ductility. At the first stage the damage did not have significant effect on Young’s modulus. After 50 F/T cycles (M3a) and 80 F/T cycles (M1) the values begin to decrease. Although the wallets M3a built with improved mortar achieve significantly higher Young’s modulus, it’s decrease is more pronounced compared to wallets M1 built with ordinary one. Regarding the ductility properties somewhat better behavior can be observed by wallets built with improved mortar. In addition, proposed fragility curves for ductility and damage index (which is important novelty of the presented study) enable probabilistic risk assessments in case of deterioration of ductility and increase of damage on brick masonry due to F/T cycles. In terms of damage index wallets built with improved mortar showed higher durability compared to ordinary ones which is a good indicator for development and usage of other types of modified mortars. A simple use case has also been presented demonstrating usefulness of proposed graphs. It was shown how one is able to predict and obtain the values of Young’s modulus as well as the ductility at the selected age of a building exposed to F/T cycles.
Point 2: Fig. 1: What does it mean the peaks in green curves around -15 C?
Response 2: The following text was included in the article in the description of Figure 1:
From Fig. 1 it can be seen that the black line representing EN requirement and the green line which represents the average achieved temperature are not aligned. The differences can be attributed to deficiencies of insulation of the climate chamber but also to the cooling system, unable to provide complete illustration of programmed cooling-heating scheme. The average temperature was obtained from eight thermocouples at different positions in the climate chamber. The proximity of some thermocouples to the door opening or to the cooling-heating source thus affected the average measured temperature resulting in peaks in green curve.
Point 3: It is better to use Young's modulus instead of modulus of elasticity.
Response 3: Throughout the text, we replaced the previously used term modulus of elasticity with Young's modulus.
Point 4: The units in Figs. 4, 5, 6, and 9 is missing.
Response 4: Variables, presented in Figs. 4 (Fragility and Ductility), 5 (Damage index and Number of F/T cycles), 6 (Fragility and Damage index) and 9 (Fragility and Damage index) are all without units. This can be seen according to their definition and/or presented expressions. However, we noticed inconsistencies at variable’s abbreviations and corresponding designations of units in some figures – they are now fixed in Figs. 2-6 and 8-9.
Point 5: Fig. 5: Use English style of numbers.
Response 5: Fixed!
Point 6: The resolution of Fig. 8 is poor.
Response 6: Fixed!
Author Response File: Author Response.docx
Round 2
Reviewer 1 Report
The article can be published in the present form