Graph Convolutional Networks with Bidirectional Attention for Aspect-Based Sentiment Classification
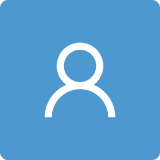
Round 1
Reviewer 1 Report
The paper overall looks like a solid piece of work, and I have no doubt the authors will be able to make it publish-worthy very quickly by fixing the flaws I list below: Major: 1. The paper does not emphasize what part of the newly proposed algorithm is new compared to the state of the art. A short paragraph should be added to make this more clear (personally, I would put it into a new subsection of Section 3, before going into the technical details, but other places are also possible. 2. The paper should present results on much bigger data sets as well. Currently, all data sets used in Section 4 are tiny, with thousands of instances. 3. When comparing algorithms, the times needed to train them should also be listed. 4. The conclusions section is very short and should be expanded. Minor: 1. Graph axes should be labelled 2. Section 4 starts directly with subsection 4.1 - this is bad style and should be avoided) 3. In tables presenting results, the best value in each column should be bold to ease readingAuthor Response
Please see the attachment.
Author Response File: Author Response.docx
Reviewer 2 Report
In this paper, the authors proposed a model for aspect-based sentiment classification using Graph Convolution Network. They evaluated the proposed model using three databases, and the results showed that it is an effective model for aspect-based sentiment classification.
The LOSS equation reference is wrong.
In line 412 the sentence "see from the table", please mention the table number.
Figures 3 and 4 need more explanation and comparison for your proposal model among the three databases and the reasons for obtaining better results with Rest14.
Figure 5 is blurred make it clear.
Author Response
Please see the attachment.
Author Response File: Author Response.docx
Reviewer 3 Report
The authors propose an aspect-based sentiment based on an attention mechanism and graph convolutional network.
The paper is quite interesting and the related presentation and technical qualities are good. Some reviews are suggested to improve the quality of paper.
First of all, the paper needs a linguistic revision. The related work misses several further relevant work on attention based deep architectures. Because you are introducing a sentiment analysis framework, its overview (integrating the entire process and the main system components) with the adopted technologies should be introduced. The section discussing effectiveness performances should be enriched by describing efficiency of the approach (running times and scalability).
Author Response
Please see the attachment.
Author Response File: Author Response.docx
Round 2
Reviewer 3 Report
The reviews have been adrressed.