Edge Computing Based on Federated Learning for Machine Monitoring
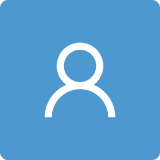
Round 1
Reviewer 1 Report
Figures quality must be improved
Figure 8 is it a figure or a table? what is observed from this?
The role of federated learning should be elaborated.
The need for federated learning in such an environment should be justified
More references and discussion should be included about machine monitoring
The research gap should be discussed about machine monitoring
The algorithm used for image recognition should be properly presented.
Accuracy of the image capturing and real-time processing should be discussed
Author Response
Response to Reviewer 1 Comments
The authors of this paper would like to thank the reviewer for spending so much valuable time reviewing our article. We deeply appreciate the constructive comments presented by the referee to improve the readability and increase the value of our article. We have thoroughly gone through the reviewer's comments and suggestions, and revised our article accordingly. In the following sections, we will state all comments raised by the reviewers and then present our modification to that comment.
Point 1: Figures quality must be improved
Response 1: Thank you for the valuable comment. The authors increased the resolution of each image in all figures to improve its quality.
Point 2: Figure 8 is it a figure or a table? what is observed from this?
Response 2: Thank you for the valuable comment. Figure 8 was changed to be Table 1. It shows the relationship between PLC panel features and corresponding abnormal events. For example, the intersection of row 1 and column 1 in Table 1 represents that the injection pressure has to increase in priority 2 when underfilling event are detected. These were stored in the local database of the factory. When the local system get a notification received from the cloud, the corresponding adjustment suggestion will send to the operator and manager to make a quick decision about the production process. Only features and parameters are communicated in the IoT outside of the factory such that each factory can keep its own confidential information for production. The paragraph was rewritten based on reviewer’s comment. Please find it in the revised file in Lines 382-401.
Point 3: The role of federated learning should be elaborated.
Response 3: The federated learning were used to generate features in fog and cloud computing based on the data sent from the edge such that the identification results could be obtained by combining those mid- and high-level features with the nonlinear classifier. Therefore, each factory could monitor the real-time condition of machines without operators. Since federated learning trained the decision model across multiple decentralized system of factories holding local data samples without exchanging them, it could keep the data privacy for real production of the factory. The paragraph was rewritten based on reviewer’s comment. Please find it in the revised file in Lines 204-211.
Point 4: I The need for federated learning in such an environment should be justified.
Response 4: Most factory managers are concerned about leaking confidential information about the production process. The traditional centralized machine learning techniques had to upload all the local datasets to the server. Since the cloud of the proposed IoT system is outside the factory, federated learning helped the system hold local data samples without exchanging them while training models. The paragraph was rewritten based on reviewer’s comment. Please find it in the revised file in Lines 204-211.
Point 5: More references and discussion should be included about machine monitoring.
Response 5: Thank you for the valuable comment. We added three references [27-29] in the revised version of the proposed paper. The corresponding paragraph was added. Please find it in the revised file in Lines 54-62 and 81-85.
Point 6: The research gap should be discussed about machine monitoring
Response 6: Most of the existing approaches about machine monitoring focused on building a total solution of IoT to fit the need of industry 4.0. It would not be acceptable under cost consideration. As the best of our knowledge, many control panel of the machines for small and medium sized factories were stand alone and did not have communication interface to transmit data directly. It might violate the warranty or cause damage to the production machine if we brute force install new communication interface on the control panel. Therefore, we proposed the vision based IoT technology based on federated learning to solve the immediate machine monitoring problem. The paragraph was added based on reviewer’s comment. Please find it in the revised file in Lines 109-117.
Point 7: The algorithm used for image recognition should be properly presented.
Response 7: Thank you for the valuable comment. The algorithm used for image recognition and its corresponding explanation was added. Please find it in the revised file in Fig. 7 and Lines 353-361.
Point 8: Accuracy of the image capturing and real-time processing should be discussed.
Response 8: Thank you for the valuable comment. We added some descriptions to discuss the accuracy of the image capturing and real-time processing. Please find it in the revised file in Lines 454-459 and 465-469.
Reviewer 2 Report
This paper presents an iot-based solution for machine monitoring in manufacturing. The paper claims to use federated learning and edge computing for such a system. The issue of the paper starts from the title which is misleading.
From the content, it seems that the problem of monitoring can be solved with a simple edge computing architecture. The need for federated learning is not well justified. What is the reason? Is there any privacy concern?
In addition, this is not clear if traditional FL is adopted or if any changes have been made.
Performance evaluation is very weak without much systematic results collection and explanations. How these results are generated and presented are not clear.
Last but not least, all figures in the paper are useless or irrelevant. They are barely readable or bring any more information to the content.
Author Response
Response to Reviewer 2 Comments
The authors of this paper would like to thank the reviewer for spending so much valuable time reviewing our article. We deeply appreciate the constructive comments presented by the referee to improve the readability and increase the value of our article. We have thoroughly gone through the reviewer's comments and suggestions, and revised our article accordingly. In the following sections, we will state all comments raised by the reviewers and then present our modification to that comment.
Point 1: This paper presents an iot-based solution for machine monitoring in manufacturing. The paper claims to use federated learning and edge computing for such a system. The issue of the paper starts from the title which is misleading.
Response 1: Thank you for the valuable comment. This paper actually presents an IoT based solution based on federated learning and edge computing for machine monitoring in manufacturing. Therefore, the title of the paper was changed to “IoT system for machine monitoring based on federated learning”. Please find it in the revised file.
Point 2: From the content, it seems that the problem of monitoring can be solved with a simple edge computing architecture. The need for federated learning is not well justified. What is the reason? Is there any privacy concern?
Response 2: The federated learning were used to generate features in fog and cloud computing based on the data sent from the edge such that the identification results could be obtained by combining those mid- and high-level features with the nonlinear classifier. Therefore, each factory could monitor the real-time condition of machines without operators and make a quick decision based on the knowledge database in the cloud server. Most factory managers are concerned about leaking confidential information about the production process. The traditional centralized machine learning techniques had to upload all the local datasets to the server. Since federated learning trained the decision model across multiple decentralized system of factories holding local data samples without exchanging them while training models, it could keep the data privacy for real production of the factory. Under reviewer’s constructive comments, the corresponding paragraph was rewritten based on reviewer’s comment. Please find it in the revised file in Lines 204-211.
Point 3: In addition, this is not clear if traditional FL is adopted or if any changes have been made.
Response 3: Thank you for the valuable comment. The traditional federated learning was adopted in the proposed system and the model was trained to fit the need of the study.
Point 4: Performance evaluation is very weak without much systematic results collection and explanations. How these results are generated and presented are not clear.
Response 4: We added the descriptions about the performance evaluation and experiment explanation to the revised version of the proposed paper. Please find it in the revised file in Lines 454-459 and 465-469.
Point 5: Last but not least, all figures in the paper are useless or irrelevant. They are barely readable or bring any more information to the content.
Response 5: Thank you for the valuable comment. We modified figures including its resolution and descriptions to the revised version of the proposed paper. Please find it in the revised file.
Round 2
Reviewer 1 Report
Comments given are addressed well
Reviewer 2 Report
My comments are addressed. I think better figures could be more useful for this paper.