Research on Chinese Medical Entity Recognition Based on Multi-Neural Network Fusion and Improved Tri-Training Algorithm
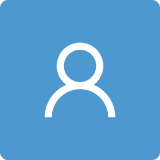
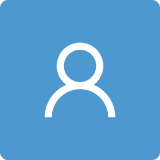
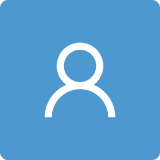
Round 1
Reviewer 1 Report
Although this is a manuscript produced from a local study, it can be considered as an approach that may have global implications. I think that more results can be given in the manuscript and more evaluation can be made with them.
Author Response
Please see the attachment.
Author Response File: Author Response.pdf
Reviewer 2 Report
The paper is very interesting in the NER domain, especially in medical text while dealing with low-resourced annotated corpus. The proposed approach is finely explained. The authors could improve more the paper quality.
Here are some recommendations.
Line 126: The reference [29] should be placed after [28].
Line 127: Please give any reference for Tri-training algorithm.
Line 137: LI et al. [32] should be Li et al.
Line 193: What does it mean IDCNN ? BERT does not be explained in the structure.
Could you please give some examples for BIO annotated data for training for ?
Section 4.1. Please make a table for training data about how many tokens, characters, words, etc.
Lines 170, and 172: Model3 should be Model 3. Model4 should be Model 4. And so on for lines 284, 286, 294, 302.
Lines 280, 321: Tab should be Table.
In the Table 2, should you mean the precision rate (P) instead of the accuracy rate ? This is not coherent with P, R, and F1 in your evaluation.
Please give some illustrations of prediction from all models.
Please make a sub-section for error analysis with some error illustrations from all your proposed models.
Would you open source your code, such as github or at your choice ?
Author Response
Please see the attachment.
Author Response File: Author Response.pdf
Reviewer 3 Report
Dear sir
It is an interesting paper, but please do the improvements based on what I mentioned above and I suggest to change the title.
Good Luck
Author Response
Please see the attachment.
Author Response File: Author Response.pdf