Pyramid Spatial-Temporal Graph Transformer for Skeleton-Based Action Recognition
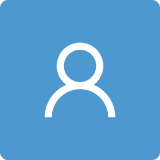
Round 1
Reviewer 1 Report
The authors propose a new technique for action recognition based on the Pyramid Transformer backbone (PGT) algorithm. The method proposes feature extraction in a multi-scale mode in a four-stage architecture for data represented as skeleton graphs.
The results and tests are robust and the authors have performed several tests on available databases to compare the results with previous works.
Overall the paper is clear, and the authors have presented an extensive review of the literature. However, section 3.1, which details the critical part of the algorithm is not clear. I believe that this section should be improved so that the explanation of the type of data being used is clearer. Unlike the image application, in this case, the data corresponds to a sequence of 3D points.
Author Response
The authors would like to thank the editor and reviewers for their insightful comments and suggestions which have significantly improved this manuscript.
For the problem in Section 3.1, we have added more explanation and revised the manuscript with colored fonts in this part as:" The skeleton data used for action recognition consists of several joints of humans, which is the 3D coordinates specifically. Unlike image data, skeleton graphs are more lightweight and more structurally relevant. For one action, it has three-dimensional parameters, like frame, joint number, and coordinate."
"Unlike the image-based vision tasks, we denote the 3D skeleton coordinate sequences as Fin \in RT x V x C, where T, V, C represent the frame, joint number and coordinate respectively. At the start of each stage i, a Spatial Graph Convolution is first applied to aggregate local information from neighbours according to the natural connection of joints."
Reviewer 2 Report
This is a very good paper, technically sounds good. There is some typo, please fix those, for e.g.,
line 205, As shown in fig ??, the 1*1 convolution
line 366, Northwestern-UCLA is a small dataset, which the pre-training is needed
Author Response
The authors would like to thank the editor and reviewers for their insightful comments and suggestions which have significantly improved this manuscript.
We have fixed the typo and grammatical errors to improve the writing. Thank you very much for your kind work.